the Creative Commons Attribution 4.0 License.
the Creative Commons Attribution 4.0 License.
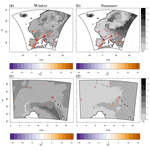
High-resolution LGM climate of Europe and the Alpine region using the regional climate model WRF
Emmanuele Russo
Jonathan Buzan
Sebastian Lienert
Guillaume Jouvet
Patricio Velasquez Alvarez
Basil Davis
Patrick Ludwig
Fortunat Joos
Christoph C. Raible
In this study we present a series of sensitivity experiments conducted for the Last Glacial Maximum (LGM, ∼21 ka) over Europe using the regional climate Weather Research and Forecasting model (WRF). Using a four-step two-way nesting approach, we are able to reach a convection-permitting horizontal resolution over the inner part of the study area, covering central Europe and the Alpine region. The main objective of the paper is to evaluate a model version including a series of new developments better suitable for the simulation of paleo-glacial time slices with respect to the ones employed in former studies. The evaluation of the model is conducted against newly available pollen-based reconstructions of the LGM European climate and takes into account the effect of two main sources of model uncertainty: a different height of continental glaciers at higher latitudes of the Northern Hemisphere and different land cover. Model results are in good agreement with evidence from the proxies, in particular for temperatures. Importantly, the consideration of different ensemble members for characterizing model uncertainty allows for increasing the agreement of the model against the proxy reconstructions that would be obtained when considering a single model realization. The spread of the produced ensemble is relatively small for temperature, besides areas surrounding glaciers in summer. On the other hand, differences between the different ensemble members are very pronounced for precipitation, in particular in winter over areas highly affected by moisture advection from the Atlantic. This highlights the importance of the considered sources of uncertainty for the study of European LGM climate and allows for determining where the results of a regional climate model (RCM) are more likely to be uncertain for the considered case study. Finally, the results are also used to assess the effect of convection-permitting resolutions, at both local and regional scales, under glacial conditions.
- Article
(7845 KB) - Full-text XML
-
Supplement
(4779 KB) - BibTeX
- EndNote
The Last Glacial Maximum (LGM) is known as the time at which the Northern Hemisphere ice-sheet volume reached its maximum during the last glacial period, at around 21 ka (Clark et al., 2009). The radiative forcing of the LGM was considerably different than the preindustrial (PI) period. Atmospheric greenhouse gas (GHG) concentrations were lower at LGM than at PI. At the same time, changes in the orbital configuration of the Earth around the Sun induced a different seasonal pattern of incoming insolation during the LGM, with lower values over the higher latitudes of the Northern Hemisphere during summer, with respect to the present day (Berger, 1978; Loulergue et al., 2008; Kageyama et al., 2017; Bereiter et al., 2015). The direct effect of different radiative forcing led to important changes at the global scale in the climate of the LGM compared to the present day, with overall cooler and drier conditions. Syntheses of proxy data and model outputs indicate that annual global temperatures were in the range of 4 to less than 8 °C lower than their PI values (Osman et al., 2021; Hargreaves et al., 2011). At the same time, feedback mechanisms, such as the ice-albedo effect, land cover changes, and ice-sheet expansion, played an important role in modulating the climate of the LGM, not only at the global scale but also at a regional and local level. For example, a large extension of northern hemispheric ice sheets had a strong impact on the large-scale circulation, with a different downward impact over different parts of Europe (Hofer et al., 2012b, a; Löfverström et al., 2014; Merz et al., 2015; Löfverström and Liakka, 2016; Löfverström et al., 2016; Ludwig et al., 2016; Kageyama et al., 2017).
The study of the climate of the LGM offers the opportunity to better understand processes and feedbacks of the climate system that have no analogue for the present day and the near-future (Kohfeld and Harrison, 2000; Kageyama et al., 2017; Raible et al., 2020). For this reason, the LGM represents a unique opportunity for evaluating the response of climate models to an extreme change in climate forcing, improving model reliability and contributing to model development (Kohfeld and Harrison, 2000). Consequently, the LGM has been one of the main target periods of paleoclimate modeling studies, with the first attempts at reproducing and understanding LGM climate dating back more than 40 years (Alyea, 1972; Williams et al., 1973; Kutzbach and Guetter, 1986; Rind, 1987; Gates, 1976; Manabe and Hahn, 1977).
The complexity of processes and feedbacks that directly or indirectly influenced the LGM climate makes the task of reproducing it via dynamical models particularly challenging. Over the years, this has led to models of increasing complexity being applied to the study of the LGM climate, from intermediate complexity models to fully coupled Earth system models (ESMs) (Braconnot et al., 2012; Harrison et al., 2014, 2015; Annan and Hargreaves, 2015; Masson-Delmotte et al., 2006; Izumi et al., 2013; Li and Morrill, 2013; Lambert et al., 2013; Schmidt et al., 2014; Otto-Bliesner et al., 2007; Muglia and Schmittner, 2021, 2015; Menviel et al., 2017; Sime et al., 2013; Kageyama et al., 2013; Hargreaves et al., 2011). However, these increases in model complexity have not generally led to improved model performance when compared against proxy data (Harrison et al., 2014; Annan and Hargreaves, 2015). In particular, the latest results of the Paleoclimate Modelling Intercomparison Project phase 4 (PMIP4) for the LGM show that regional climate biases that characterized previous generation of climate models, such as underestimation of winter extratropical cooling and precipitation changes, are still present in the more recent model developments (Kageyama et al., 2021).
In recent years, regional climate models (RCMs) have been employed for the study of the LGM climate, mainly motivated by their improved representation of local processes and having a spatial resolution better matched to those of proxy reconstructions with respect to general circulation models (GCMs). In particular, several studies have shown that RCMs for the LGM can improve the simulated climate in comparison with the driving GCM simulation (Strandberg et al., 2011; Ludwig et al., 2019). Recently, a series of LGM studies have revealed that areas with mountainous terrain, such as the Alps, profit from the use of an RCM with convection-permitting resolution (Velasquez et al., 2020, 2021, 2022). They have also highlighted the important role of land-surface characterization for the representation of the LGM climate over Europe.
It is important to acknowledge that the added value of RCMs for paleo- applications is still under debate. While some studies highlight that an RCM signal is too dictated by its driving GCM, playing a major role (Armstrong et al., 2019), others consider the relatively low computational demands of RCMs as an added value compared to their driving GCMs, since it allows for a more comprehensive characterization of uncertainties related to changes in soil and surface features (Russo et al., 2022). Both points are actually very important for the LGM, for which high uncertainties in RCM simulations may derive from the “garbage in–garbage out” effect related to the imposed GCM signal and from differences in the characterization of surface features such as land cover and ice height (Kjellström et al., 2010; Strandberg et al., 2011). These sources of uncertainty have to be properly taken into account when willing to assess the climate of the LGM using an RCM.
In this study, we present a series of LGM sensitivity experiments performed over Europe with the RCM WRF 3.8.1 (Skamarock et al., 2008; Powers et al., 2017) but including some important technical developments with respect to the same model version used in the former studies of Velasquez et al. (2020, 2021, 2022). These developments are crucial for ensuring the model's accurate application in paleoclimate studies. The performed experiments are built considering two different land-cover datasets, as well as changes in continental and Alpine glaciers extent for both WRF and its driving GCM. Model results are evaluated against the newly developed pollen-based reconstruction database for the European LGM climate of Davis et al. (2022). The main goals of the study are the evaluation of a new model version against proxy reconstructions, the characterization of model uncertainties resulting from changes in the simulations setup relative to land cover and ice height and the assessment of the role of convection-permitting simulations for paleoclimate studies. At the same time, with a main focus on the Alpine LGM climate, the study is aimed at producing high-resolution outputs to force a glacier model and reconstructing the extent of glaciers during glacial maxima (Jouvet et al., 2023).
Section 2 gives a general overview of the different methods and data used in this study. In Sect. 3, the results are presented: first a comparison against the proxy-based reconstructions is conducted for summer and winter values of temperatures and precipitation in Sect. 3.1; secondly, the role of different uncertainties is considered for the same seasons and variables in Sect. 3.2; thirdly, some considerations on the role of convection-permitting resolutions are drawn in Sect. 3.3. Finally, a summary of the obtained results is discussed in Sect. 4.
The results of this study are based on a set of simulations performed with the RCM WRF 3.8.1 (Skamarock et al., 2008; Powers et al., 2017). In the following, first the driving data used to conduct the performed simulations are introduced in Sect. 2.1. Successively, the general model setup is presented in Sect. 2.2, highlighting the main differences and improvements applied for the use of WRF in paleoclimate studies with respect to the same model version employed by Velasquez et al. (2020, 2021, 2022). Then, the complete set of performed LGM experiments is described in detail in Sect. 2.3. Finally, the new proxy reconstructions used for the assessment of the LGM model performance are introduced in Sect. 2.4.
2.1 Driving data
RCMs need climate information at their lateral boundaries. Here, outputs of a series of equilibrium climate simulations performed in a model chain of the Community Earth System Model (CESM 1.2 version, Hurrell et al., 2013) are used. CESM is a state-of-the-art Earth system model (ESM) consisting of different model components for the description of the atmosphere, land surface, ocean, and sea ice. In a first step, a fully coupled simulation at a horizontal resolution of 1.9° × 2.5° for the atmospheric and the land components and of nominal 1° × 1° for the ocean and the sea-ice components are executed (Buzan et al., 2023). Then, time-varying sea-ice mask and sea surface temperatures are derived from the simulation and are prescribed, in a successive step, to another experiment using only the atmosphere and land components of the CESM over an integration time of 24 years, at a horizontal resolution of 1.25° × 0.9°. Through these two steps, it is possible to reach a high spatial resolution, allowing for a realistic representation of the large-scale atmospheric circulation (Merz et al., 2015) while keeping the computational costs low.
For all of the CESM LGM simulations employed in this study, values of the orbital parameters (obliquity 22.949°, eccentricity 0.018994, and precession 294.425°), greenhouse gas concentrations (CO2 190 ppm, CH4 375 ppb, N2O 200 ppb), and land use changes for the corresponding period at 21 ka are used following the directives of the Paleoclimate Model Intercomparison Project 4 (PMIP4, Kageyama et al., 2017).
The reference simulation is performed using the ICE-6G ice-sheet reconstruction of Peltier et al. (2015), the modification of which follows the setup for the LGM PIMP4 protocol (Zhu et al., 2017). Moreover, three additional sensitivity experiments are performed (Buzan et al., 2023), where only the ice-sheet height of the main Northern Hemisphere ice sheets (Laurentide, Greenland, Fennoscandia) is varied, respectively, to 33 %, 67 %, and 125 % of their height as derived from the dataset of Peltier et al. (2015). Additional details on the CESM model set up are presented in Buzan et al. (2023).
2.2 WRF general model setup
The setup of the performed WRF simulations is partly based on the same model setup of earlier studies using the same model version 3.8.1 (Velasquez et al., 2020, 2021, 2022). This setup considers four two-way nested domains, with horizontal resolutions of 54, 18, 6, and 2 km, respectively (Fig. 1). The domains cover the entire European region, with a focus on the Alps. For the time integration, an adaptive time step is used with a minimum time step of 108, 36, 12, and 4 s, respectively, for the domains going from coarser to higher resolutions. The simulations are conducted considering a total of 40 vertical eta levels in the atmosphere, and four layers in the soil, with varying vertical resolution. The Kain–Fritsch cumulus convection scheme (Kain and Fritsch, 1990, 1993; Kain, 2004) is used for the coarser domains d01 and d02 of Fig. 1. In the inner domains d03 and d04, the convection parameterization is switched off since higher resolutions permit obtaining an explicit representation of convective processes. For all four domains, the land surface model NOAH-MP (Yang et al., 2011a) is used. For long-wave and short-wave radiation, the Dudhia and the RRTM schemes are respectively employed. The different LGM GHG values cannot be set in the model namelist but are passed directly to the corresponding RRTM shortwave radiation module prior to the model compilation, consistently with the values of the CESM simulations, as specified in Table 1. A general overview of the model setup employed for the different domains is presented in Table 2.
Sakaguchi and Zeng (2009)Having introduced the main features of the WRF simulations in common with Velasquez et al. (2021), we successively describe the main differences with respect to this former study. The initial setup of Velasquez et al. (2021) included a glacier scheme of NOAH-MP, which allowed ice-phase changes in the soil, that was found to be incorrect, producing unrealistic soil temperatures. The here presented simulations use a slab ice scheme of NOAH-MP that does not consider ice-phase changes in the soil, resulting in more realistic soil temperatures than the former option (not shown). Another major improvement with respect to the model version of Velasquez et al. (2021) considered in this study, particularly relevant for the application of WRF to paleoclimate studies, concerns the model representation of changes in the orbital parameters of the Earth on millennial timescales. In fact, while different values of the obliquity of the orbit can be passed directly into the code of the default model version 3.8.1, this is not possible for the different values of the parameters representing changes in the eccentricity of the Earth's orbit around the Sun and in the precession of the equinoxes. For this reason, a FORTRAN subroutine already employed in WRF version 4.1.2 in Ludwig and Hochman (2022) is implemented in the main radiation module of the model to include the full orbital forcing of the LGM experiments. The routine allows for scaling the value of the solar constant depending on the effective position of the Earth on its orbit, taking into account changes in the orbital parameters. It has been developed from a subroutine used in several other paleoclimate studies with the RCM COSMO-CLM (Fallah et al., 2016, 2018; Prömmel et al., 2013; Russo and Cubasch, 2016; Russo et al., 2022) and is based on an original subroutine used in the GCM ECHAM5 (Roeckner et al., 2003), considering basic Kepler laws only and using the long-term series expansions of Laskar et al. (1993). The effect of the implemented subroutine on the seasonal pattern of insolation is here assessed for the coarsest study domain d01. Figure S1 of the Supplement shows the differences in incoming radiation on top of the atmosphere calculated for each day of a year between the LGM and the PI periods, for the default (left) and the new (right) version of the model. The results with the new treatment of orbital parameters (Fig. S1, right) are now in line with the expected seasonal pattern of insolation at the LGM over the study domain (Kageyama et al., 2017), substantially different than the one obtained from the original model version (Fig. S1, left).
2.3 WRF sensitivity experiments
The different CESM simulations with a spatial resolution of 1.25° × 0.9° introduced in Sect. 2.1 are used as initial and boundary conditions to run WRF. A total of five simulations with convection-permitting resolutions are performed with the WRF model for the LGM, each one covering approximately 11 years. For each of these simulations, given the extremely high computational demands of very high-resolution, each period is divided into three sub-periods of different length, between 52 and 40 months and considering the first 4 months as spinup time. In the end, the results of these sub-periods are joined together to retrieve a mean climatology for each of the performed experiments, based on the mean calculated over a total of 10 years. The approach is similar to the one of previous studies such as the one of Velasquez et al. (2020, 2021, 2022). It might not allow for exhaustively assessing the interannual variability in a model over a given period of study, due to the short time frames considered for each experiment. Nevertheless, since the interest of this study is mainly climatological mean values, this represents a good compromise between high demand in computational resources and the length of a signal to be used for building up a climatology. In support of this choice, the results of a 31-year long simulation of Velasquez et al. (2021) are also considered.
To perform experiments with WRF under LGM conditions, further modifications to the surface boundary conditions are necessary. Changes to land cover, soil composition, ice extent, ice height, and the land–sea mask are applied here to the original present-day WRF datasets. A reference simulation, here referred to as DEF (Table 3), is performed using the outputs from the reference CESM simulation of Buzan et al. (2023) as boundary conditions. For the DEF experiment, the LGM topography is prescribed directly from the dataset of Peltier et al. (2015). In particular, the maximum ice height in the period from 24 and 18 ka as derived from Peltier et al. (2015) is considered in this case. For the Alpine region, in the DEF experiment the ice-cap elevation is derived using the Parallel Ice Sheet Model (PISM, Khroulev and the PISM Authors, 2020) forced by the WRF LGM simulation of Velasquez et al. (2021), and tuned such that the glacial maximum area fits the reconstructed one by Ehlers et al. (2011). The land–sea mask of the DEF simulation is derived from Peltier et al. (2015), also considering the largest extension of ice in between 24 and 18 ka.
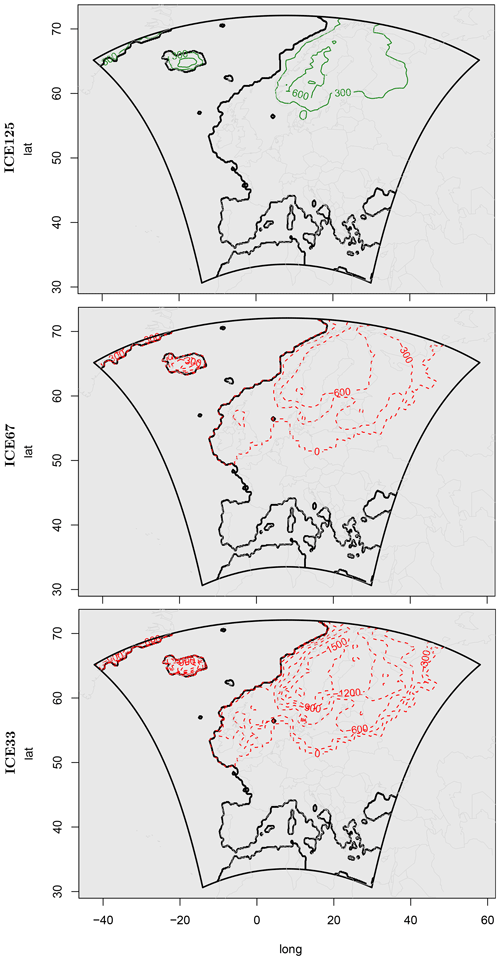
Figure 2Orographic differences in the simulations (from top to bottom) ICE125, ICE67, and ICE33, calculated for the coarser domain of study d01 with respect to the reference simulation DEF. The different contours are drawn at intervals of 300 m. Dotted red contours represent negative values, while continuous green contours represent positive values.
Three additional simulations are conducted using in each case the different boundary data derived from the CESM simulations with perturbed LGM ice height over the higher latitudes of the Northern Hemisphere (see Sect. 2.1). Consistently with the driving data, for these three simulations the height of the northern latitudes ice sheets of the DEF simulation over the considered domain of study is increased by 25 %, and reduced by 67 % and 33 %. These simulations will be referred to, in the following text, respectively, as 125ICE, 67ICE, and 33ICE (Table 3). The orographic differences in each of these simulations with modified ice sheet, calculated for domain d01 with respect to the DEF experiment, are presented in Fig. 2. The most pronounced differences in prescribed topography are evident over the inner part of Scandinavia, with values lower than −1800 m obtained from the comparison between experiments 33ICE and DEF. In all the different cases, the ice-sheet extent (represented as white shadings in Fig. 3) is kept constant.
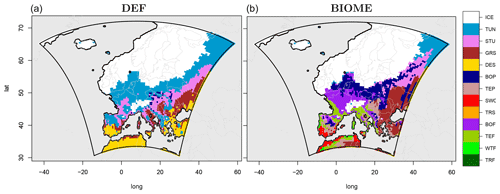
Figure 3LGM land cover and biome classes for the coarser domain of study d01, passed as input to the DEF (a) and BIOME (b) simulations. Besides land ice cover indicated as ICE, all the legend acronyms of the different biome species derived from LPX-Bern are specified in Table 4.
Table 4List of biomes from LPX-Bern (left) and the corresponding ones from MODIS they have been converted to (right).
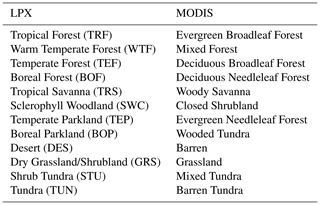
For the LGM land cover, the LGM simulation by Velasquez et al. (2021) is used offline to drive the dynamic vegetation Land surface Processes and eXchanges model of the University of Bern (LPX-Bern v1.4) (Lienert and Joos, 2018; Spahni et al., 2013; Stocker et al., 2013). LPX-Bern features dynamic carbon, water, and nitrogen cycles and vegetation is internally represented by 10 plant functional types (PFTs; eight tree and two herbaceous) competing for resources and adhering to bioclimatic limits. Input variables for LPX-Bern are the fraction of land in a grid cell and monthly values of total precipitation, near-surface temperature, and net downward shortwave radiation at surface. Furthermore, for nitrogen deposition preindustrial values of the forcing product (Tian et al., 2018) closest to the model grid cell are used. LPX-Bern horizontal resolution is adapted to match the horizontal resolution of the respective WRF domains. For using the LGM maps of vegetation derived from LPX-Bern into WRF, the 12 biomes of LPX-Bern need to be translated into one of the 16 classes of WRF (based on the data from the US Geological Survey (USGS)). The selected BIOME correspondence between the two different datasets is presented in Table 3.
All the four simulations introduced above (namely DEF, ICE33, ICE67, and ICE125) use the same vegetation map derived with LPX-Bern from the simulation of Velasquez et al. (2021). Additionally, in order to acknowledge the possible effect of uncertainties in the prescribed land cover, a simulation is performed by driving offline LPX-Bern with the climatological outputs of the DEF simulation. This simulation will be referred to as the BIOME simulation. The DEF and the BIOME experiments are run using the same CESM boundary conditions at 1° × 1° resolution. A map of the two different land cover datasets used for the conducted experiments is presented in Fig. 3. For a large part of the domain there are noticeable differences in vegetation, both on a continental and local scale. The two datasets seem to correspond only over a remote part of northeastern Europe. For this reason, the designed experiments are likely to provide important information on the role of uncertainty related to the use of a different land cover in RCM simulations of European climate at the LGM. Present-day soil categories are used for all the LGM simulations, with some modifications: all the soil layers corresponding to a point covered by glaciers are set to ice. Consistently, for a given grid box, the same soil category of the upper soil layer is used for all soil levels at different depths.
In order to conduct a more quantitative evaluation of WRF against the pollen-based reconstructions taking into account different model uncertainties, the five experiments introduced above (i.e., DEF, ICE33, ICE67, ICE125, and BIOME) are considered together as an ensemble of simulations. Here, as model uncertainty, we consider the range of outputs that is obtained using the same model but applying changes to the model chain setup inherent to land cover and ice height.
Finally, in addition to the already described simulations, an experiment with the same setup of the DEF simulation but using only two nested domains, D01 and D02, down to a spatial resolution of 18 km and with the convection parameterization switched on (i.e., convection is not explicitly solved), is performed. This experiment is indicated as DEF_parconv in Table 3 and is conducted with the goal of better assessing the role of convection-permitting simulations for the representation of the European LGM climate.
2.4 Proxy reconstructions dataset
The model simulations are evaluated against a new pollen-based reconstruction of LGM seasonal and annual temperature and precipitation by Davis et al. (2022). This reconstruction is based on pollen data from 63 pollen records that were selected for the quality of their dating control over the LGM period (21 ka cal ±2 kyr). Records were excluded if they fell within category 7 (“poor”) of the 1–7 COHMAP scale of chronological quality based on the proximity of dates to the LGM time slice. Only absolute dating methods (e.g., radiometric dating such as radiocarbon) were considered, and relative dates based on pollen correlation were excluded. The record selection process, therefore, excluded many of the pollen records used in previous studies, reflecting the generally poor quality of the dating control in LGM pollen records, including 10 of the 18 records used in the PMIP benchmarking dataset (Bartlein et al., 2011). The reconstruction itself uses a standard modern analogue technique (MAT) that finds the best six analogues amongst a dataset of over 8000 modern pollen samples from the Eurasian Modern Pollen Database (Davis et al., 2020). The MAT method has been used for previous LGM pollen–climate reconstructions (Peyron et al., 1998) but it has not been known how much the method may be influenced during the LGM by problems such as having no modern analogue vegetation (and climate), as well as changing plant physiological responses to climate associated with lower atmospheric CO2. For this reason, the method was evaluated against a previous pollen–climate reconstruction based on inverse modeling (Wu et al., 2007), which is designed to account for many of these problems, as well as a chironomid-based reconstruction (Samartin et al., 2016). The result of this evaluation showed little difference between the MAT reconstruction and these other methods, indicating that the MAT method is reliable for this time period and location. This can be attributed in part to advances in the size and spatial coverage of the modern pollen training set, which is an order of magnitude larger than those used in previous MAT reconstructions, permitting good vegetation analogues (chord distance <0.3, Huntley, 1990) to be found for all fossil pollen samples. Importantly, the uncertainty estimates of the considered pollen-based reconstructions are considered in their comparison against the results of the presented model simulations.
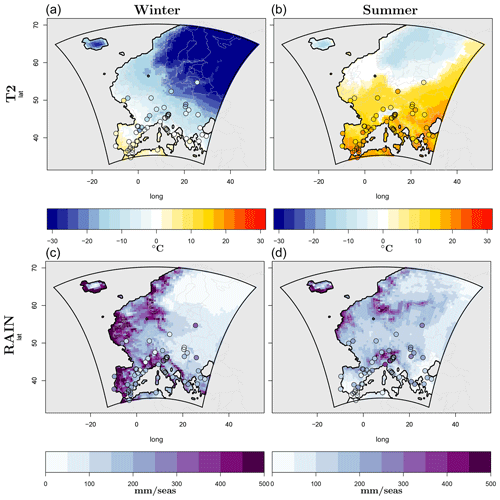
Figure 4LGM winter (a, c) and summer (b, d) climatological values of mean near-surface temperature (a, b) and precipitation (c, d) as derived from the reference WRF LGM simulation, for the domain d01 of Fig. 1, at coarser resolution. The circles represent the values as derived from pollen-based reconstructions.
3.1 Comparison of the reference simulation against pollen-based reconstructions
In Fig. 4 we present the mean climatologies of winter and summer temperatures and precipitation of the DEF LGM simulation over the coarsest domain of the study, together with the corresponding values derived from the pollen-based reconstructions. Winter temperatures below 0 °C characterize almost the entire domain for the DEF experiment, except for a few points over southern Spain and northwestern Africa. Particularly cold simulated temperatures are evident in winter over the northeastern part of Europe, with values generally below −25 °C. Temperatures remain lower than 0 °C in the model in summer over north and northeastern Europe, over areas largely covered by glaciers. Simulated temperatures rarely exceed a mean value of 20 °C in summer, only over specific areas of southern Europe and northern Africa. The values of the pollen-based reconstructions, displayed in Fig. 4 as filled circles, show a similar behavior, even though their coverage is mainly limited to central and southern Europe. In winter, the pollen data are also characterized by a northeast-to-southwest gradient in temperatures, with values consistent with the ones of the WRF DEF experiment. In summer, reconstructed temperatures are similar to the ones simulated by WRF for the entire domain, presenting in particular a similar north-to-south gradient. The simulated precipitation pattern in winter is characterized by high values in the western areas of the domain (Fig. 4, lower row), for which moisture advection from the Atlantic Ocean plays a major role (Beghin et al., 2016; Ulbrich et al., 1999), up to seasonal values of 500 mm/season in some cases. In summer, drier conditions are evident over almost the entire domain, except for few areas with complex topography such as part of the Alps and western Scandinavia, where precipitation exceeds 300 mm/season. The pollen-based reconstructions show a slightly different picture compared to the DEF simulation for precipitation in winter. While the western coastal areas are wetter than eastern Europe according to the pollen, pronounced dry conditions are evident over the central part of the domain, particularly at the northern edges of the Alps. Additionally, the range of values of winter precipitation derived from the pollen-based reconstructions is generally narrower than in the case of the DEF simulation, with maximum values rarely exceeding 250 mm/season. For summer precipitation, on the other hand, there is a better agreement between the simulated data and proxy reconstructions than in winter, except for specific areas, such as again around the Alps. In summer, the simulated values of precipitation are in the same range as the climate reconstructions.
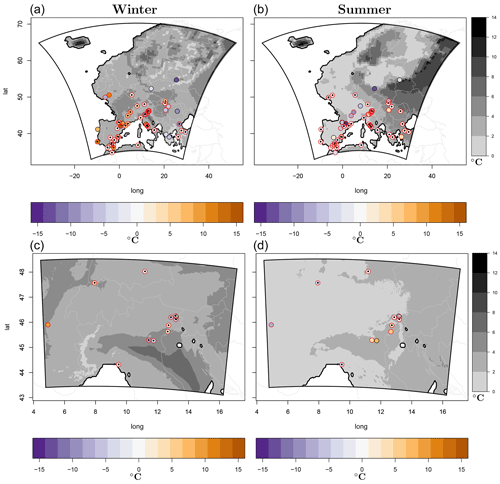
Figure 5Maps for the bias of winter (a, c) and summer (b, d) temperatures calculated between the WRF reference simulation and the pollen-based reconstructions. Upper row (a, b) shows the results for the coarser-resolution domain d01, while the bottom row (c, d) presents the same analysis for the higher-resolution domain d04. The colored circles show the biases calculated between the two datasets. In the case of the bias being smaller than 1 standard deviation of the reconstructions, a black dot is added at the center of the circle. Considering then the five-member ensemble, in the case of the bias of at least one of these members against the reconstructions being smaller than 1 standard deviation of the latter, then the circle is highlighted in red. Finally, the gray scale represents the maximum differences for each point of the domains, between the different members of the ensemble.
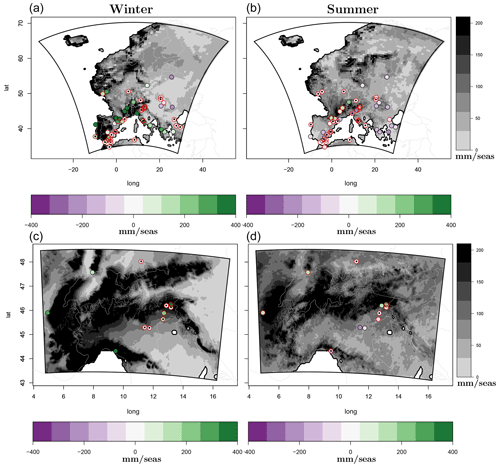
Figure 6Maps for the bias of winter (a, c) and summer (b, d) seasonal precipitation calculated between the WRF reference simulation and the pollen-based reconstructions. Upper row (a, b) shows the results for the coarser-resolution domain d01, while the bottom row (c, d) presents the same analysis for the higher-resolution domain d04. The colored circles show the biases calculated between the two datasets. In the case of the bias being smaller than 1 standard deviation of the reconstructions, a black dot is added at the center of the circle. Considering then the five-member ensemble, in the case of the bias of at least one of these members against the reconstructions being smaller than 1 standard deviation of the latter, then the circle is highlighted in red. Finally, the gray scale represents the maximum differences for each point of the domains, between the different members of the ensemble.
3.2 Consideration of different model uncertainties
A more quantitative assessment of the differences in seasonal values of temperature and precipitation between the DEF WRF simulation and the pollen-based reconstructions is provided in Figs. 5 and 6, respectively. In these figures, the temperature and precipitation derived from the additional WRF simulations BIOME, 33ICE, 67ICE, and 125ICE of Table 3 (forming, together with the DEF simulation, a five-member ensemble) are also considered. The comparison between the pollen-based reconstructions and the model outputs is additionally conducted on both the coarse-resolution domain, and the inner domain d04 of Fig. 1 in this case. A circle with the values of the differences between the reference simulation DEF and the pollen-based reconstructions is drawn for the proxy locations. If the bias of the reference simulation DEF lies within 1 standard deviation of the uncertainty values of the pollen-based reconstructions, then a black dot is added at the center of a given circle. Successively, if at least one of the five-member ensemble lies within 1 standard deviation of the pollen values, then the circle is highlighted in red. Finally, the maximum absolute differences in the considered variables calculated between the different ensemble members are plotted for each point of the domain on a gray scale in order to provide an assessment of the ensemble spread and model uncertainty.
Figure 5 confirms that, for both winter and summer, there is a very good agreement in temperatures between the performed simulations and the values derived from the proxies. The consideration of the different simulations with the applied changes in the model configuration allow for obtaining results that are very close to the evidence of the proxies for almost the entire points of the domain, in both seasons and for both considered variables. For winter temperature, the bias of the reference (DEF) exceeds in very few cases an absolute value of 5 °C. Interestingly, despite the large changes in the configuration of the different ensemble members, their results in terms of winter temperature are relatively close for almost the entire domain of study (see also Fig. S2). Differences between the different ensemble members larger than 4 °C are evident for winter temperature mainly over the northeastern part of the domain and Iceland, with values of the different ensemble members spreading here over a range of more than 10 °C. For summer, again, the mean model temperatures are very close to the values of the pollen-based reconstructions over the entire domain (Fig. 5, right column). The DEF simulation, in particular, shows a small bias against the proxies over the entire domain, with values rarely exceeding 4 °C and well within the range of the pollen uncertainty. The main exceptions are some points over the area of present-day France, for which a closer agreement is reached only when considering other members of the ensemble. In summer, more notable and spatially heterogeneous differences in temperatures are evident between the different ensemble members than in winter. In particular, large differences, of up to 14 °C, are found between the different experiments for areas at the border of glaciers over Scandinavia and Iceland, mainly due to a reduction in the height of glaciers over these regions (Fig. S3).
Analyses for the inner Alpine domain (Fig. 5, bottom row) confirm an overall good match between temperatures derived from the performed simulations and the pollen-based reconstructions, for both summer and winter. In this case, the main differences between the different ensemble members are evident in winter over the Po valley, exceeding in some cases 6 °C. Just two points of the pollen reconstructions, in summer, have temperature values outside the range of the ensemble members for the inner domain of study. This gives high confidence on the use of the presented results for the study of the LGM climate of the area.
For precipitation (Fig. 6), the agreement between model simulations and proxy reconstructions is not as good as in the case of temperature. In winter, the model seems to be generally too wet, in particular over the northern Iberian Peninsula, present-day France, the Alps, and continental areas of present-day Greece and Turkey, with seasonal values of precipitation exceeding the ones derived from pollen-based reconstructions by more than 300 mm/season. For these areas, none of the performed simulations gets significantly closer to the range of values of the proxies, also when taking into account pollen uncertainties. It is important to note here that most of the locations for which the model presents the largest positive biases in winter precipitation are at the boarder of mountainous areas covered with glaciers, such as the Alps and part of the Pyrenees. This positive bias could partly be related to uncertainties in the extent or height of the prescribed glaciers, not properly resolved at the scales characteristic of the considered pollen data. This is confirmed by the fact that the bias for the two points northwest of the Alps is reduced when considering the model results over the Alpine region at an horizontal resolution of 2 km (Fig. 6, bottom row). In winter, the areas for which the DEF simulation presents higher values of precipitation, i.e., western Europe (Fig. 4, lower row), are the same as those for which higher differences in the ensemble members are evident, exceeding in most of these areas 150 mm/season. These pronounced differences between the different ensemble members are mainly dictated by the experiments with changes in the height of glaciers (Fig. S4). A similar overlapping of the largest differences in the ensemble members over regions presenting the highest values of precipitation in the DEF simulation is also evident in summer. In this case though, there is a better agreement between the results of the performed simulations and the pollen-based reconstructions, although the model seems to be generally drier, in particular over the Balkans, where the differences against the pollen-based reconstructions are in some cases below −200 mm/season. Notable in this case is that changes in land cover seem to be very important for the representation of summer precipitation over the Mediterranean region, in particular over the Balkans (Fig. S5), where the BIOME simulation produces significant changes with respect to the DEF experiment.
For the inner domain of study there is also a relatively good agreement between the proposed simulations and the pollen-based reconstructions in terms of precipitation, for both seasons, but this is again worse than in the case of temperature (Fig. 5, lower row), at least when considering the number of points for which the simulations lie within the pollen uncertainty. Three important points need to be mentioned in the case of precipitation for the inner domain of study. Firstly, the differences between the different ensemble members are considerable over all of the Alpine range, in both winter and summer, with values extensively exceeding 200 mm/season. This confirms again the relevance of the considered sources of uncertainty in the model for the investigation of the LGM climate of the region. Secondly, even though the simulations are out of the range of the considered pollen-based reconstructions over some points in one season, they get closer in the other. This indicates that some of the results, even if not perfectly matching the values of the proxies, are not completely off. Thirdly, a point also valid for the rest of the domain is that the pollen reconstructions are trained with present-day observations, and studies show that even observations nowadays tend to underestimate precipitation in particular areas characterized by complex terrain (Fu, 1996; Frei and Schär, 1998; Frei et al., 2003).
Finally, to confirm that the differences between the different ensemble members are robust and not simply an effect of the selection of a relatively short simulation period of 10 years, from which the climatological values are obtained, we have also calculated the maximum differences in the climatological values of 20 different periods of 10 years derived from the 31-year long simulation of Velasquez et al. (2021), for summer temperatures. The results, as illustrated in Fig. S6, indicate that the spread of this set of experiments is negligible compared to the one obtained when comparing the different realizations of our five-member ensemble.
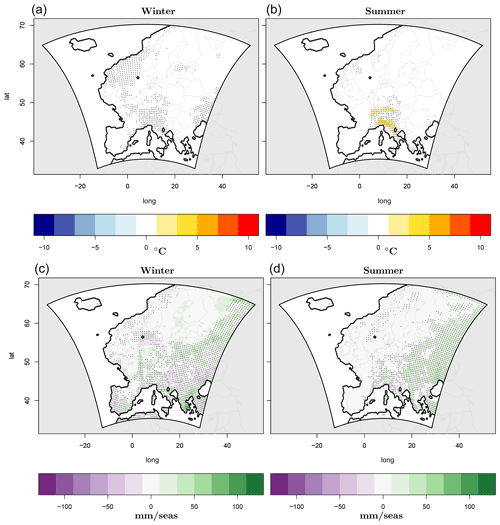
Figure 7Bias in winter (a, c) and summer (b, d) values of temperatures (a, b) and precipitation (c, d) calculated for the coarser-resolution domain d01, between the reference simulation and the one with only two nested domains, down to 18 km. The dots in each of the panels represent the points for which the values differ significantly, at a significance level of 0.05, according to the results of a KS significance test.
3.3 Considerations on the role of convection-resolving resolutions
In Fig. 7 the differences in the mean seasonal values of temperature and precipitation between the reference DEF simulation and the DEF_parconv experiment are calculated for each grid box of the outer domain d01. The main goal of such an analysis is to assess whether the explicit representation of convection over the inner part of the domain has a relevant impact on the simulated variables at both the local and continental scale, with respect to a simulation exclusively using a parameterized representation of convection over all the domain. In Fig. 7, for each grid-box where the daily mean anomalies of the considered variable and season can be considered significantly different according to the results of a Kolmogorov–Smirnov (KS) significance test at a significance level of 0.05, a dot is plotted.
Results show that for the Alpine region, the effects of higher resolution and the explicit representation of convection are significant, for both variables and seasons. For precipitation, in particular, the effects of the convection-permitting simulation are seen not only over the inner domains but also over the entire domain of study, presenting significant differences between the two simulations. These differences are in some cases of the same order of magnitude as the differences between the different sensitivity tests conducted by perturbing the glaciers ice height and extension (see Supplement, Fig. S2) and considering considerably different boundaries discussed in Sect. 3.2. Therefore, the consideration of convection-permitting resolutions for the simulation of European LGM climate has a significant impact on the results, comparable to the effect of other relevant sources of uncertainty, not only at a local scale but over the entire European domain.
In this study, we conduct an evaluation of a version of the RCM WRF 3.8.1, including a series of important technical developments necessary for paleoclimate applications, against newly available pollen-based reconstructions for the LGM climate of Europe and the Alpine region. Through the performance of a set of sensitivity experiments taking into account the role of different large-scale and surface model error sources, such as changes in the imposed northern hemispheric ice height and land cover, we aim to assess the general performance of the model. At the same time, we quantify the possible effect of changes in the model setup on the obtained results, highlighting where outcomes of RCMs can be considered more robust and where factors such as error in the representation of surface features could play a major role in the reconstruction of the European LGM climate.
Results show that the model ensemble produced by changing surface drivers and using different boundaries is in very good agreement with the considered pollen-based reconstructions for summer and winter values of temperature. For precipitation, the agreement is also relatively good, but in this case none of the produced ensemble members gets as close to the proxies over the entire domain of study as in the case of temperature. In particular, the model results are too wet in winter over western Europe and too dry in summer over the eastern part of Europe, with respect to the pollen-based reconstructions, even when considering the uncertainty associated with the considered pollen-based reconstructions. The model shows a positive northeast-to-southwest gradient of temperatures in winter over Europe at the LGM, in accordance with the picture drawn from the pollen-based reconstructions. Most of the European domain presents mean winter values below 0 °C as derived from the model simulations. A positive north-to-south gradient characterizes simulated summer temperatures, again similarly to evidence from the proxies (Russo, 2023). In summer, mean simulated temperatures rarely exceed 20 °C. The LGM WRF precipitation shows particularly high values, exceeding in some cases 400 mm/season over Atlantic regions of western Europe and the Alps. In summer, the pattern of precipitation in the model is quite homogeneously distributed, with values rarely exceeding 150 mm/season, except for specific areas characterized by complex topography, such as Scandinavia and the Alps. The consideration of different northern hemispheric ice height and land cover types in the model leads to largely differing precipitation values over specific areas, with differences between the different experiments exceeding 200 mm/season in some cases. This allows for increasing the match between the model and the proxies, at the same time highlighting the importance of the considered sources of uncertainty for the simulation of European climate at the LGM over different regions. Errors associated with changes in the ice height of the higher latitudes of the Northern Hemisphere produce very pronounced changes in both winter and summer precipitation over western Europe and the Alpine region. Changes in land cover also play an important role in summer precipitation over the Mediterranean basin. The considered changes have a relatively limited impact on winter temperatures over almost the entire domain, playing instead a larger role in summer temperatures, in particular over areas at the boarders of glaciers, with differences between the different ensemble members reaching 14 °C in some cases. Finally, the use of four two-way nested domains, enabling reaching a spatial resolution in the inner domain of approximately 2×2 km and explicitly representing convection, leads to important changes in model results with respect to an experiment with parameterized convection over the inner part of the domain. Significant differences between the two experiments are evident not only over the inner Alpine region but also over the outer domain of study. These changes are particularly pronounced for precipitation rather than for temperature, being in the range of the changes relative to the use of different ice height and land cover in this case.
The paper sets the basis for an improved representation of the LGM climate of Europe. It introduces some important technical developments useful for the application of WRF to the study of the LGM with respect to previously employed model versions, allowing for obtaining seasonal values of temperature and precipitation in very good agreement with newly available pollen-based reconstructions. Additionally, it permits assessment of the role of different sources of model uncertainty, as well as where and for which variable largest differences in model results might be expected as a result of a different model setup. Finally, the study enables assessment of the effect of convection-permitting resolutions for the study of the LGM climate at both the local and continental scale.
WRF is a community model that can be downloaded from the following web page: https://www2.mmm.ucar.edu/wrf/users/ (WRF, 2022). The climate simulations employed in this study (global: CESM; regional: WRF), as well as the land cover simulations, occupy several terabytes of memory and thus are not freely available. Nevertheless, they can be accessed upon request to the contributing authors. The scripts used for the presented analyses are available at https://doi.org/10.5281/zenodo.7998789 (Russo, 2023).
The supplement related to this article is available online at: https://doi.org/10.5194/cp-20-449-2024-supplement.
ER, JB, and CCR contributed to the design of the experiments. ER carried out the regional simulations and wrote the first draft. JB carried out the global simulations. SL performed the LPX simulations and PV provided first input data. BD provided the proxy reconstructions. All authors contributed to the interpretation of the results, the writing, and scientific discussion.
The contact author has declared that none of the authors has any competing interests.
Publisher's note: Copernicus Publications remains neutral with regard to jurisdictional claims made in the text, published maps, institutional affiliations, or any other geographical representation in this paper. While Copernicus Publications makes every effort to include appropriate place names, the final responsibility lies with the authors.
The simulations were performed on the supercomputing architecture of the Swiss National Supercomputing Centre (CSCS).
This research has been supported by the Schweizerischer Nationalfonds zur Förderung der Wissenschaftlichen Forschung (grant no. 200021-162444).
This paper was edited by Laurie Menviel and reviewed by two anonymous referees.
Alyea, F.: Numerical simulation of an ice age paleoclimate Atmosopheric Science Paper No. 193, Colorado State University, 1972. a
Annan, J. and Hargreaves, J.: A perspective on model-data surface temperature comparison at the Last Glacial Maximum, Quaternary Sci. Rev., 107, 1–10, 2015. a, b
Armstrong, E., Hopcroft, P., and Valdes, P.: Reassessing the value of regional climate modeling using paleoclimate simulations, Geophys. Res. Lett., 46, 12464–12475, 2019. a
Bartlein, P., Harrison, S., Brewer, S., Connor, S., Davis, B., Gajewski, K., Guiot, J., Harrison-Prentice, T., Henderson, A., Peyron, O., Prentice, I., Scholze, M., Seppä, H., Shuman, B., Sugita, S., Thompson, R., Viau, A., Williams, J., and Wu, H.: Pollen-based continental climate reconstructions at 6 and 21 ka: a global synthesis, Clim. Dynam., 37, 775–802, 2011. a
Beghin, P., Charbit, S., Kageyama, M., Combourieu-Nebout, N., Hatté, C., Dumas, C., and Peterschmitt, J.: What drives LGM precipitation over the western Mediterranean? A study focused on the Iberian Peninsula and northern Morocco, Clim. Dynam., 46, 2611–2631, 2016. a
Bereiter, B., Eggleston, S., Schmitt, J., Nehrbass-Ahles, C., Stocker, T., Fischer, H., Kipfstuhl, S., and Chappellaz, J.: Revision of the EPICA Dome C CO2 record from 800 to 600 kyr before present, Geophys. Res. Lett., 42, 542–549, 2015. a
Berger, A. L.: Long-term variations of daily insolation and quaternary climatic changes, J. Atmos. Sci., 35, 2362–2367, https://doi.org/10.1175/1520-0469(1978)035<2362:LTVODI>2.0.CO;2, 1978. a
Braconnot, P., Harrison, S., Kageyama, M., Bartlein, P., Masson-Delmotte, V., Abe-Ouchi, A., Otto-Bliesner, B., and Zhao, Y.: Evaluation of climate models using palaeoclimatic data, Nat. Clim. Change, 2, 417–424, 2012. a
Buzan, J. R., Russo, E., Kim, W. M., and Raible, C. C.: Winter sensitivity of glacial states to orbits and ice sheet heights in CESM1.2, EGUsphere [preprint], https://doi.org/10.5194/egusphere-2023-324, 2023. a, b, c, d
Clark, P. U., Dyke, A. S., Shakun, J. D., Carlson, A. E., Clark, J., Wohlfarth, B., Mitrovica, J. X., Hostetler, S. W., and McCabe, A. M.: The last glacial maximum, Science, 325, 710–714, 2009. a
Davis, B. A. S., Chevalier, M., Sommer, P., Carter, V. A., Finsinger, W., Mauri, A., Phelps, L. N., Zanon, M., Abegglen, R., Åkesson, C. M., Alba-Sánchez, F., Anderson, R. S., Antipina, T. G., Atanassova, J. R., Beer, R., Belyanina, N. I., Blyakharchuk, T. A., Borisova, O. K., Bozilova, E., Bukreeva, G., Bunting, M. J., Clò, E., Colombaroli, D., Combourieu-Nebout, N., Desprat, S., Di Rita, F., Djamali, M., Edwards, K. J., Fall, P. L., Feurdean, A., Fletcher, W., Florenzano, A., Furlanetto, G., Gaceur, E., Galimov, A. T., Gałka, M., García-Moreiras, I., Giesecke, T., Grindean, R., Guido, M. A., Gvozdeva, I. G., Herzschuh, U., Hjelle, K. L., Ivanov, S., Jahns, S., Jankovska, V., Jiménez-Moreno, G., Karpińska-Kołaczek, M., Kitaba, I., Kołaczek, P., Lapteva, E. G., Latałowa, M., Lebreton, V., Leroy, S., Leydet, M., Lopatina, D. A., López-Sáez, J. A., Lotter, A. F., Magri, D., Marinova, E., Matthias, I., Mavridou, A., Mercuri, A. M., Mesa-Fernández, J. M., Mikishin, Y. A., Milecka, K., Montanari, C., Morales-Molino, C., Mrotzek, A., Muñoz Sobrino, C., Naidina, O. D., Nakagawa, T., Nielsen, A. B., Novenko, E. Y., Panajiotidis, S., Panova, N. K., Papadopoulou, M., Pardoe, H. S., Pędziszewska, A., Petrenko, T. I., Ramos-Román, M. J., Ravazzi, C., Rösch, M., Ryabogina, N., Sabariego Ruiz, S., Salonen, J. S., Sapelko, T. V., Schofield, J. E., Seppä, H., Shumilovskikh, L., Stivrins, N., Stojakowits, P., Svobodova Svitavska, H., Święta-Musznicka, J., Tantau, I., Tinner, W., Tobolski, K., Tonkov, S., Tsakiridou, M., Valsecchi, V., Zanina, O. G., and Zimny, M.: The Eurasian Modern Pollen Database (EMPD), version 2, Earth Syst. Sci. Data, 12, 2423–2445, https://doi.org/10.5194/essd-12-2423-2020, 2020. a
Davis, B. A. S., Fasel, M., Kaplan, J. O., Russo, E., and Burke, A.: The climate and vegetation of Europe, North Africa and the Middle East during the Last Glacial Maximum (21,000 years BP) based on pollen data, Clim. Past Discuss. [preprint], https://doi.org/10.5194/cp-2022-59, in review, 2022. a, b
Ehlers, J., Gibbard, P. L., and Hughes, P. D.: Developments in Quaternary Sciences: Quaternary Glaciations-Extent and Chronology: A Closer Look, vol. 15, 2–1108, Elsevier, 2011. a
Fallah, B., Sodoudi, S., and Cubasch, U.: Westerly jet stream and past millennium climate change in Arid Central Asia simulated by COSMO-CLM model, Theor. Appl. Climatol., 124, 1079–1088, 2016. a
Fallah, B., Russo, E., Acevedo, W., Mauri, A., Becker, N., and Cubasch, U.: Towards high-resolution climate reconstruction using an off-line data assimilation and COSMO-CLM 5.00 model, Clim. Past, 14, 1345–1360, https://doi.org/10.5194/cp-14-1345-2018, 2018. a
Frei, C. and Schär, C.: A precipitation climatology of the Alps from high-resolution rain-gauge observations, Int. J. Climatol., 18, 873–900, 1998. a
Frei, C., Christensen, J., Déqué, M., Jacob, D., Jones, R. G., and Vidale, P.: Daily precipitation statistics in regional climate models: Evaluation and intercomparison for the European Alps, J. Geophys. Res.-Atmos., 108, 4124, https://doi.org/10.1029/2002JD002287, 2003. a
Fu, Q.: An accurate parameterization of the solar radiative properties of cirrus clouds for climate models, J. Climate, 9, 2058–2082, 1996. a
Gates, W.: Modeling the ice-age climate, Science, 191, 1138–1144, 1976. a
Hargreaves, J. C., Paul, A., Ohgaito, R., Abe-Ouchi, A., and Annan, J. D.: Are paleoclimate model ensembles consistent with the MARGO data synthesis?, Clim. Past, 7, 917–933, https://doi.org/10.5194/cp-7-917-2011, 2011. a, b
Harrison, S., Bartlein, P., Brewer, S., Prentice, I., Boyd, M., Hessler, I., Holmgren, K., Izumi, K., and Willis, K.: Climate model benchmarking with glacial and mid-Holocene climates, Clim. Dynam., 43, 671–688, 2014. a, b
Harrison, S., Bartlein, P., Izumi, K., Li, G., Annan, J., Hargreaves, J., Braconnot, P., and Kageyama, M.: Evaluation of CMIP5 palaeo-simulations to improve climate projections, Nat. Clim. Change, 5, 735–743, 2015. a
Hofer, D., Raible, C. C., Dehnert, A., and Kuhlemann, J.: The impact of different glacial boundary conditions on atmospheric dynamics and precipitation in the North Atlantic region, Clim. Past, 8, 935–949, https://doi.org/10.5194/cp-8-935-2012, 2012a. a
Hofer, D., Raible, C. C., Merz, N., Dehnert, A., and Kuhlemann, J.: Simulated winter circulation types in the North Atlantic and European region for preindustrial and glacial conditions, Geophys. Res. Lett., 39, 15805, https://doi.org/10.1029/2012GL052296, 2012b. a
Huntley, B.: European vegetation history: Palaeovegetation maps from pollen data – 13 000 yr BP to present, J. Quaternary Sci., 5, 103–122, 1990. a
Hurrell, J., Holland, M., Gent, P., Ghan, S., Kay, J., Kushner, P., Lamarque, J., Large, W., Lawrence, D., Lindsay, K., Lipscomb, W., Long, M., Mahowald, N., Marsh, D. R., Neale, R. B., Rasch, P., Vavrus, S., Vertenstein, M., Bader, D., Collins, W. D., Hack, J. J., Kiehl, J., and Marshall, S.: The Community Earth System Model: A framework for collaborative research, B. Am. Meteorol. Soc., 94, 1339–1360, 2013. a
Izumi, K., Bartlein, P., and Harrison, S.: Consistent large-scale temperature responses in warm and cold climates, Geophys. Res. Lett., 40, 1817–1823, 2013. a
Jouvet, G., Cohen, D., Russo, E., Buzan, J., Raible, C., Hischer, U., Haeberli, W., Kamleitner, S., Ivy-Ochs, S., Imhof, M., Becker, J., and Landgraf, A.: Coupled climate-glacier modelling of the last glaciation in the Alps, J. Glaciol., online first, 1–15, https://doi.org/10.1017/jog.2023.74, 2023. a
Kageyama, M., Braconnot, P., Bopp, L., Mariotti, V., Roy, T., Woillez, M., Caubel, A., Foujols, M., Guilyardi, E., Khodri, M., Guilyardi, E., Khodri, M., Lloyd, J., Lombard, F., and Marti, O.: Mid-Holocene and last glacial maximum climate simulations with the IPSL model: Part II: Model-data comparisons, Clim. Dynam., 40, 2469–2495, 2013. a
Kageyama, M., Albani, S., Braconnot, P., Harrison, S. P., Hopcroft, P. O., Ivanovic, R. F., Lambert, F., Marti, O., Peltier, W. R., Peterschmitt, J.-Y., Roche, D. M., Tarasov, L., Zhang, X., Brady, E. C., Haywood, A. M., LeGrande, A. N., Lunt, D. J., Mahowald, N. M., Mikolajewicz, U., Nisancioglu, K. H., Otto-Bliesner, B. L., Renssen, H., Tomas, R. A., Zhang, Q., Abe-Ouchi, A., Bartlein, P. J., Cao, J., Li, Q., Lohmann, G., Ohgaito, R., Shi, X., Volodin, E., Yoshida, K., Zhang, X., and Zheng, W.: The PMIP4 contribution to CMIP6 – Part 4: Scientific objectives and experimental design of the PMIP4-CMIP6 Last Glacial Maximum experiments and PMIP4 sensitivity experiments, Geosci. Model Dev., 10, 4035–4055, https://doi.org/10.5194/gmd-10-4035-2017, 2017. a, b, c, d, e
Kageyama, M., Harrison, S. P., Kapsch, M.-L., Lofverstrom, M., Lora, J. M., Mikolajewicz, U., Sherriff-Tadano, S., Vadsaria, T., Abe-Ouchi, A., Bouttes, N., Chandan, D., Gregoire, L. J., Ivanovic, R. F., Izumi, K., LeGrande, A. N., Lhardy, F., Lohmann, G., Morozova, P. A., Ohgaito, R., Paul, A., Peltier, W. R., Poulsen, C. J., Quiquet, A., Roche, D. M., Shi, X., Tierney, J. E., Valdes, P. J., Volodin, E., and Zhu, J.: The PMIP4 Last Glacial Maximum experiments: preliminary results and comparison with the PMIP3 simulations, Clim. Past, 17, 1065–1089, https://doi.org/10.5194/cp-17-1065-2021, 2021. a
Kain, J.: The Kain-Fritsch convective parameterization: An update, J. Appl. Meteorol., 43, 170–181, 2004. a
Kain, J. and Fritsch, J. M.: A one-dimensional entraining/detraining plume model and its application in convective parameterization, J. Atmos. Sci., 47, 2784–2802, 1990. a
Kain, J. and Fritsch, J. M.: Convective parameterization for mesoscale models: The Kain-Fritsch scheme, in: The representation of cumulus convection in numerical models, Springer, 165–170, 1993. a
Khroulev, C. and the PISM Authors: PISM, a Parallel Ice Sheet Model v1.2: User's Manual, http://www.pism-docs.org (last access: 1 June 2022), 2020. a
Kjellström, E., Brandefelt, J., Näslund, J.-O., Smith, B., Strandberg, G., Voelker, A. H. L., and Wohlfarth, B.: Simulated climate conditions in Europe during the Marine Isotope Stage 3 stadial, Boreas, 39, 436–456, https://doi.org/10.1111/j.1502-3885.2010.00143.x, 2010. a
Kohfeld, K. and Harrison, S.: How well can we simulate past climates? Evaluating the models using global palaeoenvironmental datasets, Quaternary Sci. Rev., 19, 321–346, 2000. a, b
Kutzbach, J. and Guetter, P.: The influence of changing orbital parameters and surface boundary conditions on climate simulations for the past 18 000 years, J. Atmos. Sci., 43, 1726–1759, 1986. a
Lambert, F., Kug, J., Park, R., Mahowald, N., Winckler, G., Abe-Ouchi, A., O’ishi, R., Takemura, T., and Lee, J.: The role of mineral-dust aerosols in polar temperature amplification, Nat. Clim. Change, 3, 487–491, 2013. a
Laskar, J., Joutel, F., and Boudin, F.: Orbital, precessional, and insolation quantities for the Earth from −20 Myr to +10 Myr, Astron. Astrophys., 270, 522–533, 1993. a
Li, Y. and Morrill, C.: Lake levels in Asia at the Last Glacial Maximum as indicators of hydrologic sensitivity to greenhouse gas concentrations, Quaternary Sci. Rev., 60, 1–12, 2013. a
Lienert, S. and Joos, F.: A Bayesian ensemble data assimilation to constrain model parameters and land-use carbon emissions, Biogeosciences, 15, 2909–2930, https://doi.org/10.5194/bg-15-2909-2018, 2018. a
Löfverström, M. and Liakka, J.: On the limited ice intrusion in Alaska at the LGM, Geophys. Res. Lett., 43, 11–030, 2016. a
Löfverström, M., Caballero, R., Nilsson, J., and Kleman, J.: Evolution of the large-scale atmospheric circulation in response to changing ice sheets over the last glacial cycle, Clim. Past, 10, 1453–1471, https://doi.org/10.5194/cp-10-1453-2014, 2014. a
Löfverström, M., Caballero, R., Nilsson, J., and Messori, G.: Stationary wave reflection as a mechanism for zonalizing the Atlantic winter jet at the LGM, J. Atmos. Sci., 73, 3329–3342, 2016. a
Loulergue, L., Schilt, A., Spahni, R., Masson-Delmotte, V., Blunier, T., Lemieux, B., Barnola, J., Raynaud, D., Stocker, T., and Chappellaz, J.: Orbital and millennial-scale features of atmospheric CH4 over the past 800,000 years, Nature, 453, 383–386, 2008. a
Ludwig, P. and Hochman, A.: Last glacial maximum hydro-climate and cyclone characteristics in the Levant: A regional modelling perspective, Environ. Res. Lett., 17, 014053, https://doi.org/10.1088/1748-9326/ac46ea, 2022. a
Ludwig, P., Schaffernicht, E. J., Shao, Y., and Pinto, J. G.: Regional atmospheric circulation over Europe during the Last Glacial Maximum and its links to precipitation, J. Geophys. Res.-Atmos., 121, 2130–2145, https://doi.org/10.1002/2015JD024444, 2016. a
Ludwig, P., Gómez-Navarro, J. J., Pinto, J. G., Raible, C. C., Wagner, S., and Zorita, E.: Perspectives of regional paleoclimate modeling, Ann. NY Acad. Sci., 1436, 54–69, https://doi.org/10.1111/nyas.13865, 2019. a
Manabe, S. and Hahn, D.: Simulation of the tropical climate of an ice age, J. Geophys. Res., 82, 3889–3911, 1977. a
Masson-Delmotte, V., Kageyama, M., Braconnot, P., Charbit, S., Krinner, G., Ritz, C., Guilyardi, E., Jouzel, J., Abe-Ouchi, A., Crucifix, M., Gladstone, R., Hewitt, C. D., Kitoh, A., LeGrande, A., Marti, O., Merkel, U., Motoi, T., Ohgaito, R., Otto-Bliesner, B., Peltier, W., Ross, I., Valdes, P., Vettoretti, G., Weber, S., Wolk, F., and Yu, Y.: Past and future polar amplification of climate change: Climate model intercomparisons and ice-core constraints, Clim. Dynam., 26, 513–529, 2006. a
Menviel, L., Yu, J., Joos, F., Mouchet, A., Meissner, K., and England, M.: Poorly ventilated deep ocean at the Last Glacial Maximum inferred from carbon isotopes: A data-model comparison study, Paleoceanography, 32, 2–17, 2017. a
Merz, N., Raible, C., and Woollings, T.: North Atlantic eddy-driven jet in interglacial and glacial winter climates, J. Climate, 28, 3977–3997, 2015. a, b
Muglia, J. and Schmittner, A.: Glacial Atlantic overturning increased by wind stress in climate models, Geophys. Res. Lett., 42, 9862–9868, 2015. a
Muglia, J. and Schmittner, A.: Carbon isotope constraints on glacial Atlantic meridional overturning: Strength vs depth, Quaternary Sci. Rev., 257, 106 844, 2021. a
Osman, M., Tierney, J., Zhu, J., Tardif, R., Hakim, G., King, J., and Poulsen, C.: Globally resolved surface temperatures since the Last Glacial Maximum, Nature, 599, 239–244, 2021. a
Otto-Bliesner, B., Hewitt, C., Marchitto, T., Brady, E., Abe-Ouchi, A., Crucifix, M., Murakami, S., and Weber, S.: Last Glacial Maximum ocean thermohaline circulation: PMIP2 model intercomparisons and data constraints, Geophys. Res. Lett., 34, L12706, https://doi.org/10.1029/2007GL029475, 2007. a
Peltier, W., Argus, D., and Drummond, R.: Space geodesy constrains ice age terminal deglaciation: The global ICE-6G_C (VM5a) model, J. Geophys. Res.-Sol. Ea., 120, 450–487, 2015. a, b, c, d, e
Peyron, O., Guiot, J., Cheddadi, R., Tarasov, P., Reille, M., de Beaulieu, J., Bottema, S., and Andrieu, V.: Climatic reconstruction in Europe for 18,000 yr BP from pollen data, Quaternary Res., 49, 183–196, 1998. a
Powers, J., Klemp, J., Skamarock, W., Davis, C., Dudhia, J., Gill, D., Coen, J., Gochis, D., Ahmadov, R., Peckham, S., Grell, G., Michalakes, J., Trahan, S., Benjamin, S., Alexander, C., Dimego, G., Wang, W., Schwartz, C., Romine, G., Liu, Z., Snyder, C., Chen, F., Barlage, M., Yu, W., and Duda, M.: The weather research and forecasting model: Overview, system efforts, and future directions, B. Am. Meteorol. Soc., 98, 1717–1737, 2017. a, b
Prömmel, K., Cubasch, U., and Kaspar, F.: A regional climate model study of the impact of tectonic and orbital forcing on African precipitation and vegetation, Palaeogeogr. Palaeocl., 369, 154–162, 2013. a
Raible, C. C., Pinto, J. G., Ludwig, P., and Messmer, M.: A review of past changes in extratropical cyclones in the Northern Hemisphere and what can be learned for the future, Wiley Interdisciplinary Reviews: Climate Change, 12, e680, https://doi.org/10.1002/wcc.680, 2020. a
Rind, D.: Components of the ice age circulation, J. Geophys. Res.-Atmos., 92, 4241–4281, 1987. a
Roeckner, E., Bäuml, G., Bonaventura, L., Brokopf, R., Esch, M., Giorgetta, M., Hagemann, S., Kirchner, I., Kornblueh, L., Manzini, E., Rhodin, A. Schlese, U., Schulzweida, U., and Tompkins, A.: The atmospheric general circulation model ECHAM 5. PART I: Model description, Tech. rep., Max-Planck-Institut für Meteorologie, 2003. a
Russo, E.: R scripts for paper of Russo et al. 2023: “High-resolution LGM climate of Europe and the Alpine region using the regional climate model WRF”, Zenodo [code], https://doi.org/10.5281/zenodo.7998789, 2023. a, b
Russo, E. and Cubasch, U.: Mid-to-late Holocene temperature evolution and atmospheric dynamics over Europe in regional model simulations, Clim. Past, 12, 1645–1662, https://doi.org/10.5194/cp-12-1645-2016, 2016. a
Russo, E., Fallah, B., Ludwig, P., Karremann, M., and Raible, C. C.: The long-standing dilemma of European summer temperatures at the mid-Holocene and other considerations on learning from the past for the future using a regional climate model, Clim. Past, 18, 895–909, https://doi.org/10.5194/cp-18-895-2022, 2022. a, b
Sakaguchi, K. and Zeng, X.: Effects of soil wetness, plant litter, and under‐canopy atmospheric stability on ground evaporation in the Community Land Model (CLM3.5), J. Geophys. Res.-Atmos., 114, D01107, https://doi.org/10.1029/2008JD010834, 2009. a
Samartin, S., Heiri, O., Kaltenrieder, P., Kühl, N., and Tinner, W.: Reconstruction of full glacial environments and summer temperatures from Lago della Costa, a refugial site in Northern Italy, Quaternary Sci. Rev., 143, 107–119, 2016. a
Schmidt, G. A., Annan, J. D., Bartlein, P. J., Cook, B. I., Guilyardi, E., Hargreaves, J. C., Harrison, S. P., Kageyama, M., LeGrande, A. N., Konecky, B., Lovejoy, S., Mann, M. E., Masson-Delmotte, V., Risi, C., Thompson, D., Timmermann, A., Tremblay, L.-B., and Yiou, P.: Using palaeo-climate comparisons to constrain future projections in CMIP5, Clim. Past, 10, 221–250, https://doi.org/10.5194/cp-10-221-2014, 2014. a
Sime, L., Kohfeld, K., Le Quéré, C., Wolff, E., de Boer, A., Graham, R., and Bopp, L.: Southern Hemisphere westerly wind changes during the Last Glacial Maximum: Model-data comparison, Quaternary Sci. Rev., 64, 104–120, 2013. a
Skamarock, W. C., Klemp, J. B., Dudhia, J., Gill, O., Barker, D., Duda, G., Huang, X.-Y., Wang, W., and Powers, G.: A description of the advanced research WRF Version 3, National Center for Atmospheric Research, Mesoscale and Microscale Meteorology Division, https://doi.org/10.5065/D68S4MVH, 2008. a, b
Spahni, R., Joos, F., Stocker, B. D., Steinacher, M., and Yu, Z. C.: Transient simulations of the carbon and nitrogen dynamics in northern peatlands: from the Last Glacial Maximum to the 21st century, Clim. Past, 9, 1287–1308, https://doi.org/10.5194/cp-9-1287-2013, 2013. a
Stocker, B., Roth, R., Joos, F., Spahni, R., Steinacher, M., Zaehle, S., Bouwman, L., and Prentice, I.: Multiple greenhouse-gas feedbacks from the land biosphere under future climate change scenarios, Nat. Clim. Change, 3, 666–672, 2013. a
Strandberg, G., Brandefelt, J., Kjellstro M., E., and Smith, B.: High-resolution regional simulation of Last Glacial Maximum climate in Europe, Tellus A, 63, 107–125, https://doi.org/10.1111/j.1600-0870.2010.00485.x, 2011. a, b
Tian, H., Yang, J., Lu, C., Xu, R., Canadell, J., Jackson, R., Arneth, A., Chang, J., Chen, G., Ciais, P., Gerber, S., Ito, A., Huang, Y., Joos, F., Lienert, S., Messina, P., Olin, S., Pan, S., Peng, C., Saikawa, E.and Thompson, R., Vuichard, L., Winiwarter, W., Zaehle, S., Zhang, B., Zhang, K., and Zhu, Q.: The global N2O model intercomparison project, B. Am. Meteorol. Soc., 99, 1231–1251, 2018. a
Ulbrich, U., Christoph, M., Pinto, J., and Corte-Real, J.: Dependence of winter precipitation over Portugal on NAO and baroclinic wave activity, Int. J. Climatol., 19, 379–390, 1999. a
Velasquez, P., Messmer, M., and Raible, C. C.: A new bias-correction method for precipitation over complex terrain suitable for different climate states: a case study using WRF (version 3.8.1), Geosci. Model Dev., 13, 5007–5027, https://doi.org/10.5194/gmd-13-5007-2020, 2020. a, b, c, d, e
Velasquez, P., Kaplan, J. O., Messmer, M., Ludwig, P., and Raible, C. C.: The role of land cover in the climate of glacial Europe, Clim. Past, 17, 1161–1180, https://doi.org/10.5194/cp-17-1161-2021, 2021. a, b, c, d, e, f, g, h, i, j, k, l, m
Velasquez, P., Messmer, M., and Raible, C. C.: The role of ice-sheet topography in the Alpine hydro-climate at glacial times, Clim. Past, 18, 1579–1600, https://doi.org/10.5194/cp-18-1579-2022, 2022. a, b, c, d, e
Williams, J., Berry, R., and Washington, W.: Simulation of the climate at the last glacial maximum using the NCAR global circulation model, Institute of Alpine and Arctic Research , University of Colorado, Boulder, 1973. a
WRF: WRF Model Users' Page, WRF [data set], https://www2.mmm.ucar.edu/wrf/users/, last access: 4 April 2022. a
Wu, H., Guiot, J., Brewer, S., and Guo, Z.: Climatic changes in Eurasia and Africa at the last glacial maximum and mid-Holocene: reconstruction from pollen data using inverse vegetation modelling, Clim. Dynam., 29, 211–229, 2007. a
Yang, Z., Niu, G., Mitchell, K., Chen, F., Ek, M., Barlage, M., Longuevergne, L., Manning, K., Niyogi, D., Tewari, M., and Xia, Y.: The community Noah land surface model with multiparameterization options (Noah-MP): 2. Evaluation over global river basins, J. Geophys. Res.-Atmos., 116, D12110, https://doi.org/10.1029/2010JD015140, 2011. a
Zhu, J., Liu, Z., Brady, E., Otto-Bliesner, B., Zhang, J., Noone, D., Tomas, R., Nusbaumer, J., Wong, T., Jahn, A., and Tabor, C.: Reduced ENSO variability at the LGM revealed by an isotope-enabled Earth system model, Geophys. Res. Lett., 44, 6984–6992, https://doi.org/10.1002/2017GL073406, 2017. a