the Creative Commons Attribution 4.0 License.
the Creative Commons Attribution 4.0 License.
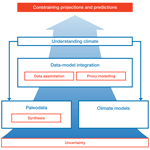
Preface: Advances in paleoclimate data synthesis and analysis of associated uncertainty: towards data–model integration to understand the climate
Oliver Bothe
Michal Kucera
- Article
(283 KB) - Full-text XML
- BibTeX
- EndNote
Paleoclimate data1 can provide insights into climate dynamics across a range of timescales and for climate states that are inaccessible from the instrumental record. Thus, the study of the paleoclimate record can improve our understanding of how the climate system operates. Whilst interest in past climate is valid in its own right, research on past climate change has gained urgency because of the ongoing anthropogenic intervention in the climate system, which has led to changes that are unparalleled over at least a few thousands of years and possibly irreversible for centuries and already negatively affects societies and ecosystems across the world (IPCC, 2021). This is because the paleoclimate record can serve as a baseline of natural variability, provides information on how the climate system responds to changes in the forcing and may serve as an analogue of future climate (e.g. Tierney et al., 2020).
Climate models are in a way a summary of our current knowledge of the climate system. They are essential tools to assess mechanisms, test hypotheses and project future climate trajectories. To apply climate models outside the current climate state, their skill under conditions different from today has to be demonstrated. In this respect, comparing paleoclimate simulations with paleoclimate data is the only means of evaluating and constraining climate models under boundary conditions different from those during the instrumental period. Paleoclimate data–model integration can therefore deliver insights into the climate system that data or models on their own cannot provide (Fig. 1).
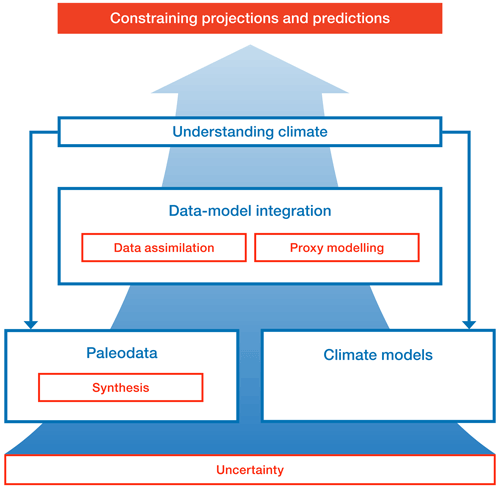
Figure 1Schematic workflow of paleodata–model integration to improve the understanding of the climate system in order to constrain projections and predictions of future climate. The four aspects of the data–model integration process discussed here are highlighted in red font. The process relies on thorough quantification and understanding of the uncertainty associated with both. For this reason uncertainty is at the base of the workflow (however, model-related uncertainty is not explicitly covered here). Further steps in this process that are covered in this special issue include data synthesis and data assimilation and proxy modelling as means to bring data and models together.
Comparing paleoclimate data with simulations appears simple at first sight. However, from a paleoclimate data perspective this process is not straightforward because paleoclimate observations are associated with considerable uncertainty since they are by nature indirect and contain chronological error. In addition, paleoclimate information is irregularly distributed in time and space, and meta-analysis of existing paleoclimate data is non-trivial because of fragmented and non-standardised archiving. Thus, in order to allow meaningful data–model integration, paleoclimate data need to be standardised and synthesised, and the associated uncertainty needs to be constrained.
This short article serves as an introduction to the inter-journal special issue “Paleoclimate data synthesis and analysis of associated uncertainty” in the journals Climate of the Past (https://cp.copernicus.org/articles/special_issue11_936.html, last access: 13 December 2021) and Earth System Science Data (https://essd.copernicus.org/articles/special_issue11_936.html, last access: 13 December 2021). The purpose of this special issue is to provide a platform to present new paleoclimate synthesis products, to review the current state of proxy uncertainty analysis and to present new developments in data–model integration approaches such as proxy forward modelling and data assimilation. The special issue was conceived as part of the paleoclimate synthesis working group of the German climate modelling project PALMOD (https://www.palmod.de, last access: 13 December 2021) but with submission open to the entire paleoclimate science community.
This special issue contains 23 papers covering various steps in the data–model integration process. In this introduction we place the individual contributions in the context of a paleodata–model integration concept (Fig. 1). In this concept, paleoclimate data and climate model simulations provide information, which is combined in the integration efforts such that an improved understanding of the climate as well as the nature of the paleoclimate data and the models emerges. The contributions in this issue are grouped into four important aspects of the integration process, which we briefly describe below: paleoclimate data synthesis, paleoclimate data uncertainty, proxy modelling and data assimilation.
The starting point of the data–model integration process is formed by individual paleoclimate time series. Over the past decades thousands of paleoclimate time series based on different proxy sensors and derived from different geological archives have been generated. Fortunately, a large part of the data in these time series is publicly available. However, the data are archived in a non-standardised way, and important metadata are often hidden in the original publications. Meaningful use of paleoclimate time series thus requires laborious retrieval and some form of harmonisation of data and metadata. This process can be facilitated through the use of dedicated software, such as PaleoDataView (Langner and Mulitza, 2019), which is specifically designed for standardisation and visualisation of time series from marine sediment archives.
This special issue contains detailed descriptions of several synthesis approaches and products. Two are archive specific: the VARDA synthesis (Ramisch et al., 2020) contains detailed chronological information about annually resolved time series from laminated (varved) lakes and the PalMod 130k marine paleoclimate data synthesis contains multi-proxy time series from marine sediments across the world oceans (Jonkers et al., 2020). Other syntheses are focussed on specific variables, such as water isotope time series over the Common Era (Konecky et al., 2020), pollen records across Siberia from the past 40 000 years (Cao et al., 2020) and greenhouse gas concentrations for the past 156 000 years (Köhler et al., 2017). These contributions show how the value of the individual time series is increased through harmonisation of ontologies, metadata and chronological information.
Further contributions make use of such synthesis efforts to provide temporally or spatially continuous coverage that can be compared more easily with simulations or used as boundary conditions for climate model experiments. Thus, the pollen database (Cao et al., 2020) has been used to derive maps of land cover (Cao et al., 2019), and Paul et al. (2021) transformed previously synthesised data (MARGO Project Members, 2009) to provide a global map of sea surface temperature including uncertainty for the last glacial maximum. The gridding method used to construct the global sea surface temperature map also holds promise to extrapolate paleoclimate observations that have generally coarse spatial distribution.
Two contributions show how individual time series can be used to derive continuous regional paleoclimate time series. Building on previous work (Lisiecki and Stern, 2016), Peterson and Lisiecki (2018) provide regional stacks of benthic foraminifera stable carbon isotopes, which reflect the carbon isotope composition of dissolved inorganic carbon in oceanic bottom waters. In turn, this stable carbon isotope composition provides important constraints on the changes in the carbon cycle and ocean circulation. Fuhrmann et al. (2020) present continental aridity time series spanning 60 000 years based on multiple proxies from key regions.
All data underlying the syntheses as well as the derived data products are publicly available (see individual papers for access). Each collection has the potential to reveal spatial and/or temporal patterns that remain invisible in individual time series. By including rich metadata and chronological information, they facilitate the consideration of uncertainty in paleoclimate data–model integration.
For meaningful interpretation of paleoclimate data, it is crucial to be able to separate signal from noise. This requires first a quantification of the uncertainty associated with the indirect recording of climate by proxies.
Two contributions to this special issue aim to quantify the signal-to-noise ratio of proxy time series directly by assessing the correlation among nearby paleoclimate time series that should have experienced the same climate (Reschke et al., 2019; Münch and Laepple, 2018). Both studies indicate a timescale-dependent signal-to-noise ratio in paleoclimate time series. This timescale dependence of proxy uncertainty is explored further in two companion papers (Kunz et al., 2020; Dolman et al., 2021). In part one, Kunz et al. (2020) develop a theoretical framework for a spectral approach to estimate timescale-dependent uncertainty, and this concept is applied in part two (Dolman et al., 2021). The studies above focussed on geochemical proxy sensors, whereas Jonkers and Kučera (2019) present an approach to detect noise in reconstructions based on the taxonomic composition of microfossil assemblages. A final contribution to the set of papers exploring paleoclimate data uncertainty provides a first attempt to quantify the error due to uncertainty in temporal representativeness (Amrhein, 2020). The issue of the accuracy of paleoclimate observations in representing climate at specific times and during time intervals is particularly relevant for paleoclimate data–model integration of transient simulations.
Together these papers provide important new constraints on the uncertainty of paleoclimate data. They present new ways to detect and quantify various aspects of the uncertainty and thus present new tools for the paleoclimate (data) community. The spectral approach comes together with open-source code (Dolman et al., 2021) to facilitate broad use.
Because the transfer of climate signals to the geological archive and the extraction of the proxy signal involve many steps, paleoclimate data reflect climate with some degree of distortion or bias. This makes direct comparison between paleoclimate data and model output complex. However, many aspects of the incorporation of climate signals within proxies are systematic. Proxy forward models can be used to encode this recording process and can hence serve as an important bridge between paleoclimate simulations and paleoclimate observations. Two examples of proxy forward models for sedimentary archives are presented in this special issue (Dolman and Laepple, 2018; Bothe et al., 2019). Both make code available for future use of these forward models in paleoclimate data–model integration.
Whereas proxy forward modelling uses climatic input to produce hypothetical proxy records, another approach is illustrated in two articles in the special issue that present results on explicitly including the simulation of a paleoclimate proxy property in models. Cauquoin et al. (2019) present simulated estimates of the relation between climate and water isotopes, which can improve our understanding of interactions within the climate system and also of proxy systems based on water isotopes. In addition, Breil et al. (2021) show how isotope-enabled simulations can well represent the paleo-observations of isotopes for a specific region and how we can use not only global earth system models, but also regional climate models for the study of past climate.
Offline data assimilation is a method to fill the gaps in (spatio-temporal) coverage of paleoclimate data using physical constraints from climate models. The approach relies on a thorough understanding of the climate recording process by proxies in order to take uncertainties into account. When successfully applied, data assimilation is an effective way to derive meaningful climate state estimates from irregularly spaced observations. Different methods are illustrated in three papers in this special issue. Two focus on mid-Holocene climate in Europe. The first describes a computationally efficient approach using optimal interpolation (Fallah et al., 2018) and the second employs a Bayesian framework to integrate paleoclimate and model data (Weitzel et al., 2019). Finally, in a technical note, Bothe and Zorita (2021) apply and test the analogue method to infer climate over the past 21 000 years by using paleoclimate data time series as constraints for a pool of simulation data.
The contributions to this special issue clearly highlight the value of paleoclimate data and of paleoclimate data science. The different synthesis products will undoubtedly prove valuable to the community, especially because they include rich metadata that are crucial for the interpretation of the data and for data–model integration. Nevertheless, the value of the paleoclimate data and the ease of keeping the syntheses up to date could be increased if paleodata archiving followed more strongly community guidelines on standardisation (Khider et al., 2019; Morrill et al., 2021). Whilst the data products in this issue present major steps forward in standardisation, the various products also show that work still needs to be done to harmonise the different syntheses in order to further increase their value (Bothe et al., 2021).
The articles also indicate further aspects that could be considered in paleoclimate data–model integration. Since the focus of this special issue was on the paleoclimate data side, most of the uncertainty assessment focussed on the uncertainties associated with indirect paleoclimate observations. However, as shown by Breil et al. (2021), a meaningful data–model integration framework should also consider climate model uncertainty (Fig. 1). Furthermore, whilst data assimilation presents a promising example of data–model integration, validation of the resulting reconstructions and state estimates deserves additional attention, because when models and paleodata are truly integrated, other information is needed to validate the result.
This special issue provides important approaches to extend the value of paleoclimate data in providing new knowledge about climate proxies, climate models and ultimately the climate system. Collectively the contributions highlight the importance of developing a formal framework for paleoclimate data–model integration that considers uncertainty in the data and in the models. Such a framework should turn disagreement into diagnosis. After all, for a thorough understanding of the climate system, there is as much value in understanding data–model agreement as disagreement.
No data sets were used in this article.
LJ wrote the draft and all the authors reviewed and added the manuscript and discussed the visualisation.
The contact author has declared that neither they nor their co-authors have any competing interests.
This article is part of the special issue “Paleoclimate data synthesis and analysis of associated uncertainty (BG/CP/ESSD inter-journal SI)”. It is not associated with a conference.
Publisher's note: Copernicus Publications remains neutral with regard to jurisdictional claims in published maps and institutional affiliations.
We would like to thank all the scientists who contributed to the success of this special issue and the editors and reviewers for their time and efforts in improving each contribution. The special issue was organized within the paleoclimate data synthesis working group of the PALMOD project (http://www.palmod.de/, last access: 13 December 2021), which also provided funding for Lukas Jonkers and Oliver Bothe. PALMOD is a climate modelling initiative funded by the German Ministry of Science and Education (BMBF).
The article processing charges for this open-access publication were covered by the University of Bremen.
Amrhein, D. E.: How large are temporal representativeness errors in paleoclimatology?, Clim. Past, 16, 325–340, https://doi.org/10.5194/cp-16-325-2020, 2020.
Bothe, O. and Zorita, E.: Technical note: Considerations on using uncertain proxies in the analogue method for spatiotemporal reconstructions of millennial-scale climate, Clim. Past, 17, 721–751, https://doi.org/10.5194/cp-17-721-2021, 2021.
Bothe, O., Wagner, S., and Zorita, E.: Simple noise estimates and pseudoproxies for the last 21 000 years, Earth Syst. Sci. Data, 11, 1129–1152, https://doi.org/10.5194/essd-11-1129-2019, 2019.
Bothe, O., Rehfeld, K., Konecky, B., and Jonkers, L.: Towards increased interoperability of paleoenvironmental observation data, Past Global Change Magazine, 29, 59–59, 2021.
Breil, M., Christner, E., Cauquoin, A., Werner, M., Karremann, M., and Schädler, G.: Applying an isotope-enabled regional climate model over the Greenland ice sheet: effect of spatial resolution on model bias, Clim. Past, 17, 1685–1699, https://doi.org/10.5194/cp-17-1685-2021, 2021.
Cao, X., Tian, F., Li, F., Gaillard, M.-J., Rudaya, N., Xu, Q., and Herzschuh, U.: Pollen-based quantitative land-cover reconstruction for northern Asia covering the last 40 ka cal BP, Clim. Past, 15, 1503–1536, https://doi.org/10.5194/cp-15-1503-2019, 2019.
Cao, X., Tian, F., Andreev, A., Anderson, P. M., Lozhkin, A. V., Bezrukova, E., Ni, J., Rudaya, N., Stobbe, A., Wieczorek, M., and Herzschuh, U.: A taxonomically harmonized and temporally standardized fossil pollen dataset from Siberia covering the last 40 kyr, Earth Syst. Sci. Data, 12, 119–135, https://doi.org/10.5194/essd-12-119-2020, 2020.
Cauquoin, A., Werner, M., and Lohmann, G.: Water isotopes – climate relationships for the mid-Holocene and preindustrial period simulated with an isotope-enabled version of MPI-ESM, Clim. Past, 15, 1913–1937, https://doi.org/10.5194/cp-15-1913-2019, 2019.
Dolman, A. M. and Laepple, T.: Sedproxy: a forward model for sediment-archived climate proxies, Clim. Past, 14, 1851–1868, https://doi.org/10.5194/cp-14-1851-2018, 2018.
Dolman, A. M., Kunz, T., Groeneveld, J., and Laepple, T.: A spectral approach to estimating the timescale-dependent uncertainty of paleoclimate records – Part 2: Application and interpretation, Clim. Past, 17, 825–841, https://doi.org/10.5194/cp-17-825-2021, 2021.
Fallah, B., Russo, E., Acevedo, W., Mauri, A., Becker, N., and Cubasch, U.: Towards high-resolution climate reconstruction using an off-line data assimilation and COSMO-CLM 5.00 model, Clim. Past, 14, 1345–1360, https://doi.org/10.5194/cp-14-1345-2018, 2018.
Fuhrmann, F., Diensberg, B., Gong, X., Lohmann, G., and Sirocko, F.: Aridity synthesis for eight selected key regions of the global climate system during the last 60 000 years, Clim. Past, 16, 2221–2238, https://doi.org/10.5194/cp-16-2221-2020, 2020.
IPCC: Climate Change 2021: The Physical Science Basis. Contribution of Working Group I to the Sixth Assessment Report of the Intergovernmental Panel on Climate Change, edited by: Masson-Delmotte, V., Zhai, P., Pirani, A., Connors, S. L., Péan, C., Berger, S., Caud, N., Chen, Y., Goldfarb, L., Gomis, M. I., Huang, M., Leitzell, K., Lonnoy, E., Matthews, J. B. R., Maycock, T. K., Waterfield, T., Yelekçi, O., Yu, R., and Zhou, B., Cambridge University Press, in press, 2021.
Jonkers, L. and Kučera, M.: Sensitivity to species selection indicates the effect of nuisance variables on marine microfossil transfer functions, Clim. Past, 15, 881–891, https://doi.org/10.5194/cp-15-881-2019, 2019.
Jonkers, L., Cartapanis, O., Langner, M., McKay, N., Mulitza, S., Strack, A., and Kucera, M.: Integrating palaeoclimate time series with rich metadata for uncertainty modelling: strategy and documentation of the PalMod 130k marine palaeoclimate data synthesis, Earth Syst. Sci. Data, 12, 1053–1081, https://doi.org/10.5194/essd-12-1053-2020, 2020.
Khider, D., Emile-Geay, J., McKay, N. P., Gil, Y., Garijo, D., Ratnakar, V., Alonso-Garcia, M., Bertrand, S., Bothe, O., Brewer, P., Bunn, A., Chevalier, M., Comas-Bru, L., Csank, A., Dassié, E., DeLong, K., Felis, T., Francus, P., Frappier, A., Gray, W., Goring, S., Jonkers, L., Kahle, M., Kaufman, D., Kehrwald, N. M., Martrat, B., McGregor, H., Richey, J., Schmittner, A., Scroxton, N., Sutherland, E., Thirumalai, K., Allen, K., Arnaud, F., Axford, Y., Barrows, T. T., Bazin, L., Pilaar Birch, S. E., Bradley, E., Bregy, J., Capron, E., Cartapanis, O., Chiang, H. W., Cobb, K., Debret, M., Dommain, R., Du, J., Dyez, K., Emerick, S., Erb, M. P., Falster, G., Finsinger, W., Fortier, D., Gauthier, N., George, S., Grimm, E., Hertzberg, J., Hibbert, F., Hillman, A., Hobbs, W., Huber, M., Hughes, A. L. C., Jaccard, S., Ruan, J., Kienast, M., Konecky, B., Le Roux, G., Lyubchich, V., Novello, V. F., Olaka, L., Partin, J. W., Pearce, C., Phipps, S. J., Pignol, C., Piotrowska, N., Poli, M. S., Prokopenko, A., Schwanck, F., Stepanek, C., Swann, G. E. A., Telford, R., Thomas, E., Thomas, Z., Truebe, S., von Gunten, L., Waite, A., Weitzel, N., Wilhelm, B., Williams, J., Williams, J. J., Winstrup, M., Zhao, N., and Zhou, Y.: PaCTS 1.0: A Crowdsourced Reporting Standard for Paleoclimate Data, Paleoceanography and Paleoclimatology, 34, 1570–1596, 2019.
Köhler, P., Nehrbass-Ahles, C., Schmitt, J., Stocker, T. F., and Fischer, H.: A 156 kyr smoothed history of the atmospheric greenhouse gases CO2, CH4, and N2O and their radiative forcing, Earth Syst. Sci. Data, 9, 363–387, https://doi.org/10.5194/essd-9-363-2017, 2017.
Konecky, B. L., McKay, N. P., Churakova (Sidorova), O. V., Comas-Bru, L., Dassié, E. P., DeLong, K. L., Falster, G. M., Fischer, M. J., Jones, M. D., Jonkers, L., Kaufman, D. S., Leduc, G., Managave, S. R., Martrat, B., Opel, T., Orsi, A. J., Partin, J. W., Sayani, H. R., Thomas, E. K., Thompson, D. M., Tyler, J. J., Abram, N. J., Atwood, A. R., Cartapanis, O., Conroy, J. L., Curran, M. A., Dee, S. G., Deininger, M., Divine, D. V., Kern, Z., Porter, T. J., Stevenson, S. L., von Gunten, L., and Iso2k Project Members: The Iso2k database: a global compilation of paleo-δ18O and δ2H records to aid understanding of Common Era climate, Earth Syst. Sci. Data, 12, 2261–2288, https://doi.org/10.5194/essd-12-2261-2020, 2020.
Kunz, T., Dolman, A. M., and Laepple, T.: A spectral approach to estimating the timescale-dependent uncertainty of paleoclimate records – Part 1: Theoretical concept, Clim. Past, 16, 1469–1492, https://doi.org/10.5194/cp-16-1469-2020, 2020.
Langner, M. and Mulitza, S.: Technical note: PaleoDataView – a software toolbox for the collection, homogenization and visualization of marine proxy data, Clim. Past, 15, 2067–2072, https://doi.org/10.5194/cp-15-2067-2019, 2019.
Lisiecki, L. E. and Stern, J. V.: Regional and global benthic δ18O stacks for the last glacial cycle, Paleoceanography, 31, 1368–1394, 2016.
MARGO Project Members: Constraints on the magnitude and patterns of ocean cooling at the Last Glacial Maximum, Nat. Geosci., 2, 127–132, https://doi.org/10.1038/ngeo411, 2009.
Morrill, C., Thrasher, B., Lockshin, S. N., Gille, E. P., McNeill, S., Shepherd, E., Gross, W. S., and Bauer, B. A.: The Paleoenvironmental Standard Terms (PaST) Thesaurus: Standardizing heterogeneous variables in paleoscience, Paleoceanography and Paleoclimatology, 36, e2020PA004193, https://doi.org/10.1029/2020pa004193, 2021.
Münch, T. and Laepple, T.: What climate signal is contained in decadal- to centennial-scale isotope variations from Antarctic ice cores?, Clim. Past, 14, 2053–2070, https://doi.org/10.5194/cp-14-2053-2018, 2018.
Paul, A., Mulitza, S., Stein, R., and Werner, M.: A global climatology of the ocean surface during the Last Glacial Maximum mapped on a regular grid (GLOMAP), Clim. Past, 17, 805–824, https://doi.org/10.5194/cp-17-805-2021, 2021.
Peterson, C. D. and Lisiecki, L. E.: Deglacial carbon cycle changes observed in a compilation of 127 benthic δ13C time series (20–6 ka), Clim. Past, 14, 1229–1252, https://doi.org/10.5194/cp-14-1229-2018, 2018.
Ramisch, A., Brauser, A., Dorn, M., Blanchet, C., Brademann, B., Köppl, M., Mingram, J., Neugebauer, I., Nowaczyk, N., Ott, F., Pinkerneil, S., Plessen, B., Schwab, M. J., Tjallingii, R., and Brauer, A.: VARDA (VARved sediments DAtabase) – providing and connecting proxy data from annually laminated lake sediments, Earth Syst. Sci. Data, 12, 2311–2332, https://doi.org/10.5194/essd-12-2311-2020, 2020.
Reschke, M., Rehfeld, K., and Laepple, T.: Empirical estimate of the signal content of Holocene temperature proxy records, Clim. Past, 15, 521–537, https://doi.org/10.5194/cp-15-521-2019, 2019.
Tierney, J. E., Poulsen, C. J., Montañez, I. P., Bhattacharya, T., Feng, R., Ford, H. L., Hönisch, B., Inglis, G. N., Petersen, S. V., Sagoo, N., Tabor, C. R., Thirumalai, K., Zhu, J., Burls, N. J., Foster, G. L., Goddéris, Y., Huber, B. T., Ivany, L. C., Kirtland Turner, S., Lunt, D. J., McElwain, J. C., Mills, B. J. W., Otto-Bliesner, B. L., Ridgwell, A., and Zhang, Y. G.: Past climates inform our future, Science, 370, eaay3701, https://doi.org/10.1126/science.aay3701, 2020.
Weitzel, N., Hense, A., and Ohlwein, C.: Combining a pollen and macrofossil synthesis with climate simulations for spatial reconstructions of European climate using Bayesian filtering, Clim. Past, 15, 1275–1301, https://doi.org/10.5194/cp-15-1275-2019, 2019.
“Paleoclimate data” here refers to data from paleoenvironmental observations. Output from paleoclimate simulations is explicitly referred to when it is mentioned.