the Creative Commons Attribution 4.0 License.
the Creative Commons Attribution 4.0 License.
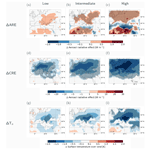
Effects of land use and anthropogenic aerosol emissions in the Roman Empire
Anina Gilgen
Stiig Wilkenskjeld
Jed O. Kaplan
Thomas Kühn
Ulrike Lohmann
As one of the first transcontinental polities that led to widespread anthropogenic modification of the environment, the influence of the Roman Empire on European climate has been studied for more than 20 years. Recent advances in our understanding of past land use and aerosol–climate interactions make it valuable to revisit the way humans may have affected the climate of the Roman era. Here we estimate the effect of humans on some climate variables in the Roman Empire at its apogee, focusing on the impact of anthropogenic land cover and aerosol emissions. For this we combined existing land use scenarios with novel estimates (low, medium, high) of aerosol emissions from fuel combustion and burning of agricultural land. Aerosol emissions from agricultural burning were greater than those from fuel consumption but of the same order of magnitude.
Using the global aerosol-enabled climate model ECHAM-HAM-SALSA, we conducted simulations with fixed sea-surface temperatures to gain a first impression about the possible climate impact of anthropogenic land cover and aerosols in the Roman Empire. While land use effects induced a regional warming for one of the reconstructions caused by decreases in turbulent flux, aerosol emissions enhanced the cooling effect of clouds and thus led to a cooling in the Roman Empire. Quantifying the anthropogenic influence on climate is, however, challenging since our model likely overestimates aerosol-effective radiative forcing and prescribes the sea-surface temperatures.
- Article
(5868 KB) - Full-text XML
-
Supplement
(1472 KB) - BibTeX
- EndNote
Humans shaped the European landscape thousands of years before the Industrial Revolution (Kaplan et al., 2016), primarily through deforestation, which was applied for several purposes: less dense forests facilitated hunting, foraging, and mobility (Kaplan et al., 2016), removal of trees was required for most forms of agricultural land use (Klein Goldewijk et al., 2011), and wood was harvested for fuel or as timber for manufacturing and building purposes (Harris, 2013). The replacement of forests by other vegetation types influences climate through both biogeochemical and biogeophysical effects (Bathiany et al., 2010). Biogeochemical effects occur primarily through changes in the chemical composition of the atmosphere, e.g. transfers of carbon from land to the atmosphere, where it resides as the greenhouse gas CO2. Because atmospheric CO2 is long-lived and well mixed, biogeochemical effects have a global impact on climate (Boysen et al., 2014). In contrast, biogeophysical effects rather act at regional scale. These include changes in physical land surface characteristics, such as roughness length or surface albedo (Claussen et al., 2001). Increases in surface albedo due to deforestation usually lead to a cooling, while changes in energy redistribution (evaporative fraction and turbulent flux) are associated with a warming (Lee et al., 2011). The net effect of biogeophysical effects on temperature is a strong function of radiation and thus latitude (Lee et al., 2011; Y. Li et al., 2016b): in the tropics, changes in energy redistribution usually dominate; as a consequence, deforestation induces a net warming. In high latitudes, changes due to surface albedo are generally more important, and deforestation thus leads to a net cooling.
Smith et al. (2016) modelled the impact of biogeophysical effects during the Holocene and found that anthropogenic land cover change in Europe and East Asia already had significant impacts on climate (temperature, precipitation, and near-surface wind speed) a few thousand of years ago. They used the reconstruction of anthropogenic land cover change from Kaplan and Krumhardt (KK10; Kaplan et al., 2009, 2011) for their simulations.
Humans also affect the climate by aerosol emissions. Aerosol particles absorb and scatter radiation, which leads to a radiative forcing due to aerosol–radiation interactions (RFari). Furthermore, aerosols affect clouds by heating atmospheric layers when they absorb a considerable amount of the solar radiation (Koch and Del Genio, 2010). Depending on the cloud type and the position of the aerosol layer relative to the cloud, cloud cover can either decrease or increase (Koch and Del Genio, 2010). Together with RFari, these absorption-related adjustments are called ERFari (effective radiative forcing due to aerosol–radiation interactions).
Aerosols further impact radiation indirectly by influencing the number of cloud droplets and ice crystals. Aerosols that activate into cloud droplets are called cloud condensation nuclei (CCN). If the CCN concentration increases at a fixed cloud liquid water content, then the cloud droplet number concentration (CDNC) generally also increases, which enhances the backscattering of radiation (Twomey, 1974, 1977). This effect is called RFaci (radiative forcing due to aerosol–cloud interactions; Boucher et al., 2013) and generally increases the cooling effect of clouds. An increase in CCN (and thus CDNC) can also have other effects, e.g. affecting the cloud lifetime by decelerating collision–coalescence (Albrecht, 1989). Together with RFaci, such adjustments are called ERFaci (effective radiative forcing due to aerosol–cloud interactions; Boucher et al., 2013).
It is not straightforward to disentangle all these different aerosol effects. Here, we define the aerosol radiative effect (ARE) and the cloud radiative effect (CRE) as the differences between radiative fluxes at the top of the atmosphere in the presence and absence of aerosols and clouds, respectively. These quantities can be calculated with a climate model by a double call of the radiation scheme, once with and once without aerosols or clouds.
Present-day anthropogenic aerosol emissions are very high compared to pre-industrial emissions. However, when the effect of anthropogenic aerosol emissions on the radiative balance is quantified, it makes a difference whether 1850 CE or 1750 CE is chosen as the reference year (Carslaw et al., 2017). This shows that anthropogenic aerosol emissions probably already had an impact on climate in 1850 CE. But when did anthropogenic aerosol emissions start to change the climate? Is it possible that locally significant changes already occurred thousands of years ago?
In the first century CE, the Roman Empire was at its apogee, with a “surprisingly high standard of living” for antiquity (Temin, 2006). Around the same time, the Han dynasty in China also controlled a large part of the world's population. These two empires had comparable spatial extents and population sizes (Bielenstein, 1986; Scheidel, 2009). Although the global population was approximately 2.6 and 5.5 times smaller in 1 CE than in 1700 CE and 1850 CE, respectively (Klein Goldewijk et al., 2017), industrial activities between 100 BCE and 200 CE in both Europe and East Asia left imprints in ice cores and sedimentary records, for example heavy metals and 13C-enriched methane (Hong et al., 1996; Brännvall et al., 1999; Sapart et al., 2012). In cities and towns during antiquity, smoke emissions were sufficiently severe to darken buildings and to enforce laws against air pollution (Makra, 2015).
Of course, these activities are by no means comparable to the anthropogenic impact on climate under present-day conditions. Nevertheless, we hypothesise that these anthropogenic activities could already have had an influence on climate on a continental scale. The goal of this study is to gain a first impression on whether or not anthropogenic land cover change and aerosol emissions could have already influenced climate in antiquity. We use the global aerosol–climate model ECHAM-HAM-SALSA for this assessment. Since the sea-surface temperatures (SSTs) are prescribed in all simulations, ocean–atmosphere feedbacks are disabled and the temperature and precipitation responses are dampened. Nevertheless, the results provide valuable information about changes in variables such as surface albedo, turbulent flux, ARE, and CRE.
To analyse the influence of land use on climate, we compare a control simulation without land use to simulations including crop and pasture areas representative for 100 CE (Sect. 3.1). Two different reconstructions of anthropogenic land cover were used. Since ECHAM-HAM-SALSA does not calculate a full carbon cycle, we only investigate biogeophysical effects and not the biogeochemical effects. The impact of anthropogenic land cover on secondary organic aerosol (SOA) precursors is simulated.
To assess the impact of anthropogenic aerosol emissions, we first constructed three possible scenarios (called “low”, “intermediate”, and “high”) of emissions representative for 100 CE. We considered emissions from fuel consumption, crop residue burning, and pasture burning. The magnitude of these new aerosol emissions is compared with that of natural fire emissions in Sect. 3.2 and with that of anthropogenic emissions in 1850 CE in Sect. 3.3. Simulations with and without anthropogenic aerosol emissions were compared to quantify their impact on certain climate variables (Sect. 3.4).
We conducted several simulations (Sect. 2.8) with an aerosol–climate model (Sect. 2.1). The simulations aim to represent the Roman Empire at its maximum extent around 100 CE (Fig. S1 in the Supplement). Our study domain is a box between 10∘ W–50∘ E and 20–60∘ N, which fully encompasses the Roman Empire at that time. We acknowledge that this definition includes some regions that were not part of the Roman Empire, such as the highly populated northern Germany, but drawing precise boundaries was challenging due to the coarse spatial resolution of our model, and many of the land use and industrial activities present within the political boundaries of the Roman Empire at this time also occurred outside it. Since the main focus of our study lies on the anthropogenic influence on climate, and humans near but outside the Roman Empire also carried out agriculture and emitted aerosols, our main conclusions should not be affected by the exact geographical definition. Similarly, some boundary conditions (vegetation, fire emissions) refer to a somewhat earlier period than 100 CE (e.g. 1 CE) due to data availability, but concerning the large temporal uncertainties when going so far back in time, we do not consider this to be an issue.
Associated with these experiments, we needed data for (i) the boundary conditions (Sect. 2.2), (ii) anthropogenic land cover change (Sect. 2.3), (iii) natural aerosol emissions (Sect. 2.4), and (iv) anthropogenic aerosol emissions (Sect. 2.5, 2.6, 2.7).
2.1 Model
To study the potential anthropogenic effects of land cover and aerosols, we used the global aerosol–climate model ECHAM6.3-HAM2.3-SALSA2.0. SALSA stands for Sectional Aerosol module for Large Scale Applications (Kokkola et al., 2008; Bergman et al., 2012; Kokkola et al., 2018). The aerosol size distribution is described with 10 size sections. The aerosol species black carbon (BC), organic matter (, where OC stands for organic carbon), sulfate (SO4), dust, and sea salt are considered. Particles below r=25 nm are comprised exclusively of OM and/or SO4, whereas larger particles can contain any aerosol species.
The land component of ECHAM-HAM-SALSA is called JSBACH (Jena Scheme for Biosphere–Atmosphere Coupling in Hamburg; Raddatz et al., 2007). In our simulations, heterogeneity in each grid box is represented by geographically varying fractions of 12 different plant functional types. The implementation of anthropogenic land cover change in JSBACH is described in Reick et al. (2013).
2.2 Boundary conditions
The greenhouse gas concentrations follow Meinshausen et al. (2017). Both the greenhouse gas concentrations and the orbital parameters were averaged over 50–150 CE (Table S1 in the Supplement). To prescribe natural vegetation fractions (Sect. 2.3), SSTs, and sea ice concentration (SIC) we used output from a simulation with the Earth system model MPI-ESM (Bader et al., 2019, Fig. 1), called MPI_no_LCC in the following. The annual cycle of SSTs and SIC was derived by averaging the MPI_no_LCC output from 50 to 150 CE. The MPI-ESM has the same atmospheric core (ECHAM) and uses the same vegetation model (JSBACH) as ECHAM-HAM-SALSA. MPI_no_LCC ran from 6000 BCE to 1850 CE and considered slow forcings, i.e. changes in greenhouse gases (CO2, CH4, N2O) and orbital parameters, but no anthropogenic land cover change. Vegetation was calculated dynamically (Brovkin et al., 2009).
For the simulated sulfur cycle and SOA calculation, climatologies for the following oxidants are needed: H2O2 (only for sulfur), O3, OH, and NO3. In general, it is uncertain how these oxidants have changed over the past millennia. OH concentrations seem to be relatively stable (Pinto and Khalil, 1991; Lelieveld et al., 2002; Murray et al., 2014). In contrast, ice core measurements suggest that H2O2 has increased by >50 % over the last 200 years (Sigg and Neftel, 1991; Anklin and Bales, 1997). Modelling studies suggest that NO3 and O3 have also increased since the pre-industrial (Khan et al., 2015; Murray et al., 2014). For O3, Pinto and Khalil (1991) and Crutzen and Brühl (1993) found that the changes are relatively small between the glacial and pre-industrial; however, the ozone profile shows larger changes between pre-industrial and present-day conditions (Crutzen and Brühl, 1993). In line with this, we expect that differences in oxidant concentrations are larger between 1850 CE and the present day than between 100 CE and 1850 CE due to large anthropogenic emissions of various gases since 1850 CE. Therefore, we used climatological monthly mean mixing ratios of oxidants representative for 1850 CE conditions (Fig. 1). They were derived from simulations with the Community Earth System Model version 2.0 (CESM2.0) Whole Atmosphere Community Climate Model (WACCM1).
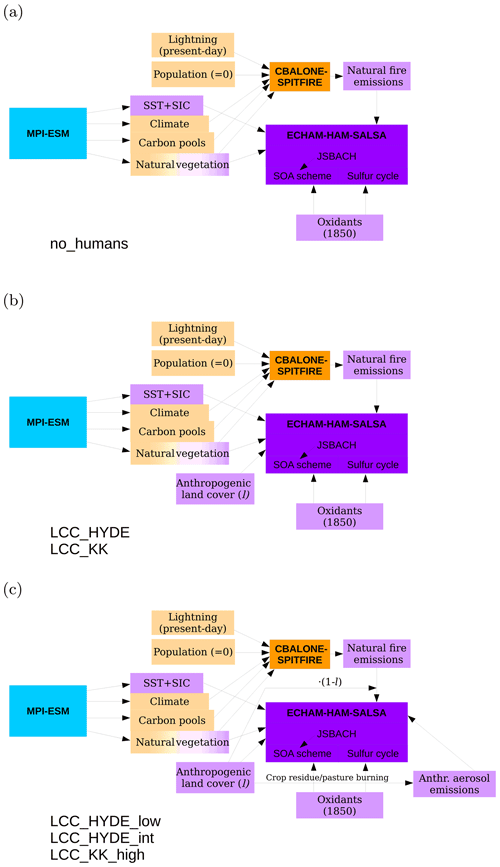
Figure 1Illustrated are the setups of the six simulations conducted with ECHAM-HAM-SALSA (no_humans, LCC_HYDE, LCC_KK, LCC_HYDE_low, LCC_HYDE_int, and LCC_KK_high). Models that we used are shown in dark colours, whereas inputs to these models are shown in light colours. ECHAM-HAM-SALSA (violet) includes (among other components) the vegetation model JSBACH, a secondary organic aerosol scheme, and a sulfur cycle. Natural fire emissions were calculated with CBALONE–SPITFIRE (orange). For driving the two models, output from the Earth system model MPI-ESM was used (blue; simulations come from the study by Bader et al., 2019) among others.
2.3 Vegetation and land cover change
In our simulations with ECHAM-HAM-SALSA, natural vegetation was not dynamic. The coverages of different natural vegetation types representative for 100 CE were taken from MPI_no_LCC (Fig. 1). These natural vegetation fractions are fixed over time. They are from an earlier year than 100 CE (end of year 10 BCE) because vegetation around 1 CE was used to calculate the fire emissions (Sect. 2.4).
For the sensitivity simulations in ECHAM-HAM-SALSA, we used two different reconstructions to estimate anthropogenic land cover fractions around 100 CE: the anthropogenic land cover reconstructions from KK11 (an update of KK10; Kaplan et al., 2011, 2012) and the reconstructions from the HYDE database version 3.1 (Klein Goldewijk et al., 2010, 2011, HYDE11 in the following). The empirical model of KK11 assumes that per capita land use declines over time. In contrast, the reconstruction of cropland and pasture from HYDE11 assumes a nearly constant per capita land use hindcasting approach with allocation algorithms that change over time (Klein Goldewijk et al., 2017). As a consequence, the anthropogenic land cover fraction in the past is considerably higher in the estimate by KK11 compared to the estimate of HYDE11.
KK11 provides information about the fraction of a grid box subject to anthropogenic land use. For our simulations, we interpolated the values for 1 CE from a grid to the Gaussian grid of ECHAM-HAM-SALSA and assumed that the same fraction of natural vegetation is converted to crop and pasture in equal shares. This seems to be a good 1st-order approximation: in the reconstruction of HYDE11, which estimated pasture and crop areas separately, they contribute each roughly 50 %, both when averaged over the whole world and when averaged over the study domain. However, there are of course regions (both in HYDE11 and in reality) where either crop or pasture dominated.
HYDE11 provides information about the area of crop and pasture (square kilometres per grid cell) on a 5' grid. We divided these areas by the maximum land area available per grid cell (also from HYDE11) to get fractions of crop and pasture per land area and interpolated the values to the Gaussian grid of ECHAM-HAM-SALSA.
The anthropogenic land cover fractions were scaled to the fraction of the vegetable part of the model grid. Due to inconsistencies between the datasets and the model setup, including differences in the land–sea mask, the actually applied land use changes are smaller than prescribed in the original datasets. In our model, the total crop areas in the study domain amount to 5.46×105 and 13.8×105 km2 for HYDE11 and KK11, respectively (i.e. 47 % and 21 % lower than the original estimate). For pasture, the total areas add up to 4.75×105 and 13.8×105 km2, respectively (47 % and 12 % lower than the original estimate). For comparison, the total land area in the study domain is 163×105 km2 in our model. Part of the underestimation in both datasets is due to the binary land–sea mask of JSBACH, which can lead to an underestimation along the coastlines: no crop or pasture can grow in grid boxes that are considered to be ocean in JSBACH, even if the anthropogenic land cover fraction from the original estimate would be larger than zero at this location. The underestimation is considerably more pronounced for KK11 since some areas that are subject to land use in this reconstruction are hardly hospitable to plants in JSBACH. Examples are the Arabian Peninsula, which is and presumably was in reality mainly covered by desert, and parts of North Africa. For instance, the area subject to land use between 35–50∘ E and 20–35∘ N (roughly corresponding to the part of the Arabian Peninsula lying within our study domain) amounts to 12.5×105 km2 in the original estimate of KK11 but only 2.28×105 km2 in the model.
2.4 Natural aerosol emissions
Sea salt, dust, and oceanic dimethylsulfide (DMS) emissions are calculated online as described in Tegen et al. (2019). Tropospheric SO2 emissions from volcanoes are based on Andres and Kasgnoc (1998) and Halmer et al. (2002) as described in Stier et al. (2005).
To estimate SOA formation from biogenic sources, a volatility basis set (VBS) was used (Kühn et al., 2019; Mielonen et al., 2018; Stadtler et al., 2018). The VBS uses three volatility bins following Farina et al. (2010), which are classified as non-volatile (log(inf), low-volatile (log(), and semi-volatile (log(), where C* is the equilibrium vapour concentration (g m−3) over a flat surface at 298 K. Gas-to-particle partitioning is computed using a kinetic algorithm after Jacobson (2005). The volatile SOA precursors (monoterpenes and isoprene) are converted into SOA-forming species using a simplified one-step oxidation chemistry (Sect. 2.2) and grouped into the volatility bins using predefined partitioning coefficients. The emission strengths of the biogenic SOA precursors were calculated online based on the vegetation in the model (Henrot et al., 2017). As a consequence, all ECHAM-HAM-SALSA simulations that include anthropogenic land use account for the effect of these land cover changes on SOA precursor emissions (Table S2). DMS emissions from terrestrial sources were set to present-day values (Pham et al., 1995); the emissions are very low compared to oceanic DMS emissions, though.
Fires have played an important role in shaping the composition and structure of Mediterranean vegetation communities (Naveh, 1975). To simulate past natural fire emissions, we used a stand-alone version of the carbon and vegetation dynamics submodel of JSBACH (CBALONE; called CBALANCE in Wilkenskjeld et al., 2014) together with the fire submodel SPITFIRE (Thonicke et al., 2010; Lasslop et al., 2014; Rabin et al., 2017). CBALONE–SPITFIRE needs forcing data related to the vegetation and the atmosphere at a daily time resolution as well as a starting point for the carbon pools. To drive CBALONE–SPITFIRE we used data from MPI_no_LCC (illustrated in Fig. 1) from which output at a high temporal resolution was saved for a few selected 30-year periods (among them a period around 1 CE and one around 1835 CE). The driving data for the 100 CE CBALONE–SPITFIRE simulations thus represent a slightly earlier period (around 1 CE). However, we do not expect a significant difference between 1 CE and 100 CE since the forcing in MPI_no_LCC is very similar for the two periods. For simplicity we thus refer to the 1 CE fire emissions as those of 100 CE. The 30-year period around 1 CE was repeatedly used to drive both the spin-up (≈100 years) and the analysed simulated period of CBALONE–SPITFIRE. The emissions have a daily resolution and show interannual variability.
We also calculated fire emissions around 1835 CE (not shown). These emissions were not used as input for our Roman Empire ECHAM-HAM-SALSA simulations but for comparison with the fire emissions from van Marle et al. (2017, Sect. S9). We again used high-time-resolution output from an MPI-ESM simulation (hereinafter MPI_LCC) to drive CBALONE–SPITFIRE. The only difference between MPI_no_LCC and MPI_LCC is that the latter considers anthropogenic land cover change.
Next to carbon pools and variables related to the vegetation or the atmosphere, the fire model depends on the following additional inputs: (i) lightning; (ii) population density; and (iii) a regionally varying anthropogenic influence factor an.
To estimate the lightning frequency, we tested the parameterisation by Magi (2015) that is based on convective precipitation fluxes. We found that the such-derived lightning frequency is very similar for 100 CE and 1835 CE. However, the simulated lightning frequency differs from the standard lighting frequency used by the MPI-ESM implementation of SPITFIRE (e.g. different spatial pattern; not shown), which is based on the lightning imaging sensor–optical transient detector (LIS–OTD) as described in Lasslop et al. (2014). The difference between the simulated and observation-based lightning frequency is probably associated with the high uncertainties in the parameterised convection scheme. Furthermore, the parameterisation by Magi (2015) works better for convective mass flux through the 0.44 hybrid-sigma pressure level than for convective precipitation, but the former was not available from the MPI-ESM simulations. Thus, we decided to use the observationally derived lightning frequencies by LIS–OTD for both 100 CE and 1835 CE (Fig. 1) in order to not introduce a large bias in natural fire ignitions.
For the CBALONE–SPITFIRE simulations around 1835 CE, we averaged the population density from HYDE11 over the years 1830 and 1840. Furthermore, we considered anthropogenic land cover change when calculating the fire emissions; the same changes were used as in MPI_LCC. For 100 CE, we used CBALONE–SPITFIRE to calculate natural fire emissions (Fig. 1), assuming no anthropogenic influence. Hence, no anthropogenic land cover change was considered in these CBALONE–SPITFIRE simulations. Furthermore, the population density was set to 0. The ECHAM-HAM-SALSA simulations LCC_HYDE_low, LCC_HYDE_int, and LCC_KK_high (Sect. 2.8) account for anthropogenic aerosol emissions, which include agricultural burning. Using the same natural fire emissions in these simulations as in the other simulations (no_human, LCC_HYDE, and LCC_KK) would lead to an overestimation in total aerosol emissions since natural aerosol emissions should not occur where crop or pasture now grows. Therefore, we reduced the natural fire emissions of the simulations LCC_HYDE_low, LCC_HYDE_int, and LCC_KK_high offline to account for regions subject to anthropogenic land use (Table S2; Fig. 1). As a 1st-order approximation, the natural fire emissions calculated with CBALONE–SPITFIRE were multiplied with (1−l), where l is the fraction of the vegetated area per grid box that is covered by crop and pasture.
Humans impact fires directly by ignitions and fire fighting as well as indirectly, e.g. by forest management and landscape fragmentation (Arora and Melton, 2018). It is very likely that the relationship between humans and fires has changed in the past centuries and millennia as a consequence of large cultural and political changes. This is neglected in SPITFIRE since an, which reflects cultural differences, does not change over time. Instead, an (and thus the number of anthropogenic ignitions) in SPITFIRE is tuned towards present-day fires where satellite data are available. For estimating the fire emissions in 1835 CE, we nevertheless used CBALONE–SPITFIRE with the standard an, as was done by the FIREMIP project for calculating fire emissions from 1750 to today (Rabin et al., 2017). For calculating the (natural) fire emissions around 100 CE, an is irrelevant because the population density is set to zero.
2.5 Aerosol emissions from fuel consumption
Many variables influencing the anthropogenic aerosol emissions are highly uncertain. Therefore, three sets of variables respectively leading to low, intermediate, and high aerosol emissions were estimated for the Roman Empire based on the literature. Figure 3 illustrates how much these scenarios differ.
Anthropogenic emissions associated with both fuel consumption and agricultural burning were likely to have had pronounced regional variations. Despite this variability, we tried to estimate values representative for the whole of our study domain for variables such as fuel consumption and fuel load.
We treated the anthropogenic emissions in the same way as natural fire emissions, except for the emission height. In contrast to the natural fire emissions, for which the simulated emission profile depends on the planetary boundary layer (Veira et al., 2015), we emitted the anthropogenic aerosols at the surface.
For fuel consumption, the aerosol emission (EM) of species i (kgaerosol m−2 s−1) was estimated using the following equation:
where cons (kgdry_fuel capita−1 s−1) is the fuel consumption per capita, popd (capita m−2) is the population density, and EF is the aerosol emission factor of species i (kgaerosol kg). In the following, we derive estimates for these three variables for the different emission scenarios. We assume that the three variables are independent when calculating the emissions.
2.5.1 Fuel consumption per capita
In the Roman Empire, people produced aerosol particles by burning several types of fuel for different purposes such as cooking, residential heating, heating bathhouses, iron production, glass making, pottery production, and cremation (Malanima, 2013; Veal, 2017; Mietz, 2016; Janssen et al., 2017). For iron production, high temperatures are needed, which were only achieved by burning charcoal (Janssen et al., 2017). For other purposes, wood or agricultural waste products (e.g. olive pits or dung) were also used (Mietz, 2016). The Roman Empire consisted of different regions (e.g. rural versus urban; wetter versus drier climate) with different fuel consumption per capita and different fuel strategies. Presently, it is therefore not possible to estimate the fuel consumption with large confidence.
Malanima (2013) estimates that 1–2 kg of wood was consumed per capita per day in the ancient Mediterranean region. His numbers refer to fuel for residential heating and cooking, while he states that industrial contributions were negligible. In present-day developing countries that mainly depend on fuelwood for producing energy (sometimes used as surrogates for past conditions), typical estimates of domestic fuelwood consumption also range from 1 to 2 kg capita−1 d−1 according to Wood and Baldwin (1985). The values in Yevich and Logan (2003) are also in the same range: for Africa (year 1985), the mean and median of fuelwood consumption over the countries considered are approximately 1.5 kg capita−1 d−1 (assuming 15 % moisture content).
Based on the quantitative model from Pompeii, Veal (2017) applied two extreme scenarios for Rome in which the low and the high estimates for fuel consumption are 1 and 2 t capita−1 yr−1. This corresponds to 2.7 and 5.4 kg capita−1 d−1, respectively, which is 2.7 times larger than the estimates by Malanima (2013). The fuel estimates by Veal (2017), however, refer to fuel (i.e. the sum of wood and charcoal) in contrast to the estimates by Malanima (2013), which refer to wood (either burnt directly or used for charcoal making; he assumes little contribution from the latter). This distinction is important when calculating emissions because several kilogrammes of wood are needed to make 1 kg of charcoal, making the difference between the two estimates even larger. While Veal's model derived for Pompeii might give reasonable results for Rome, it is likely not applicable to all parts of the Roman Empire, e.g. in the countryside. Nevertheless, it shows that the fuel consumption in the Roman Empire might have been substantially larger than suggested by Malanima (2013), since the estimates of Veal (2017) account for all types of fuel consumption, including e.g. baths and industrial activities. Recently, Janssen et al. (2017) calculated the wood consumption for the city Sagalassos (2500–3500 inhabitants) to range between 0.6 and 0.8 kg capita−1 d−1 for local pottery production and 1.3–3.4 kg capita−1 d−1 for heating the bath (oven dry wood). Although Sagalassos might differ from other places, this indicates that the neglect of non-residential sources by Malanima (2013) might not be justified, at least in some regions. Based on these different studies, we use 1.5, 3, and 5 kg of fuel (expressed as wood, wood used for charcoal making, or agricultural waste on a dry fuel mass basis) per capita per day for the low, intermediate, and high emission scenarios, respectively (Table 1).
Although more fuel for heating was generally consumed where and when it was cold (Malanima, 2006; Warde, 2006), we assume a constant fuel consumption over the year and over latitudes since we do not differentiate between heating, cooking, iron production, and other burning activities in our calculation. Calculating emissions for individual sectors can be very challenging; as an example, recycling of glass was common (Stern, 1999; Freestone, 2015), which needs to be considered when estimating fuel consumption associated with glass making. Given the large uncertainties about the relative importance of residential heating for total fuel consumption, this seems justified as a 1st-order approximation.
Table 1The values used to calculate the aerosol emissions from fuel consumption (Eq. 1) for the low, intermediate, and high scenarios. The total population in the study domain is shown instead of the population density (popd, used in the equation) since this is more intuitive.
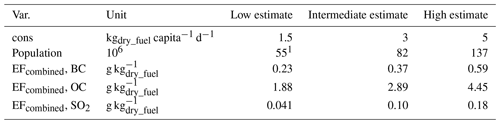
1 Klein Goldewijk et al. (2010, 2011).
2.5.2 Population size
We base the population density of our study on HYDE11 for the year 100 CE (for sources see Klein Goldewijk et al., 2010, 2011). We divided the population counts from HYDE11 (5' grid) by the grid box area before interpolating the such-derived population densities to the Gaussian grid of ECHAM-HAM-SALSA. Using this approach, the HYDE11 population size between 10–50∘ E and 20–60∘ N is around 55 million people (the number of people living within the political boundaries of the Roman Empire would be somewhat smaller). However, the population size of the Roman Empire is still debatable since there is disagreement about what the census tallies represent. The number of HYDE11 lies in the range of the so-called “low count” scenario (Scheidel, 2008), but there are also proponents of a “middle count” and a “high count” hypothesis (Hin, 2013; Scheidel, 2008). With the middle count approach, Hin (2013) arrived at 6.7 million (range between 5 and 10 million) free citizens in Italy compared to approximately 4 million with the low count for 28 BCE. Assuming a constant ratio between free citizens and all people and a similar scaling factor outside Italy, the population in the Roman Empire would be a factor of 1.25–2.5 higher in the middle count approach compared to the low count approach. The high count would result in a population size roughly 3 times larger, i.e. more than 100 million people (maybe up to 160 million) living in the whole empire if we assume that the population densities in other parts of the empire were similar to Italy (Scheidel, 2008, 2009).
Based on these different literature values, we used the estimate from HYDE11 for our low emission scenario. For the intermediate and high emission scenarios, we decided to multiply the population densities of the HYDE database with factors of 1.5 and 2.5, respectively.
2.5.3 Aerosol emission factors
Last but not least, we needed to estimate aerosol emission factors from burning biofuel. We compiled an overview of BC, OC, and SO2 emission measurements from different studies (Sect. S10, Table S17). Composite estimates were not considered. We treated BC and elemental carbon (EC) to be the same. We grouped the measurements according to the fuel type, i.e. wood (key 1 in Table S17), agricultural waste (key 2), charcoal burning (key 5), and charcoal production (key 6). Woody agricultural waste was counted as wood. We neglected coal as a fuel type although it was widespread in Roman Britain (Smith, 1997). This is justified since the centres of the classical civilisations (especially the Mediterranean region) were not rich in coal (Malanima, 2013). A few measurements refer to PM10 (i.e. particulate matter with aerodynamic diameters <10 µm) or PM4 instead of PM2.5, but the difference is usually small (a few percent between PM10 and PM2.5 in Turn et al., 1997). Similarly, we neglected the difference between SO2 and SOx since the latter is dominated by SO2.
For the different sectors (i.e. wood, agricultural waste, charcoal burning, and charcoal production), we calculated a lower, an intermediate, and an upper estimate for EF using the following procedure.
-
For all measurements, the mean, the standard deviation, and the number of samples N were collected.
-
If N was larger than 1 but the standard deviation was not given and could not be calculated (e.g. from plots, confidence intervals, or data in the Supplement), we estimated it by assuming a coefficient of variance of 50 % for OC and BC and of 80 % for SO2. We assessed these coefficients of variance from all other observations providing the standard deviation and mean. The samples for which the standard deviation was estimated are marked in Table S17.
-
If the sample size for measurements was given as a range (e.g. “3 or 4”), we always took the lower number as N. When N was larger than 1 but not given, we assumed N=2.
-
Following Bond et al. (2004), we assumed that EFs follow a log-normal distribution. From the sample mean m and standard deviation s, the mean μ and the standard deviation σ of the log-normal distribution were calculated:
-
For each emission sector, we randomly drew samples from the log-normal distributions with the calculated μ and σ.
-
We used three different methods to weight the different samples: (i) every measurement (in Table S17) had the same weight; (ii) the measurements were weighted with N; (iii) every study had the same weight (differentiated by horizontal lines in Table S17). The three weighting methods can result in very different estimates, e.g. for OC emission factors from wood combustion (medians of 2.4, 0.90, and 3.0 g kg−1, respectively).
-
From the randomly generated samples, we calculated the median and the lower and upper quartile for each weighting method (the median is somewhat smaller than the expected value; Bond et al., 2004). The medians of the three weighting methods were then averaged, and the same was done for the quartiles.
We considered the median to be the intermediate estimate for EF and the quartiles to be the lower and upper estimates. We did not choose more extreme percentiles for the lower and upper estimates because the large variability in the measurements of EF reflects the high variability in burning conditions (e.g. smoldering versus flaming), fuels, combustion devices, and measurement devices. We explicitly wanted to consider these different conditions and not only sample from one (or a few) measurement conducted under specific conditions. In general, more measurements for BC and OC than for SO2 are available; however, since SO2 emissions from biomass burning are small, we do not consider this to be an issue. In Sect. S3, the estimated EFs for the different sectors and the weighting of these different sectors are discussed. As described there, we estimate that 20 % of the fuel consisted of agricultural waste, 40 % of charcoal (in terms of wood that needs to be converted to charcoal), and 40 % of wood. The combined aerosol emission factors were thus calculated as
For the intermediate scenario, we inserted the medians for EFagr, EF, and EFwood. For the low and high estimates, the lower and upper quartiles were used, respectively. The values for EFcombined can be found in Table 1.
2.6 Aerosol emissions from crop residue burning
In the Greco-Roman world, fire was widely employed to fertilise fields, to create or regenerate pastures, to control pests, and/or to hunt (Ascoli and Bovio, 2013). In all simulations in which we included the impact of anthropogenic aerosols, we considered the impact of humans on fires by (i) reducing the (natural) fire emissions calculated from CBALONE–SPITFIRE to account for crop and pasture areas (Sect. 2.4) and (ii) estimating fire emissions from pasture and crop residue burning. In the following, we will first estimate aerosol emissions from crop residue burning before deriving estimates for pasture burning.
Next to fallow, crop rotation, and green manuring (i.e. plowing legumes in the soil; White, 1970), burning crop residues on the field was one method to increase the fertility of soils in Roman agriculture (Spurr, 1986). Since crop residues are burnt after harvest, emissions from open crop residue burning have a strong seasonal cycle (for example, as shown for present-day China by J. Li et al., 2016b; Zhang et al., 2016). Today, harvest of cereal in the Mediterranean region takes place approximately from the beginning of May to the end of August2, which is in accordance with summer being the time of harvest in Roman Italy (Spurr, 1986). We thus spread the fire emissions from crop residue burning over these four months; we neglected the fact that a part of the harvest might have been burnt after August due to drying in the field. Since fires can get out of control at very high temperatures and are unlikely to be ignited at very low temperatures (Pfeiffer et al., 2013), we assume that crop burning cannot occur when the monthly surface temperature averaged over 20 years is below 0 ∘C or above 30 ∘C. The emitted mass for these months without burning was shifted to the other months if there were any. The regions where temperature exceeded these thresholds were calculated offline using the surface temperature simulated with ECHAM-HAM-SALSA (without human impact; climatological analysis).
The aerosol emission fluxes (kg m−2 s−1) were calculated with the following equation (adapted from Webb et al., 2013):
where Frcrop is the fraction of the total grid box covered by crop, Y is the crop harvest fresh weight (kgcrop m−2), d is the dry matter content of the yield (–), s is the ratio between the residue mass and the crop yield mass (kgresidue kg), pb (–) is the proportion of residue which is burnt (and not used for other purposes such as fuel consumption), Frcrop_burnt is the fraction of crop that is burnt per time (s−1), Cf is the combustion factor (–), i.e. the proportion of available fuel that is actually burnt, and EFcrop is the emission factor of crop residue (kgaerosol kg). We consider low, intermediate, and high estimates for Frcrop_burnt and EFcrop because these variables have high uncertainties. Since Frcrop, Y, and pb depend on the population size (below), they also have different values for the different scenarios. All values are summarised in Table 2.
Table 2The values needed to calculate the aerosol emissions from crop residue burning (Eq. 5) for the low, intermediate, and high scenarios. The total area of crop in the study domain (Areacrop) is shown instead of the fraction per grid box (Frcrop). The bold values for Y show which values were taken for the calculation, and the values in brackets represent the yields considering fallow.
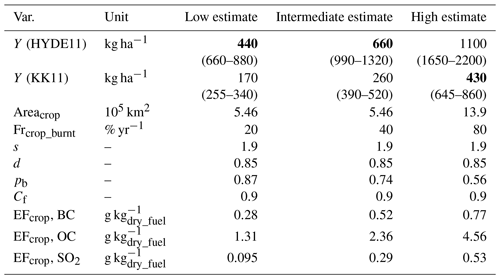
2.6.1 Crop yield Y, fraction of crop Frcrop, and proportion of residue burnt pb
Cereals were the nutritional basis in the Roman Empire (Witcher, 2016) and typical yields were recorded by the agronomists of classical antiquity. Based on ancient sources and the work of Goodchild (2007) and Hopkins (2017), we estimated that yields representative for the whole Roman Empire are in the range between 500 and 1000 kg ha−1 (Sect. S4).
It is fair to assume that the amount of crop produced scaled with the population size. Total crop production depends on both the crop area and the crop yield. The variables Frcrop and Y therefore cannot be estimated independently. Following Kessler and Temin (2007, estimate for Rome), we assumed that around 0.8 kg of wheat (which was the most important nutrient) was consumed per person per day. We roughly estimated that the wheat production was around 50 % higher than the wheat consumption (resulting in 1.2 kg of crop yield per person per day) to consider that a part of the produced crop yield was lost through e.g. transport or insect damage (Spurr, 1986), used for fodder (Spurr, 1986), or needed for seeding (Hopkins, 1980).
For the low emissions scenario in which the population size originates from HYDE11, this wheat production results in a yield of 440 kg ha−1 with the crop area based on HYDE11. For consistency, we use the simulated crop areas from ECHAM-HAM-SALSA (which are somewhat smaller; Sect. 2.3) for this calculation instead of the original input data. This yield is a bit lower than the estimates of crop production mentioned above (500–1000 kg ha−1). However, considering that part of the crop area was used for purposes other than planting cereals or legumes (e.g. vineyards, which were not burnt) and that the crop area estimates from HYDE11 include areas that can lie fallow, the number seems reasonable: if we assume that fallow took place every second or third year (Goodchild, 2007), “actual” crop yields increase from 440 to ≈660–880 kg ha−1. For the intermediate scenario in which population size is larger than in the low emission scenario, the HYDE database results in high but still reasonable estimates of crop yield (660 kg ha−1; ≈990–1320 kg ha−1 if fallow is considered). The high population density in the high emission scenario would require an unrealistically high crop yield when combined with the crop area of HYDE11 (1100 kg ha−1; 1650–2200 kg ha−1 with fallow). When using the KK11 land use reconstruction, which has a much larger crop area, the needed crop yield would be 430 kg ha−1 (≈ 645–860 kg ha−1), which is regarded as realistic. Therefore, the HYDE11 land use is chosen for the low and intermediate scenarios, while KK11 is chosen for the high scenario.
For the yields, we took the values mentioned above (Table 2), which are calculated based on the crop area and the population size, for our calculations. Since our approach assumes a constant wheat consumption per person per day across all scenarios, we ensure that crop yield provides in all cases the necessary food to feed the entire population.
In Sect. 2.5, we assumed that 20 % of the fuel consisted of agricultural waste. Therefore, we should account for the fact that the higher the assumed population in our scenarios, the more crop residue is taken from the field. On the one hand, part of this agricultural waste used as fuel consisted not of cereal crop residue but e.g. of dung or olive pits, which speaks for a larger pb. On the other hand, purposes for crop residue other than fuel also depend directly on the population density, e.g. the residue mass that was used for construction purposes or for filling mattresses (Spurr, 1986), which speaks for a lower pb. Here we simply assume that the crop residue taken from the field per person is equal to 20 % of the consumed fuel mass and thus arrive at pb=0.87, pb=0.74, and pb=0.56 for the low, intermediate, and high scenarios, respectively.
2.6.2 Fraction of crop burnt Frcrop_burnt
Part of the remaining residue on the field was burnt. We assume that the fraction of residue burnt in the field did not depend on the remaining residue mass per area but rather on cultural practices. There are several options for what happened after harvest with the remaining residue on the field: when chaff was short, straw could be used as livestock feed (Spurr, 1986) or the residues left could be directly grazed by ruminants (Spurr, 1986), as happens also nowadays e.g. in Mediterranean North Africa (Yevich and Logan, 2003). However, Spurr (1986) states that animals rarely eat stubble but rather the edible weeds and grass which grow among it. Farmers that had no further use for the stubble left after harvesting burnt it (Spurr, 1986). In ancient sources, burning was mentioned as a method to destroy weeds or to fertilise the soil (Spurr, 1986). Spurr (1986) assumed that especially small farmers who could not store straw and had few animals to feed would burn the stubble.
Nowadays, crop residue burning is no longer widespread in developed countries, and many countries in western Europe even forbid open field burning (Yevich and Logan, 2003). To have a rough indication for how much of the remaining residue might have been burnt in the Roman Empire, we used present-day estimates from developing countries as an indication (including countries that cultivate rice despite the fact that rice was not grown in the Roman Empire). Yevich and Logan (2003) found that in 1985 of the available residue, 1 %, 23 %, and 38 % was burnt in the fields in China, India, and the rest of Asia, respectively. A more recent estimate for crop residue burning in China arrives at much higher fractions than the study by Yevich and Logan (2003): based on satellite data, J. Li et al. (2016a) find that 23 % of the field area is burnt. A large part of the discrepancy can be explained by the different year: Yevich and Logan (2003) derived estimates for 1985, whereas J. Li et al. (2016a) analysed the year 2012. Field surveys from China show that the proportion of residue that is burnt is larger when the crops are harvested by a combine harvester compared to manual harvesting (Yang et al., 2008). In line with this, J. Li et al. (2016b) estimated that the fractions of crop residues burnt in fields increased from 5 % in 1990 to 23 % in 2013. Another reason why crop residue burning has increased might be that the use of biofuels has decreased in rural China (Yang et al., 2008). We do not expect mechanisation to generally lead to enhanced crop residue burning; modern-day technology can also be used to prepare fields for the next crop planting after harvest (Pfeiffer et al., 2013), which makes the burning of crop residue unnecessary.
In the Philippines and Indonesia, up to 65 % and 73 % of the residue, respectively, was burnt in fields in 1985 (Yevich and Logan, 2003). This is similar to the satellite-derived fraction of rice fields under burning for the Punjab region in India in 2015 (66 %; PRSC, 2015); as reasons for the large burning in the Punjab region, highly mechanised farming, poor storage facilities for the straw, and lack of market demand for further use are mentioned among others (PRSC, 2015). A survey in Bangladesh showed that only 3 % of the farmers burn the total residue but that 37 % burn the lower part of it (Haider, 2011); the surveyed farmers manually gathered the residue from the field.
By the use of pb, we have already accounted for the part of residue used as biofuel. If we remove this part of the residue from the estimates above, then the fractions of burning in the field would increase. Based on all the studies that we have mentioned, we estimate that 40 % of the crop area is annually burnt for the simulation LCC_HYDE_int (Frcrop_burnt=0.4 yr−1). For the low and high scenarios, we changed this fraction by a factor of 2 and arrive at 20 % and 80 %, respectively. The 80 % is reached under present-day conditions in the countries with the most crop residue burning (e.g. Indonesia). Pfeiffer et al. (2013) used a value of 20 % in their pre-industrial fire model (LPJ-LMfire v1.0) and consider this to be a conservative estimate. Fallow and the part of the residue taken from the field for fuel combustion are already accounted for in the derivation of Y and pb.
2.6.3 Other variables in Eq. 5
Modern values of the harvest index (the ratio of grain yield to the total plant mass) for wheat range between 0.4 and 0.6 (Hay, 1995). The harvest index was typically lower (around 0.3) at the end of the 19th century (Hay, 1995; Sinclair, 1998), but the sometimes exceptionally high yields in ancient times could indicate that the harvest index might have been higher at this time (Sinclair, 1998). For all simulations, we used a harvest index of 0.35, which corresponds to s=1.9. Following Webb et al. (2013), we chose d=0.85 and Cf=0.9. The emission factors for burning of crop residues on the field were estimated using the same method as described in Sect. 2.5.3 using values from Table S17 (key 3).
2.7 Aerosol emissions from pasture burning
According to the agronomist Columella (who lived in the first century CE), pasture from long fallow was burnt in late summer to achieve more tender growth (Spurr, 1986). Based on Aalde et al. (2006, Eq. 2.27), we calculated the aerosol emission fluxes (kg m−2 s−1) with
where Frpasture is the fraction of the total grid box covered by pasture, Frpasture_burnt is the fraction of pasture that is burnt per time (s−1), F stands for fuel biomass consumption (kgdry_matter m−2; i.e. the amount of fuel that is actually burnt), and EFpasture is the emission factor of pasture burning (kgaerosol kg). In accordance with the crop residue burning emissions, we used the land cover reconstructions from HYDE11 for the low and the intermediate scenarios and the land cover estimates from KK11 for the high emission scenario. With this approach, the pasture area per person lies in a range between 0.58 and 1.0 ha for the different emission scenarios, which is similar to the values (0.56 and 1.05 ha, respectively) mentioned in Klein Goldewijk et al. (2011, 2017) but somewhat lower than the number derived in a case study for Greece in the Roman period (1.75 ha; Weiberg et al., 2019). We considered low, intermediate, and high estimates for Frpasture_burnt and the emission factors because these variables have large uncertainties.
Table 3The values used to calculate the aerosol emissions from pasture burning (Eq. 6) for the low, intermediate, and high scenarios. Note that the total area of pasture in the study domain (Areapasture) is shown instead of the fraction per grid box (Frpasture).
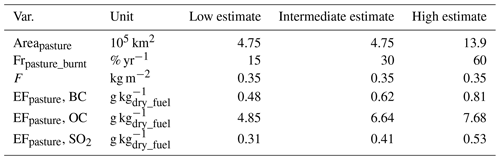
2.7.1 Fuel biomass consumption F
The increase in European grassland productivity over the last decades has been small compared to crop (Smit et al., 2008). The spatial variability of grassland productivity is quite large within Europe, ranging from ≈ 0.15 kg m−2 yr−1 in the Mediterranean region up to 0.65 kg m−2 yr−1 in the Atlantic zones; the median over the different climate zones of Europe is 0.33 kg m−2 yr−1 (Smit et al., 2008). As a consequence, we expect the fuel load and fuel biomass consumption to also show spatial variability. In contrast to fuel biomass consumption, fuel load refers to fuel that is available (but not necessarily burnt) and often refers to aboveground biomass only. Nevertheless, values for fuel biomass consumption and fuel load are quite comparable because grass easily burns and mainly aboveground biomass is consumed during pasture burning.
Values of fuel loads (kgdry_matter per area) frequently lie in the range between 0.3 and 0.5 kg m−2 for pastures and grasslands: for Kentucky bluegrass, 0.26, 0.36, and 0.64 kg m−2 were measured in Idaho (Holder et al., 2017); values for grasslands in Australia are around 0.32 and 0.46 kg m−2 (Cruz et al., 2016, 2017); in South Africa, total aboveground fuel loads in savanna parklands range from 0.22 to 0.55 kg m−2 (except for a site subjected to 38 years of fire exclusion; Shea et al., 1996), whereas the fuel loads of standing herbaceous material range from 0.30 to 0.41 kg m−2 (Smith et al., 2013); a total fuel load of 0.49 kg m−2 for Mediterranean grasslands in Greece was measured (Dimitrakopoulos, 2002).
Fuel biomass consumption for savannas is comparable: 0.26 kg m−2 for savanna woodlands for early dry season burns, 0.46 kg m−2 for savanna woodlands for middle to late dry season burns, 0.21 kg m−2 for savanna grasslands for early dry season burns, and 1.0 kg m−2 for savanna grasslands for middle to late dry season burns (0.54 kg m−2 when excluding one high value for tropical pasture; Aalde et al., 2006).
Based on these studies, we estimate that a reasonable average number for fuel biomass consumption is F=0.35 kgdry_matter m−2.
2.7.2 Fraction of pasture burnt Frpasture_burnt
In the Mediterranean region, pasture burning has continued throughout history to the present day and is one component that has shaped the diversity of Mediterranean landscapes as we know them (Montiel and Kraus, 2010). In temperate and boreal Europe, the burning of grasslands for pasture was common on lands which were too poor in nutrients for agriculture, e.g. heathlands (Montiel and Kraus, 2010).
In general, the abundance of prescribed burning depends on the accumulation of biomass: the higher the accumulation, the shorter the fire interval. As a consequence, the fire interval depends on rainfall and grazing pressure (Weir et al., 2013; Frost and Robertson, 1987), thus showing pronounced regional variability. In the following we summarise guidelines for the rate of prescribed burning from different regions around the world.
For phryganic rangelands in Greece, it is recommended to set fire every 3 to 4 years, which allows for good herbage production and at the same time a suppression of undesirable dwarf shrub (Papanastasis, 1980). In South Africa, Oluwole et al. (2008) found that the recovery period should be 3 years for optimum productivity in the absence of grazing. In line with this, the Burning Guidelines of South Africa do not recommend burning pasture every year (which some farmers do) but every 2–5 years in mesic and coastal grasslands and only when it is needed in dry Highveld grasslands (SANBI, 2014). Smith et al. (2013) found that grass richness, evenness, and diversity were high for sites with high rainfall when frequent burning was applied in the dry season (1- to 3-year return intervals), whereas Little et al. (2015) conclude that annual burning combined with intensive grazing has a detrimental effect on plant species diversity and structure. In Australia, single fires caused a short-term reduction of yield and cover of pastures in the following year, but fast recovery occurred for most burning regimes. However, perennial grasses were reduced at the expense of annual grasses, which is why burning every 5–6 and 4–6 years for arid short grass and ribbon bluegrass, respectively, is recommended (Dyer, 2011). This is in agreement with the findings of Norman (1963, 1969) for native pasture on Tippera clay loam in the Katherine region. For North America, the recommended fire-return interval of prescribed patch burning is 3 years in areas with rainfall above ≈760 mm yr−1 and 4 years in drier regions (Weir et al., 2013).
One could argue that farmers in the past did not necessarily follow these present-day guidelines. However, traditional knowledge of prescribed burning has been lost in many European areas (Montiel and Kraus, 2010). Guidelines thus partially re-establish knowledge that our ancestors had. On the one hand, if burning every year reduces the productivity of many grasslands, we think that it is unlikely that ancient farmers burnt their fields annually. On the other hand, a period without burning that is too long is also unlikely since this e.g. allows for the growth of unwanted species and can have adverse effects on the ecosystem (SANBI, 2014; Papanastasis, 1980). According to the summarised literature, burning every ≈3 years seems to be a reasonable intermediate estimate. Therefore, we assumed that 30 % of the pasture area is burnt per year for the intermediate scenario. For the low and high emission scenarios, we changed this fraction by a factor of 2 and thus arrive at 15 % and 60 %, respectively. We assumed that the aerosols from pasture burning were emitted throughout the year, but – like for crop residue – that no pasture was burnt in months which are very cold or hot (monthly average temperatures below 0 or above 30 ∘C).
2.7.3 Emission factor EFpasture
Using the method described in Sect. 2.5.3 and the measurements from Table S17 (key 4), the emission factors in Table 3 were derived. We again used the lower quartiles for the low, the medians for the intermediate, and the upper quartiles for the high emission scenarios.
2.8 Simulations
We conducted six time-slice experiments representative for the period around 100 CE (Table 4): no_human, LCC_HYDE, LCC_KK, LCC_HYDE_low, LCC_HYDE_int, and LCC_KK_high. The simulation no_human does not account for anthropogenic impacts (except for potential influences on greenhouse gas concentrations at this time). LCC_HYDE and LCC_KK consider only the effects of anthropogenic land cover change (Sect. 2.3) on climate. The simulations LCC_HYDE_low, LCC_HYDE_int, and LCC_KK_high consider, in addition to anthropogenic land cover change, anthropogenic aerosol emissions due to fuel consumption, crop residue burning, and pasture burning. While the anthropogenic land cover reconstructions provide global values and are thus applied on the global scale, we only calculated anthropogenic aerosol emissions for the Roman Empire and not for other parts of the world; i.e. no anthropogenic aerosol emissions occur outside our study domain.
The simulations have a spatial resolution of approximately and 47 vertical levels (T63L47). After the first 3 months of spin-up, natural vegetation was replaced by the area of anthropogenic land cover for 100 CE within one model year; after that the vegetation fractions were kept constant. A 1-year model spin-up was added after the transition to anthropogenic land cover was completed, resulting in a total spin-up of 2 years and 3 months. The spin-up is relatively short since we use neither an interactive ocean nor dynamic vegetation. The simulations (excluding spin-up) are 20 years long and should be representative for approximately 100 CE, i.e. the time of interest.
In the standard ECHAM-HAM-SALSA version, the cloud droplet number concentration in a cloud cannot be lower than 40 cm−3 (or optionally 10 cm−3; Tegen et al., 2019). For all simulations of this study, this minimum CDNC was lowered from 40 to 1 cm−3 (and the model was retuned) because the aerosol concentrations – and therefore most likely CDNCs – were considerably lower around 100 CE than today.
Table 4Overview of the different simulations. Note that the anthropogenic land cover change is applied in ECHAM-HAM-SALSA but not in CBALONE–SPITFIRE, which was used to calculate aerosol emissions from natural fires exclusively.
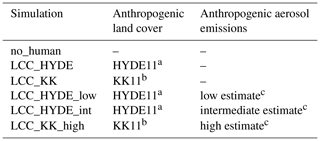
a Klein Goldewijk et al. (2010, 2011). b Kaplan et al. (2011, 2012). c This study; Sect. 2.5, 2.6, 2.7.
3.1 Climate impact of anthropogenic land cover change
The simulations LCC_HYDE and LCC_KK were compared with the simulation no_human to quantify the impact of anthropogenic land cover change. Tables S8 and S9 show seasonal and annual mean changes in some climate variables, including all that are discussed below.
The replacement of natural vegetation by crop and pasture leads to a decrease in SOA precursors, which is most likely responsible for the significant3 changes in CDNC, liquid water path (LWP), and CRE for LCC_KK (Table S9; all cloud properties are grid means).
In our simulations, the surface albedo can either increase or decrease significantly depending on the season and the region. Deforestation can introduce brighter vegetation types and thus an increase in surface albedo (e.g. in Spain; Fig. 2a,b). However, especially after harvest, crop has a smaller canopy area fraction than natural vegetation. Thus, more of the soil, which is in some regions darker than the natural vegetation, is exposed to radiation. As a consequence, the annually averaged surface albedo decreases in parts of Europe (Fig. 2a,b) because the surface albedo there increases in summer but decreases in other seasons (not shown).
Land cover change also alters latent heat (LH) and sensible heat (SH) fluxes. The annual mean evaporative fraction (Evap_frac; only calculated if both SH and LH are upward fluxes, otherwise set to zero) shows no significant changes regionally (Fig. 2c,d). In contrast, regional changes in the annual mean turbulent flux (; again only calculated if both SH and LH are upward fluxes) are significant for KK11 (Fig. 2f).
For HYDE11, the land surface temperature shows hardly any significant changes (Fig. 2g). For KK11, our results suggest that the changes in turbulent flux have a larger influence on the land surface temperature than the changes in surface albedo and evaporative fraction (Fig. 2). The decrease in turbulent flux regionally leads to a less efficient heat transport from the surface to the atmosphere, thus enhancing the land surface temperature (Fig. 2h). In contrast, the land use in Smith et al. (2016) induces a distinct cooling over Europe around 1 CE. We think that the opposite signal is mainly caused by differences in the vegetation or atmospheric models; intercomparison studies have shown that not all models agree on the sign of annual mean temperature changes induced by deforestation over North America and in mid-latitudes (Lejeune et al., 2017; Winckler et al., 2019). In our simulations, significant warming occurs south of 40∘ N, which is in qualitative agreement with present-day satellite studies (Li et al., 2015; Y. Li et al., 2016b). These studies indicate that the impact of deforestation shifts from a warming in the tropics to a cooling in boreal regions, though with high uncertainty in the exact latitude of transition (Li et al., 2015; Y. Li et al., 2016b).
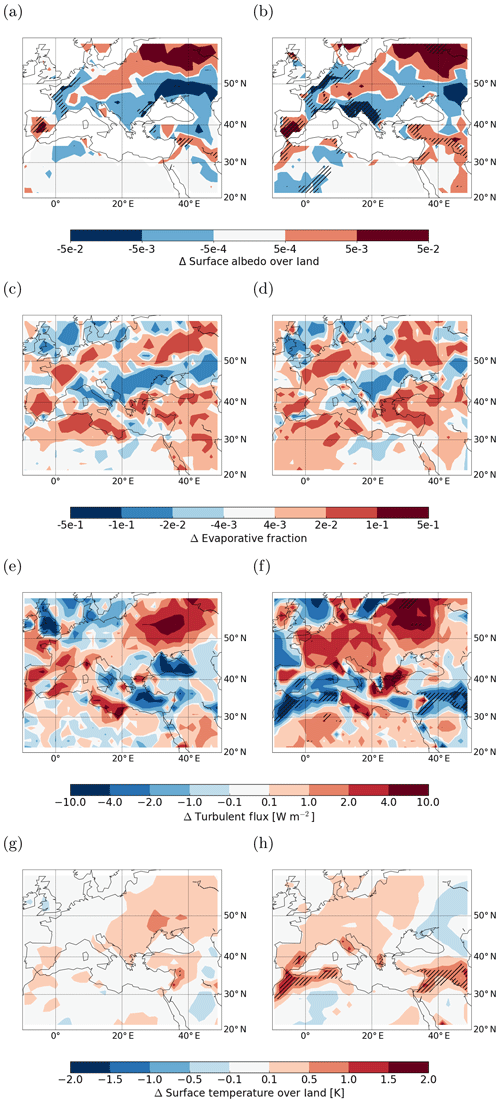
Figure 2The impact of anthropogenic land cover change using HYDE11 (a, c, e, g) and KK11 (b, d, f, h). Shown are changes in surface albedo over land (a, b), evaporative fraction (c, d), turbulent flux (e, f), and land surface temperature (g, h). Statistically significant changes (5 % significance level; N=20) are hatched. Note that the SSTs in the simulations are fixed.
3.2 Anthropogenic aerosol emissions around 100 CE and their magnitude compared to natural fire emissions
For the majority of scenarios and aerosol (precursor) species, the emissions from pasture burning contribute the most to total anthropogenic emissions (Fig. 3). The emissions from fuel consumption are smaller in most cases but of the same order of magnitude (Fig. 3). Averaged over the whole year, the emissions from crop residue burning are relatively small; however, in summer, the emissions are in some cases comparable to pasture burning and/or fuel consumption (e.g. Fig. 3b).
In the following, we compare the anthropogenic aerosol emissions to the natural fire emissions in 100 CE; if the anthropogenic aerosol emissions are very low compared to the natural fire emissions, we would not expect any influence of the anthropogenic emissions. On the other hand, if the anthropogenic aerosol emissions are of the same order of magnitude as the natural fire emissions or higher, then they could have an impact on radiation and clouds, depending on the amount of natural aerosols from other sources (i.e. mineral dust, sea salt, organic aerosol emissions from biogenic sources, and sulfate from volcanic, oceanic, and terrestrial sources).
Next to the total anthropogenic aerosol emissions, Figs. 4, S2, and S3 (for BC, OC, and SO2, respectively) also show the natural fire emissions used in the simulations without anthropogenic aerosols (no_human, LCC_HYDE, LCC_KK; called “ref” in the figure) and the natural fire emissions for the respective scenarios in which the natural fire emissions are set to 0 in areas where crop or pasture grow (LCC_HYDE_low, LCC_HYDE_int, LCC_KK_high; called LCC in the figure). By comparing ref to the sum of LCC and the anthropogenic emissions, the potential impact of the anthropogenic emissions can be estimated.
The anthropogenic emissions have a much less pronounced annual cycle than the natural emissions, the latter showing considerably higher emissions in summer than in winter. Averaged over the whole year, the anthropogenic emissions of the low (Fig. 4b) and intermediate (Fig. 4c) scenarios are small compared to natural fire emissions (ref), while the anthropogenic emissions of the high scenario are similar. However, the comparison strongly depends on the season. For the low emission scenario, the total aerosol emissions from natural fires (LCC) plus anthropogenic activities are obviously smaller than the natural fire emissions (ref) in summer; i.e. humans overall reduce aerosol emissions. In contrast, the anthropogenic emissions are clearly higher than the natural fire emissions in winter, as illustrated in Fig. 4a, which shows the months January to March on a different scale (the scale is 2 orders of magnitude lower). For the intermediate emission scenario, the anthropogenic emissions are larger than the natural fire emissions (ref) for approximately half of the year. The anthropogenic emissions for the high emission scenario are higher than the natural fire emissions for most of the year (Fig. 4d).
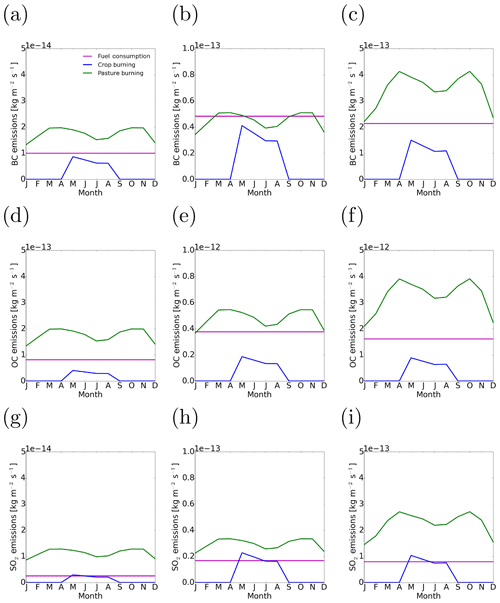
Figure 3Monthly mean anthropogenic aerosol emissions due to fuel consumption, crop residue burning, and pasture burning in the study domain over the year. (a)–(c) BC; (d)–(f) OC; (g)–(i) SO2 emissions. (a, d, g) Low emission scenario; (b, e, h) intermediate emission scenario; (c, f, i) high emission scenario. Note the different scales on the y axis.
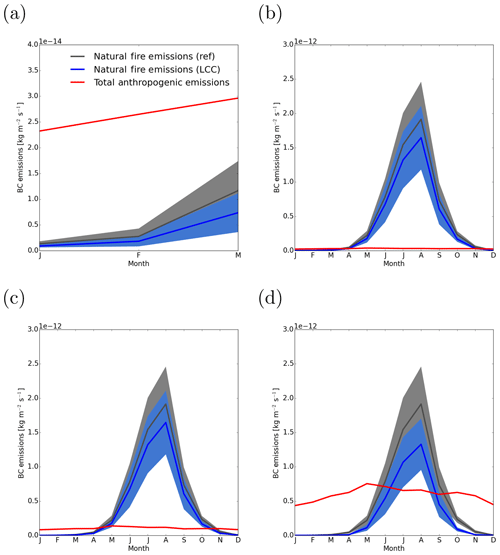
Figure 4Monthly averaged BC emissions in our study domain for (a) the beginning of the year and (b)–(d) the whole year. The shadings show the standard deviation for each month over the 20-year period since our natural fire emissions have interannual variability. The grey line shows the natural fire emissions of the simulations no_human, LCC_HYDE, and LCC_KK, whereas the blue line shows the natural fire emissions of the simulations LCC_HYDE_low, LCC_HYDE_int, and LCC_KK_high, in which anthropogenic land cover change reduces the natural fire emissions. The red line shows the total anthropogenic aerosol emissions. In (a) and (b), the BC emissions from the low scenario are shown; note that the y scale is smaller in (a) than in the other subfigures. In (c) and (d), the emissions from the intermediate and high scenarios are shown, respectively.
3.3 Comparison of anthropogenic aerosol emissions around 100 CE and 1850 CE
To put our calculated anthropogenic aerosol emissions for 100 CE into some temporal context, we compare them to the anthropogenic aerosol emissions in 1850 CE based on the ACCMIP (Atmospheric Chemistry and Climate Model Intercomparison Project) inventory (Lamarque et al., 2010). The emissions are in both cases averaged over our study domain and the year. Anthropogenic ACCMIP emissions in 1850 CE include the sectors industry, land transport, maritime transport, residential and commercial combustion, agricultural waste burning on fields, and waste. Not all of these sectors produced aerosol emissions in 100 CE; our emissions thus only include fuel consumption (due to both industrial and residential combustion), agricultural waste burning on fields, and pasture burning. Emissions from pasture burning are not an ACCMIP sector; we expect that the anthropogenic ACCMIP emissions in 1850 CE could be somewhat higher if pasture burning were included.
The BC emissions from the high scenario in 100 CE are nearly as high as the emissions in 1850 CE, whereas the emissions from the low and intermediate scenarios are considerably lower (Fig. 5a). For OC, the emissions for the low and intermediate scenarios are lower than the emissions in 1850 CE, whereas the high scenario results in approximately twice as high aerosol emissions as in 1850 CE (Fig. 5b). The large OC emissions in 100 CE could be due to differences in emission factors: uncertainties in emission factors from biomass burning are large, and the composition of fuels was different in 1850 CE than in 100 CE (e.g. more coal in 1850 CE). For SO2, the emissions in 100 CE are for all scenarios clearly lower than those in 1850 CE (Fig. 5a), which might again be related to the larger contribution of fossil fuels in 1850 CE.
Comparing the aerosol emissions per capita gives similar results (Fig. 6): relative to 1850 CE, emissions are highest in 100 CE for OC, followed by BC and then SO2. For OC, the per capita emissions are higher in 100 CE than in 1850 CE for the high and intermediate scenarios. For BC, the per capita emissions are only higher for the high emission scenario, while the per capita emissions for SO2 are lower than in 1850 CE for all scenarios.
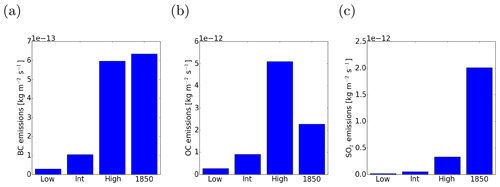
Figure 5The annual mean anthropogenic aerosol emissions of (a) BC, (b) OC, and (c) SO2 in 100 CE averaged over the study domain (10∘ W to 50∘ E, 20 to 60∘ N) for the low emission scenario (low), the intermediate emission scenario (int), and the high emission scenario (high). Also shown are the anthropogenic aerosol emissions from the ACCMIP inventory for the year 1850 CE averaged over the same region.
3.4 Impact of anthropogenic aerosol emissions around 100 CE on radiation and PM2.5 concentrations
In this section, the impact of anthropogenic aerosol emissions around 100 CE is assessed by comparing simulations considering anthropogenic land cover change and aerosol emissions with simulations that only consider anthropogenic land cover change (i.e. LCC_HYDE_low, LCC_HYDE_int compared with LCC_HYDE; LCC_KK_high compared with LCC_KK). The changes in some cloud properties and radiative effects, averaged over the study domain and the whole year, are shown in Table 5. Tables S10, S11, and S12 include more variables and also show seasonal averages for the low, intermediate, and high emission scenarios, respectively.
The BC and OC burdens (vertically integrated mass) change significantly for all emission scenarios (Tables S10 to S12), with stronger changes for BC, whereas the SO4 burden does not change significantly. This is due to the different emission sectors of the three aerosol types: while fire emissions are the only natural source of BC particles, OM also has biogenic sources, and SO4 predominantly forms from the gas-to-particle conversion of volcanic and oceanic precursor emissions. The changes in aerosol burdens show large seasonal differences: in summer, the BC and OC burdens decrease for the low and intermediate emission scenarios because of the reduction in natural fires (Sects. 2.4, 3.2). In winter, the BC and OC burdens increase pronouncedly for all emission scenarios due to anthropogenic aerosol emissions.
Averaged over the study domain and the year, ARE changes by 0.06, 0.12, and 0.12 W m−2 for the low, intermediate, and high emission scenarios, respectively (Table 5). The largest increases occur over North Africa and Europe (Fig. 7a–c). In contrast to ARE, the changes in CRE are negative and considerably more pronounced: −2.11, −4.17, and −7.63 W m−2 for the three scenarios, respectively. The changes predominantly occur over Europe (Fig. 7d–f). The cooling effect of clouds is enhanced because they become optically thicker and more abundant due to aerosol-induced increases in CDNC and LWP (Tables S10 to S12). Overall, the negative changes in CRE are larger than the positive changes in ARE. As a consequence, the anthropogenic aerosol emissions induce a decrease in land surface temperature (Fig. 7g–i).
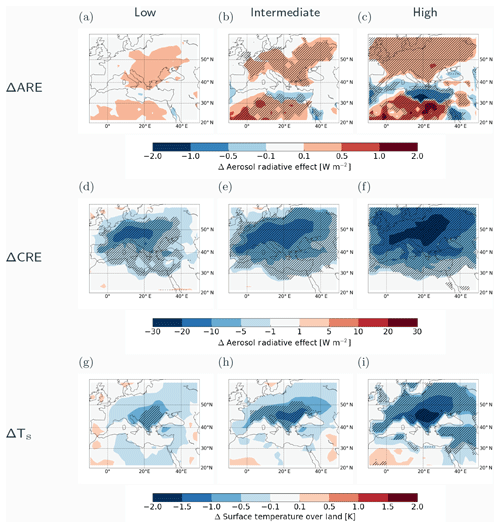
Figure 7The impact of anthropogenic aerosol emissions for the low (a, d, g), intermediate (b, e, h), and high emission scenarios (c, f, i). Shown are differences in aerosol radiative effect (a–c), cloud radiative effect (d–f), and land surface temperature (g–i). Statistically significant changes (5 % significance level; N=20) are hatched. Note that the SSTs in the simulations are fixed.
Table 5Annual mean impact of anthropogenic aerosol emissions on different variables averaged over the study domain (10∘ W to 50∘ E and 20 to 60∘ N): cloud droplet number concentration (CDNC), liquid water path (LWP), cloud cover (CC), aerosol radiative effect (ARE), and cloud radiative effect (CRE) for all simulations except no_human. Significant (5 % significance level; N=20) changes compared to the respective reference are marked with an asterisk (*).

1 Changes are relative to HYDE11. 2 Changes are relative to KK11.
Aerosol particles are also relevant because of their impact on human health. According to Makra (2015), air pollution had substantial consequences in cities in ancient times. As an example, the philosopher Seneca wrote that he needed to leave Rome in order to escape the smoke and the kitchen smells and to feel better (Makra, 2015). As maintained by the World Health Organization WHO (2006), the annual mean PM2.5 concentration should not exceed 10 µg m−3. Figure 8 shows the background surface PM2.5 concentrations and the increases due to anthropogenic emissions. For the low emission scenario, changes in the PM2.5 concentration are below 1 µg m−3 everywhere. For the intermediate emission scenario, significant increases mainly range between 0.1 and 2 µg m−3, which is for most places insufficient to increase the background concentrations to values above 10 µg m−3. Only for the high emission scenario is the increase in PM2.5 concentration pronounced enough to exceed the WHO threshold in larger areas (e.g. Algeria, Egypt). The maximum PM2.5 concentrations are to some degree underestimated due to our coarse model resolution. As an example, Y. Li et al. (2016a) found that the maximum simulated PM2.5 concentrations decrease by −21 % if the resolution decreases from to .
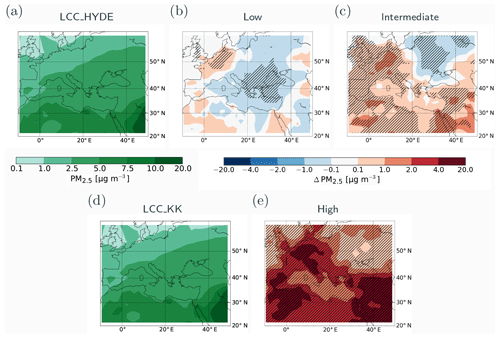
Figure 8The background surface PM2.5 concentrations (a LCC_HYDE; d LCC_KK) and the changes in PM2.5 surface concentrations due to anthropogenic aerosol emissions for the low (b LCC_HYDE_low), intermediate (c LCC_HYDE_int), and high (e LCC_KK_high) emission scenarios. Statistically significant changes (5 % significance level; N=20) are hatched.
Because ECHAM-HAM-SALSA is an atmosphere-only model, SSTs and SIC were prescribed. Therefore, temperature does not react to a perturbation as much as with an interactive ocean, implying that, in the absence of attenuating feedbacks, our simulated changes in temperature should be underestimated. We repeated two of the simulations (no_human, LCC_HYDE_int) with a mixed-layer ocean (MLO) model having a depth of 50 m (≈50 years of simulation, half of it model spin-up) and indeed arrived at larger changes in land surface temperature (a decrease in land surface temperature averaged over our study domain that is 3.5 times stronger). Nevertheless, the temperature patterns occurring with fixed SSTs remain visible with the MLO setup: the land surface temperature decreases mainly over Europe due to anthropogenic aerosol emissions (Fig. S4). As expected, the aerosol-induced cooling is more widespread with the MLO, but it is basically limited to the Roman Empire. Since the changes in variables affecting temperature (e.g. CRE) are comparable for the different model setups, qualitatively similar though stronger changes in the land surface temperature occur with the MLO. Nevertheless, we admit that a more detailed analysis using the MLO, including all scenarios, will provide more quantitative and perhaps partly different results. However, this is beyond the scope of this study.
The two land cover reconstructions that we chose differ widely and thus provide a measure for uncertainty. However, the soil albedo and vegetation cover of the simulation no_human, which have a strong influence on the climate response, are also subject to uncertainty. We found that the contribution of grasslands to the natural vegetation is quite high in our simulations. As an example, grasslands and tundra contribute ≈45 % to the natural vegetation between 0 and 30∘ E and between 30 and 60∘ N. If the forest fraction were underestimated in our simulation, the impact of the anthropogenic land cover change would likely also be underestimated. This would especially be the case for creating pasture, since grasslands are preferentially converted to pasture in the model (Reick et al., 2013). Furthermore, the crop phenology of JSBACH, which considers both winter and summer crop, allows two harvests of summer crops during 1 year in the extratropics depending on the heat sum. Generally, the productivity of crops was smaller in the Roman Empire than today, with fallow every 2 to 3 years. The number of harvests is thus likely overestimated in JSBACH (but not in the offline-calculated crop residue burning emissions), which affects, for example, changes in surface albedo (which could be more positive in reality).
The anthropogenic aerosol emissions that we calculated are only rough estimates. To calculate aerosol emission factors for fuel consumption, we used measurements from present-day fireplaces and traditional stoves. The measurements show a large variability, which is caused e.g. by the fuel moisture, burning device, fire conditions (flaming versus smoldering), or instrumental setup. To account for the large range of observed emission factors, we considered many measurements. Nevertheless, we cannot rule out the possibility that typical stoves used in the Roman Empire had systematically different emission factors than the majority of burning devices that we considered. Furthermore, the uncertainties of important parameters such as population size are considerable when going so far back in time. The emissions are thus highly uncertain, and it is extremely challenging to quantitatively verify them with palaeo-records. At least there is indirect evidence for aerosol emissions associated with fuel consumption and agricultural burning: marble turned grey in antique towns due to smoke and laws that were introduced against air pollution (Makra, 2015). Moreover, pollen and charcoal records show high positive correlations between fire and crops, weeds, and shrubs in Mediterranean and temperate regions in and around the Alps between 2300 BCE and 800 CE (Tinner et al., 2005, 2009). Palaeo-records further suggest that controlled burning was used to introduce and establish sweet chestnut in some regions (Morales-Molino et al., 2015).
Another simplification is our lack of spatial and temporal variations: for many variables, we estimated one “typical” value for the whole Roman Empire, and our anthropogenic emissions show no trends over the years (e.g. caused by wars). The time around 100 CE was a relatively stable period characterised by the expansion of infrastructure, economic wealth, and quite a low level of military activities. Nevertheless, the emissions were more dynamic in reality than in our simulations, e.g. due to Trajan's Dacian Wars (101–102 CE and 105–106 CE), which caused population movements and a possible change in anthropogenic aerosol emissions (e.g. regional emissions associated with warlike activities such as burning of villages).
The impact of our simulated anthropogenic aerosol emissions strongly depends on the natural background and its seasonality. In our simulations, oceanic DMS concentrations, dust potential sources, and volcanic tropospheric SO2 emissions are representative for present-day conditions, which could have an impact on the background aerosol concentrations. Moreover, fire models show large differences, and thus it is unclear how realistic the fire emissions and the strong seasonal cycle of our CBALONE–SPITFIRE simulations (Sect. 3.2) are. The fire emissions from our simulations are 1 order of magnitude higher than the emissions by van Marle et al. (2017) in the study domain (Sect. S9), indicating that our natural fire emissions could be overestimated. On the one hand, this could indicate that our background aerosol concentration is potentially overestimated as well. On the other hand, our model neglects pure biogenic aerosol nucleation and nitrate aerosols. Using the GLOMAP model, Gordon et al. (2016) have shown that the global annual mean CCN concentration (at 0.2 % supersaturation and at cloud-base level) was 12 % higher in the pre-industrial atmosphere when pure biogenic nucleation is considered. The effect is not the same over the year since terpene emissions are larger in summer (Gordon et al., 2016). Therefore, our simulated background concentration when we see the highest impact of anthropogenic aerosols (winter, spring) might not be affected too much by the neglect of biogenic nucleation.
The aerosol background depends not only on emissions, but also on other parameters such as the simulated size distribution and the calculation of removal processes. A simplification of our study is that most of the tropospheric chemistry is not calculated, and hence we prescribe the oxidants that are important for sulfur chemistry and SOA formation. The vertical distribution of the aerosol particles is also essential when their effect on radiation and clouds is analysed. In ECHAM-HAM, vertical mixing is strong in the lower troposphere (Veira et al., 2015), and thus the anthropogenic aerosol particles emitted near the surface can easily reach altitudes at which clouds form. If this vertical mixing were overestimated in the model, then the aerosol–cloud interactions would likely be overestimated as well.
Last but not least, aerosol–radiation and aerosol–cloud interactions from aerosol–climate models are uncertain. As an example, some studies show that the models typically overestimate the effect of aerosols on the cloud liquid water content, at least in some regions (Bender et al., 2019). The aerosol-effective radiative forcing also depends on the minimum CDNC value (Hoose et al., 2009); since we lowered the minimum CDNC to 1 cm−3, the aerosols have a stronger impact on radiation than with the standard setup of ECHAM-HAM-SALSA wherein the minimum CDNC is 40 cm−3. We conducted additional simulations showing that the total ERF due to anthropogenic aerosols between 1850 CE and 2000 CE is W m−2 with our decreased minimum CDNC value. Our ERFari+aci thus lies at the lower end of model estimates and is outside the range of Intergovernmental Panel on Climate Change (IPCC) expert judgment (−1.9 to −0.1 W m−2; Boucher et al., 2013). Nevertheless, we expect that the anthropogenic aerosol emissions around 100 CE would still increase the CDNC in the Roman Empire (and thus induce a cooling effect) with the standard minimum CDNC value of 40 cm−3: the simulated CDNCs are above 40 cm−3 in the lower troposphere for all seasons when averaged over our study domain (not shown). The strong total ERFari+aci with a minimum CDNC of 1 cm−3 therefore mainly results from other regions, e.g. the Arctic and the Antarctic.
The hypothesis of this study was that anthropogenic activities associated with land cover and aerosols already had a noticeable influence on some climate variables in the Roman Empire around 100 CE. To test this hypothesis, we first created three different scenarios of anthropogenic aerosol emissions for the Roman Empire. These scenarios were combined with two existing land use datasets.
Simulations with an aerosol-enabled global climate model showed that the anthropogenic land cover reconstruction by KK11 (Kaplan et al., 2011, 2012) induces significant decreases in turbulent flux and thus a warming in parts of North Africa and the Middle East, whereas the reconstruction based on HYDE11 (Klein Goldewijk et al., 2010, 2011) has nearly no impact. Mainly over central and eastern Europe, anthropogenic aerosol emissions lead to an enhanced cooling effect of clouds (i.e. a more negative cloud radiative effect), the extent and strength of which depends on the different scenarios. Our model likely overestimates changes in the cloud radiative effect to some degree due to the lowered minimum CDNC (Hoose et al., 2009). Overall, land use thus generally increases the land surface temperature in our simulations, whereas anthropogenic aerosol emissions decrease it. Since we prescribe the SSTs, our simulated changes in temperature are strongly underestimated. Simulations with an interactive ocean are therefore needed for a more quantitative analysis.
Around 100 CE temperatures in Europe (as well as China and the Northern Hemisphere in general) were warmer than the average over the last 2 millennia (PAGES 2k Consortium, 2013; Ge et al., 2013; Christiansen and Ljungqvist, 2012), an era that has been called the “Roman Warm Period”. While our results imply that anthropogenic land cover change may have regionally contributed to this warming, aerosol–cloud interactions would have attenuated it, suggesting other causes of the Roman Warm Period, e.g. ocean dynamics or solar forcing.
Our scenarios show that pasture burning could have been an important source of aerosol particles. A better understanding of the processes that drive the frequency, seasonality, and emissions of pasture burning could therefore be essential to quantify the anthropogenic impact far back in time. These processes could be implemented in a vegetation–fire model that is coupled with an aerosol model. Simulations with a higher spatial resolution would moreover allow models to account for the large regional variations in aerosol emissions within the Roman Empire – information which can be provided by archaeologists and historians. Therefore, further work should include collaborations with them in order to incorporate a better understanding of human behaviour in classical antiquity, particularly in terms of fuel consumption and the timing and extent of the use of fire. Such collaborations could also allow researchers to assess the effect of economic crises (e.g. in the third century CE) and wars on aerosol emissions.
The data can be found at https://data.iac.ethz.ch/Gilgen_et_al_2019_RomanEmpire/ (last access: 15 October 2019; Gilgen et al., 2019).
The supplement related to this article is available online at: https://doi.org/10.5194/cp-15-1885-2019-supplement.
AG had the initial idea for the study, calculated the anthropogenic aerosol emissions, adapted the anthropogenic land cover data, conducted the simulations with ECHAM-HAM-SALSA, analysed the results, created the figures, and wrote the main part of the paper. SW did the major work for calculating the CBALONE–SPITFIRE emissions. JOK developed the KK11 land use reconstruction and contributed to the development of the anthropogenic emission scenarios. TK implemented the VBS in ECHAM-HAM-SALSA that was used in this study. UL was responsible for supervising AG. All authors were involved in discussions about the setup of the simulations and the study; all authors participated in the analysis and the writing.
The authors declare that they have no conflict of interest.
We are deeply grateful to Thomas Raddatz, who provided temporally high-resolution data from the MPI-ESM to drive CBALONE–SPITFIRE. He also helped the main author with questions about JSBACH and its anthropogenic land cover scheme. We thank Robyn Veal, Edouard Davin, Luisa Ickes, Silvia Kloster, Tanja Stanelle, and David Neubauer for valuable inputs and discussions. Furthermore, we thank Jürgen Bader for providing SSTs and SIC from at that time unpublished MPI-ESM simulations and Silvia Kloster and Christian Reick for enabling collaborations between people from the MPI Hamburg and ETH Zürich. We generally thank the developers of the ECHAM-HAMMOZ model, which is developed by a consortium composed of ETH Zürich, Max Planck Institut für Meteorologie, Forschungszentrum Jülich, the University of Oxford, the Finnish Meteorological Institute, and the Leibniz Institute for Tropospheric Research; it is managed by the Center for Climate Systems Modeling (C2SM) at ETH Zürich. We also acknowledge all the people that made their published data available, e.g. the developers of CESM2.0 WACCM and the people contributing to CMIP6 input data and to the HYDE database. Last but not least, we cordially thank the two anonymous reviewers for their well-conceived comments and helpful suggestions.
This research has been supported by the Swiss National Science Foundation (grant no. CRSII2_154450) and by a grant from the Swiss National Supercomputing Centre (CSCS; project ID s652).
This paper was edited by Carlo Barbante and reviewed by two anonymous referees.
Aalde, H., Gonzalez, P., Gytarsky, M., Krug, T., Kurz W, A., Lasco R, D., Martino D, L., McConkey B, G., Ogle S, M., Paustian, K., Raison, J., Ravindranath N, H., Schoene, D., Smith, P., Somogyi, Z., van, A. A., and Verchot, L.: Generic methodologies applicable to multiple land-use categories, 2006 IPCC Guidelines for National Greenhouse Gas Inventories”, Volume 4: Agriculture, Forestry and Other Land Use; available at: https://www.ipcc-nggip.iges.or.jp/public/2006gl/pdf/4_Volume4/V4_02_Ch2_Generic.pdf, 2006. a, b
Albrecht, B.: Aerosols, Cloud Microphysics, and Fractional Cloudiness, Science, 245, 1227–1230, https://doi.org/10.1126/science.245.4923.1227, 1989. a
Andres, R. J. and Kasgnoc, A. D.: A time-averaged inventory of subaerial volcanic sulfur emissions, J. Geophys. Res.-Atmos., 103, 25251–25261, https://doi.org/10.1029/98JD02091, 1998. a
Anklin, M. and Bales, R. C.: Recent increase in H2O2 concentration at Summit, Greenland, J. Geophys. Res.-Atmos., 102, 19099–19104, https://doi.org/10.1029/97JD01485, 1997. a
Arora, V. K. and Melton, J. R.: Reduction in global area burned and wildfire emissions since 1930s enhances carbon uptake by land, Nat. Commun., 9, 1326–1326, 2018. a
Ascoli, D. and Bovio, G.: Prescribed burning in Italy: issues, advances and challenges, iForest, 6, 79–89, https://doi.org/10.3832/ifor0803-006, 2013. a
Bader, J., Jungclaus, J., Krivova, N., Lorenz, S., Maycock, A., Raddatz, T., Schmidt, H., Toohey, M., Wu, C.-J., and Claussen, M.: Global temperature modes shed light on the Holocene temperature conundrum, Nat. Commun., in review, 2019. a, b
Bathiany, S., Claussen, M., Brovkin, V., Raddatz, T., and Gayler, V.: Combined biogeophysical and biogeochemical effects of large-scale forest cover changes in the MPI earth system model, Biogeosciences, 7, 1383–1399, https://doi.org/10.5194/bg-7-1383-2010, 2010. a
Bender, F. A.-M., Frey, L., McCoy, D. T., Grosvenor, D. P., and Mohrmann, J. K.: Assessment of aerosol–cloud–radiation correlations in satellite observations, climate models and reanalysis, Clim. Dynam., 7–8, 4371–4392, https://doi.org/10.1007/s00382-018-4384-z, 2019. a
Bergman, T., Kerminen, V.-M., Korhonen, H., Lehtinen, K. J., Makkonen, R., Arola, A., Mielonen, T., Romakkaniemi, S., Kulmala, M., and Kokkola, H.: Evaluation of the sectional aerosol microphysics module SALSA implementation in ECHAM5-HAM aerosol-climate model, Geosci. Model Dev., 5, 845–868, https://doi.org/10.5194/gmd-5-845-2012, 2012. a
Bielenstein, H.: Wang Mang, the restoration of the Han dynasty, and Later Han, vol. 1, The Cambridge History of China, 223–290, Cambridge University Press, https://doi.org/10.1017/CHOL9780521243278.005, 1986. a
Bond, T. C., Streets, D. G., Yarber, K. F., Nelson, S. M., Woo, J.-H., and Klimont, Z.: A technology-based global inventory of black and organic carbon emissions from combustion, J. Geophys. Res.-Atmos., 109, D14203, https://doi.org/10.1029/2003JD003697, 2004. a, b
Boucher, O., Randall, D., Artaxo, P., Bretherton, C., Feingold, G., Forster, P., Kerminen, V.-M., Kondo, Y., Liao, H., Lohmann, U., Rasch, P., Satheesh, S., Sherwood, S., Stevens, B., and Zhang, X.: Clouds and Aerosols, in: Climate Change 2013: The Physical Science Basis, Contribution of Working Group I to the Fifth Assessment Report of the Intergovernmental Panel on Climate Change, edited by: Stocker, T. F., Qin, D., Plattner, G.-K., Tignor, M., Allen, S. K., Boschung, J., Nauels, A., Xia, Y., Bex, V., and Midgley, P. M., Cambridge University Press, Cambridge, United Kingdom and New York, NY, USA, 2013. a, b, c
Boysen, L. R., Brovkin, V., Arora, V. K., Cadule, P., de Noblet-Ducoudré, N., Kato, E., Pongratz, J., and Gayler, V.: Global and regional effects of land-use change on climate in 21st century simulations with interactive carbon cycle, Earth Syst. Dynam., 5, 309–319, https://doi.org/10.5194/esd-5-309-2014, 2014. a
Brännvall, M.-L., Bindler, R., Renberg, I., Emteryd, O., Bartnicki, J., and Billström, K.: The Medieval Metal Industry Was the Cradle of Modern Large-Scale Atmospheric Lead Pollution in Northern Europe, Environ. Sci. Technol., 33, 4391–4395, https://doi.org/10.1021/es990279n, 1999. a
Brovkin, V., Raddatz, T., Reick, C. H., Claussen, M., and Gayler, V.: Global biogeophysical interactions between forest and climate, Geophys. Res. Lett., 36, L07405, https://doi.org/10.1029/2009GL037543, 2009. a
Carslaw, K. S., Gordon, H., Hamilton, D. S., Johnson, J. S., Regayre, L. A., Yoshioka, M., and Pringle, K. J.: Aerosols in the Pre-industrial Atmosphere, Current Climate Change Reports, 3, 1–15, https://doi.org/10.1007/s40641-017-0061-2, 2017. a
Christiansen, B. and Ljungqvist, F. C.: The extra-tropical Northern Hemisphere temperature in the last two millennia: reconstructions of low-frequency variability, Clim. Past, 8, 765–786, https://doi.org/10.5194/cp-8-765-2012, 2012. a
Claussen, M., Brovkin, V., and Ganopolski, A.: Biogeophysical versus biogeochemical feedbacks of large-scale land cover change, Geophys. Res. Lett., 28, 1011–1014, https://doi.org/10.1029/2000GL012471, 2001. a
Crutzen, P. J. and Brühl, C.: A model study of atmospheric temperatures and the concentrations of ozone, hydroxyl, and some other photochemically active gases during the glcial, the pre-industrial Holocene and the present, Geophys. Res. Lett., 20, 1047–1050, 1993. a, b
Cruz, M. G., Sullivan, A. S., Kidnie, S., Hurley, R., and Nichols, S.: The effect of grass curing and fuel structure on fire behaviour, Tech. rep., CSIRP Land and Water, Client Report No EP 166414, Canberra, Australia, 2016. a
Cruz, M. G., Sullivan, A. L., Hurley, R. J., Plucinski, M. P., and Gould, J. S.: The effect of fuel load and structure on grassland fire behaviour and fire danger, Tech. rep., CSIRO Land and Water, Client Report No EF178976, Canberra, Australia, 2017. a
Dimitrakopoulos, A. P.: Mediterranean fuel models and potential fire behaviour in Greece, Int. J. Wildland Fire, 11, 127–130, https://doi.org/10.1071/WF02018, 2002. a
Dyer, R. M.: Fire and Vegetation Management in Pasture Lands of the Victoria River District, Northern Territory, Master's thesis, 2011. a
Farina, S. C., Adams, P. J., and Pandis, S. N.: Modeling global secondary organic aerosol formation and processing with the volatility basis set: Implications for anthropogenic secondary organic aerosol, J. Geophys. Res.-Atmos., 115, D09202, https://doi.org/10.1029/2009JD013046, 2010. a
Freestone, I. C.: The Recycling and Reuse of Roman Glass: Analytical Approaches, Journal of Glass Studies, 57, 29–40, 2015. a
Frost, P. G. H. and Robertson, F.: Chapter 5 – Fire. The ecological effects of fire in savannas, IUBS monograph series no. 3, 1987. a
Ge, Q., Hao, Z., Zheng, J., and Shao, X.: Temperature changes over the past 2000 yr in China and comparison with the Northern Hemisphere, Clim. Past, 9, 1153–1160, https://doi.org/10.5194/cp-9-1153-2013, 2013. a
Gilgen, A.: Data for study on land use and aerosol effects in the Roman Empire, available at: https://data.iac.ethz.ch/Gilgen_et_al_2019_RomanEmpire/, last access: 15 October 2019. a
Goodchild, H.: Modelling Roman agricultural production in the middle Tiber Valley, Central Italy, University of Birmingham Research Archive, e-theses repository, 2007. a, b
Gordon, H., Sengupta, K., Rap, A., Duplissy, J., Frege, C., Williamson, C., Heinritzi, M., Simon, M., Yan, C., Almeida, J., Tröstl, J., Nieminen, T., Ortega, I. K., Wagner, R., Dunne, E. M., Adamov, A., Amorim, A., Bernhammer, A.-K., Bianchi, F., Breitenlechner, M., Brilke, S., Chen, X., Craven, J. S., Dias, A., Ehrhart, S., Fischer, L., Flagan, R. C., Franchin, A., Fuchs, C., Guida, R., Hakala, J., Hoyle, C. R., Jokinen, T., Junninen, H., Kangasluoma, J., Kim, J., Kirkby, J., Krapf, M., Kürten, A., Laaksonen, A., Lehtipalo, K., Makhmutov, V., Mathot, S., Molteni, U., Monks, S. A., Onnela, A., Peräkylä, O., Piel, F., Petäjä, T., Praplan, A. P., Pringle, K. J., Richards, N. A. D., Rissanen, M. P., Rondo, L., Sarnela, N., Schobesberger, S., Scott, C. E., Seinfeld, J. H., Sharma, S., Sipilä, M., Steiner, G., Stozhkov, Y., Stratmann, F., Tomé, A., Virtanen, A., Vogel, A. L., Wagner, A. C., Wagner, P. E., Weingartner, E., Wimmer, D., Winkler, P. M., Ye, P., Zhang, X., Hansel, A., Dommen, J., Donahue, N. M., Worsnop, D. R., Baltensperger, U., Kulmala, M., Curtius, J., and Carslaw, K. S.: Reduced anthropogenic aerosol radiative forcing caused by biogenic new particle formation, P. Natl. Acad. Sci. USA, 113, 12053–12058, https://doi.org/10.1073/pnas.1602360113, 2016. a, b
Haider, M. Z.: Economics of Rice Residue Burning in the South-West Region of Bangladesh, Tech. rep., Economic Research Group, Dhaka, Bangladesh, 2011. a
Halmer, M. M., Schmicke, H.-U., and Graf, H.-F.: The annual volcanic gas input into the atmosphere, in particular into the stratosphere: a global data set for the past 100 years, J. Volcanol. Geoth. Res., 115, 511–528, 2002. a
Harris, W.: Defining and Detecting Mediterranean Deforestation, 800BCE to 700CE, 173–194, Brill, Leiden, the Netherlands, available at: https://brill.com/view/book/edcoll/9789004254053/B9789004254053_008.xml (last access: 15 October 2015), booktitle: The Ancient Mediterranean Environment between Science and History, Brill, Leiden, The Netherlands, ISBN 9789004254053, 2013. a
Hay, R. K. M.: Harvest index: a review of its use in plant breeding and crop physiology, Ann. Appl. Biol., 126, 197–216, 1995. a, b
Henrot, A.-J., Stanelle, T., Schröder, S., Siegenthaler, C., Taraborrelli, D., and Schultz, M. G.: Implementation of the MEGAN (v2.1) biogenic emission model in the ECHAM6-HAMMOZ chemistry climate model, Geosci. Model Dev., 10, 903–926, https://doi.org/10.5194/gmd-10-903-2017, 2017. a
Hin, S.: The demography of Roman Italy, Cambridge University Press, 2013. a, b
Holder, A., Gullett, B., Urbanski, S., Elleman, R., O'Neill, S., Tabor, D., Mitchell, W., and Baker, K.: Emissions from prescribed burning of agricultural fields in the Pacific Northwest, Atmos. Environ., 166, 22–33, https://doi.org/10.1016/j.atmosenv.2017.06.043, 2017. a
Hong, S., Candelone, J.-P., Patterson, C. C., and Boutron, C. F.: History of Ancient Copper Smelting Pollution During Roman and Medieval Times Recorded in Greenland Ice, Science, 272, 246–249, https://doi.org/10.1126/science.272.5259.246, 1996. a
Hoose, C., Kristjánsson, J. E., Iversen, T., Kirkevåg, A., Seland, O., and Gettelman, A.: Constraining cloud droplet number concentration in GCMs suppresses the aerosol indirect effect, Geophys. Res. Lett., 36, L12807, https://doi.org/10.1029/2009gl038568, 2009. a, b
Hopkins, K.: Taxes and Trade in the Roman Empire (200 B.C.–A.D. 400), J. Roman Stud., 70, 101–125, https://doi.org/10.2307/299558, 1980. a
Hopkins, K.: Models, Ships and Staples, Cambridge Classical Studies, Cambridge University Press, 213–268, https://doi.org/10.1017/CBO9781139093552.009, 2017. a
Jacobson, M. Z.: Fundamentals of Atmospheric Modeling, Cambridge University Press, https://doi.org/10.1017/CBO9781139165389, 2005. a
Janssen, E., Poblome, J., Claeys, J., Kint, V., Degryse, P., Marinova, E., and Muys, B.: Fuel for debating ancient economies. Calculating wood consumption at urban scale in Roman Imperial times, J. Archaeol. Sci., 11, 592–599, https://doi.org/10.1016/j.jasrep.2016.12.029, 2017. a, b, c
Kaplan, J. O., Krumhardt, K. M., and Zimmermann, N.: The prehistoric and preindustrial deforestation of Europe, Quaternary Sci. Rev., 28, 3016–3034, https://doi.org/10.1016/j.quascirev.2009.09.028, 2009. a
Kaplan, J. O., Krumhardt, K. M., Ellis, E. C., Ruddiman, W. F., Lemmen, C., and Goldewijk, K. K.: Holocene carbon emissions as a result of anthropogenic land cover change, Holocene, 21, 775–791, https://doi.org/10.1177/0959683610386983, 2011. a, b, c, d
Kaplan, J. O., Krumhardt, K. M., and Zimmermann, N. E.: The effects of land use and climate change on the carbon cycle of Europe over the past 500 years, Glob. Change Biol., 18, 902–914, https://doi.org/10.1111/j.1365-2486.2011.02580.x, 2012. a, b, c
Kaplan, J. O., Pfeiffer, M., Kolen, J. C. A., and Davis, B. A. S.: Large Scale Anthropogenic Reduction of Forest Cover in Last Glacial Maximum Europe, PLoS One, 11, e0166726, https://doi.org/10.1371/journal.pone.0166726, 2016. a, b
Kessler, D. and Temin, P.: The organization of the grain trade in the early Roman Empire, Econ. Hist. Rev., 60, 313–332, https://doi.org/10.1111/j.1468-0289.2006.00360.x, 2007. a
Khan, M., Cooke, M., Utembe, S., Archibald, A., Derwent, R., Xiao, P., Percival, C., Jenkin, M., Morris, W., and Shallcross, D.: Global modeling of the nitrate radical (NO3) for present and pre-industrial scenarios, Atmos. Res., 164, 347–357, https://doi.org/10.1016/j.atmosres.2015.06.006, 2015. a
Klein Goldewijk, K., Beusen, A., and Janssen, P.: Long-term dynamic modeling of global population and built-up area in a spatially explicit way: HYDE 3.1, Holocene, 20, 565–573, https://doi.org/10.1177/0959683609356587, 2010. a, b, c, d, e
Klein Goldewijk, K., Beusen, A., van Drecht, G., and de Vos, M.: The HYDE 3.1 spatially explicit database of human-induced global land-use change over the past 12 000 years, Global Ecol. Biogeogr., 20, 73–86, https://doi.org/10.1111/j.1466-8238.2010.00587.x, 2011. a, b, c, d, e, f, g
Klein Goldewijk, K., Beusen, A., Doelman, J., and Stehfest, E.: Anthropogenic land use estimates for the Holocene – HYDE 3.2, Earth Syst. Sci. Data, 9, 927–953, https://doi.org/10.5194/essd-9-927-2017, 2017. a, b, c
Koch, D. and Del Genio, A. D.: Black carbon semi-direct effects on cloud cover: review and synthesis, Atmos. Chem. Phys., 10, 7685–7696, https://doi.org/10.5194/acp-10-7685-2010, 2010. a, b
Kokkola, H., Korhonen, H., Lehtinen, K. E. J., Makkonen, R., Asmi, A., Järvenoja, S., Anttila, T., Partanen, A.-I., Kulmala, M., Järvinen, H., Laaksonen, A., and Kerminen, V.-M.: SALSA – a Sectional Aerosol module for Large Scale Applications, Atmos. Chem. Phys., 8, 2469–2483, https://doi.org/10.5194/acp-8-2469-2008, 2008. a
Kokkola, H., Kühn, T., Laakso, A., Bergman, T., Lehtinen, K. E. J., Mielonen, T., Arola, A., Stadtler, S., Korhonen, H., Ferrachat, S., Lohmann, U., Neubauer, D., Tegen, I., Siegenthaler-Le Drian, C., Schultz, M. G., Bey, I., Stier, P., Daskalakis, N., Heald, C. L., and Romakkaniemi, S.: SALSA2.0: The sectional aerosol module of the aerosol–chemistry–climate model ECHAM6.3.0-HAM2.3-MOZ1.0, Geosci. Model Dev., 11, 3833–3863, https://doi.org/10.5194/gmd-11-3833-2018, 2018. a
Kühn, T., Merikanto, J., Mielonen, T., Stadtler, S., Schultz, M., Hienola, A., Korhonen, H., Ferrachat, S., Lohmann, U., Neubauer, D., Tegen, I., Drian, C. S.-L., Rast, S., Schmidt, H., Stier, P., Lehtinen, K., and Kokkola, H.: SALSA2.0 – part 2: Implementation of a volatility basis set to model formation of secondary organic aerosol, Geosci. Model Dev., in preparation, 2019. a
Lamarque, J.-F., Bond, T. C., Eyring, V., Granier, C., Heil, A., Klimont, Z., Lee, D., Liousse, C., Mieville, A., Owen, B., Schultz, M. G., Shindell, D., Smith, S. J., Stehfest, E., Van Aardenne, J., Cooper, O. R., Kainuma, M., Mahowald, N., McConnell, J. R., Naik, V., Riahi, K., and van Vuuren, D. P.: Historical (1850–2000) gridded anthropogenic and biomass burning emissions of reactive gases and aerosols: methodology and application, Atmos. Chem. Phys., 10, 7017–7039, https://doi.org/10.5194/acp-10-7017-2010, 2010. a
Lasslop, G., Thonicke, K., and Kloster, S.: SPITFIRE within the MPI Earth system model: Model development and evaluation, J. Adv. Model. Earth Syst., 6, 740–755, https://doi.org/10.1002/2013MS000284, 2014. a, b
Lee, X., Goulden, M. L., Hollinger, D. Y., Barr, A., Black, T. A., Bohrer, G., Bracho, R., Drake, B., Goldstein, A., Gu, L., Katul, G., Kolb, T., Law, B. E., Margolis, H., Meyers, T., Monson, R., Munger, W., Oren, R., Paw U, K. T., Richardson, A. D., Schmid, H. P., Staebler, R., Wofsy, S., and Zhao, L.: Observed increase in local cooling effect of deforestation at higher latitudes, Nature, 479, 384–387, https://doi.org/10.1038/nature10588, 2011. a, b
Lejeune, Q., Seneviratne, S. I., and Davin, E. L.: Historical land-cover change impacts on climate: comparative assessment of LUCID and CMIP5 multimodel experiments, AMS, 30, 1439–1459, https://doi.org/10.1175/JCLI-D-16-0213.s1, 2017. a
Lelieveld, J., Peters, W., Dentener, F. J., and Krol, M. C.: Stability of tropospheric hydroxyl chemistry, J. Geophys. Res.-Atmos., 107, ACH 17-1–ACH 17-11, https://doi.org/10.1029/2002JD002272, 2002. a
Li, J., Bo, Y., and Xie, S.: Estimating emissions from crop residue open burning in China based on statistics and MODIS fire products, J. Environ. Sci., 44, 158–170, https://doi.org/10.1016/j.jes.2015.08.024, 2016a. a, b
Li, J., Li, Y., Bo, Y., and Xie, S.: High-resolution historical emission inventories of crop residue burning in fields in China for the period 1990–2013, Atmos. Environ., 138, 152–161, https://doi.org/10.1016/j.atmosenv.2016.05.002, 2016b. a, b
Li, Y., Zhao, M., Motesharrei, S., Mu, Q., Kalnay, E., and Li, S.: Local cooling and warming effects of forests based on satellite observations, Nat. Commun., 6, 6603, https://doi.org/10.1038/ncomms7603, 2015. a, b
Li, Y., Henze, D. K., Jack, D., and Kinney, P. L.: The influence of air quality model resolution on health impact assessment for fine particulate matter and its components, Air Qual. Atmos. Hlth., 9, 51–68, https://doi.org/10.1007/s11869-015-0321-z, 2016a. a
Li, Y., Zhao, M., Mildrexler, D. J., Motesharrei, S., Mu, Q., Kalnay, E., Zhao, F., Li, S., and Wang, K.: Potential and Actual impacts of deforestation and afforestation on land surface temperature, J. Geophy. Res.-Atmos., 121, 14372–14386, https://doi.org/10.1002/2016JD024969, 2016b. a, b, c
Little, I. T., Hockey, P. A., and Jansen, R.: Impacts of fire and grazing management on South Africa's moist highland grasslands: A case study of the Steenkampsberg Plateau, Mpumalanga, South Africa, Bothalia – African Biodiversity & Conservation, 45, 1–15, 2015. a
Magi, B. I.: Global Lightning Parameterization from CMIP5 Climate Model Output, J. Atmos. Ocean. Tech., 32, 434–452, https://doi.org/10.1175/JTECH-D-13-00261.1, 2015. a, b
Makra, L.: Anthropogenic Air Pollution in Ancient Times, History of Toxicology and Environmental Health, 2, 21–41, 2015. a, b, c, d
Malanima, P.: Energy crisis and growth 1650–1850: the European deviation in a comparative perspective, J. Global Hist., 1, 101–121, https://doi.org/10.1017/S1740022806000064, 2006. a
Malanima, P.: Energy Consumption in the Roman World, booktitle: The Ancient Mediterranean Environment between Science and History, 13–36, Brill, Leiden, the Netherlands, available at: https://brill.com/view/book/edcoll/9789004254053/B9789004254053_003.xml (last access: 15 October 2019), 2013. a, b, c, d, e, f, g
Meinshausen, M., Vogel, E., Nauels, A., Lorbacher, K., Meinshausen, N., Etheridge, D. M., Fraser, P. J., Montzka, S. A., Rayner, P. J., Trudinger, C. M., Krummel, P. B., Beyerle, U., Canadell, J. G., Daniel, J. S., Enting, I. G., Law, R. M., Lunder, C. R., O'Doherty, S., Prinn, R. G., Reimann, S., Rubino, M., Velders, G. J. M., Vollmer, M. K., Wang, R. H. J., and Weiss, R.: Historical greenhouse gas concentrations for climate modelling (CMIP6), Geosci. Model Dev., 10, 2057–2116, https://doi.org/10.5194/gmd-10-2057-2017, 2017. a
Mielonen, T., Hienola, A., Kühn, T., Merikanto, J., Lipponen, A., Bergman, T., Korhonen, H., Kolmonen, P., Sogacheva, L., Ghent, D., Pitkänen, M. R. A., Arola, A., De Leeuw, G., and Kokkola, H.: Summertime Aerosol Radiative Effects and Their Dependence on Temperature over the Southeastern USA, Atmosphere, 9, https://doi.org/10.3390/atmos9050180, 2018. a
Mietz, M.: The fuel economy of public bathhouses in the Roman Empire, Master's thesis, Ghent University, Faculty of Arts and Philosophy, Campus Boekentoren, Blandijnberg 2, 9000 Ghent, Belgium, 2016. a, b
Montiel, C. and Kraus, D.: Best Practices of Fire Use – Prescribed Burning and Suppression Fire Programmes in Selected Case-Study Regions of Europe, European Forest Institute, 2010. a, b, c
Morales-Molino, C., Vescovi, E., Krebs, P., Carlevaro, E., Kaltenrieder, P., Conedera, M., Tinner, W., and Colombaroli, D.: The role of human-induced fire and sweet chestnut (Castanea sativa Mill.) cultivation on the long-term landscape dynamics of the southern Swiss Alps, Holocene, 25, 482–494, https://doi.org/10.1177/0959683614561884, 2015. a
Murray, L. T., Mickley, L. J., Kaplan, J. O., Sofen, E. D., Pfeiffer, M., and Alexander, B.: Factors controlling variability in the oxidative capacity of the troposphere since the Last Glacial Maximum, Atmos. Chem. Phys., 14, 3589–3622, https://doi.org/10.5194/acp-14-3589-2014, 2014. a, b
Naveh, Z.: The evolutionary significance of fire in the mediterranean region, Vegetatio, 29, 199–208, 1975. a
Norman, M. J. T.: The short term effects of time and frequency of burning on native pastures at Katherine, N. T., Australian Journal of Experimental Agriculture and Animal Husbandry, 3, 26–29, 1963. a
Norman, M. J. T.: The effect of burning and seasonal rainfall on native pasture at Katherine, N. T., Australian Journal of Experimental Agriculture and Animal Husbandry, 9, 295–298, 1969. a
Oluwole, F. A., Sambo, J. M., and Sikhalazo, D.: Long-term effects of different burning frequencies on the dry savannah grassland in South Africa, Afr. J. Agric. Res., 3, 147–153, 2008. a
PAGES 2k Consortium: Continental-scale temperature variability during the past two millennia, Nat. Geosci., 6, 339–346, https://doi.org/10.1038/ngeo1797, 2013. a
Papanastasis, V. P.: Effects of Season and Frequency of Burning on a Phryganic Rangeland in Greece, J. Range Manage., 33, 251–255, 1980. a, b
Pfeiffer, M., Spessa, A., and Kaplan, J. O.: A model for global biomass burning in preindustrial time: LPJ-LMfire (v1.0), Geosci. Model Dev., 6, 643–685, https://doi.org/10.5194/gmd-6-643-2013, 2013. a, b, c
Pham, M., Müller, J.-F., Brasseur, G. P., Granier, C., and Mégie, G.: A three-dimensional study of the tropospheric sulfur cycle, J. Geophys. Res., 100, 26061–26092, 1995. a
Pinto, J. P. and Khalil, M. A. K.: The stability of tropospheric OH during ice ages, inter-glacial epochs and modern times, Tellus, 43B, 347–352, 1991. a, b
PRSC: Monitoring Residue Burning through Satellite Remote Sensing, Tech. rep., Punjab Remote Sensing Centre, Ludhiana – 141004, 2015. a, b
Rabin, S. S., Melton, J. R., Lasslop, G., Bachelet, D., Forrest, M., Hantson, S., Kaplan, J. O., Li, F., Mangeon, S., Ward, D. S., Yue, C., Arora, V. K., Hickler, T., Kloster, S., Knorr, W., Nieradzik, L., Spessa, A., Folberth, G. A., Sheehan, T., Voulgarakis, A., Kelley, D. I., Prentice, I. C., Sitch, S., Harrison, S., and Arneth, A.: The Fire Modeling Intercomparison Project (FireMIP), phase 1: experimental and analytical protocols with detailed model descriptions, Geosci. Model Dev., 10, 1175–1197, https://doi.org/10.5194/gmd-10-1175-2017, 2017. a, b
Raddatz, T. J., Reick, C. H., Knorr, W., Kattge, J., Roeckner, E., Schnur, R., Schnitzler, K.-G., Wetzel, P., and Jungclaus, J.: Will the tropical land biosphere dominate the climate–carbon cycle feedback during the twenty-first century?, Clim. Dynam., 29, 565–574, https://doi.org/10.1007/s00382-007-0247-8, 2007. a
Reick, C. H., Raddatz, T., Brovkin, V., and Gayler, V.: Representation of natural and anthropogenic land cover change in MPI-ESM, J. Adv. Model. Earth Syst., 5, 459–482, https://doi.org/10.1002/jame.20022, 2013. a, b
SANBI: Grazing and Burning Guidelines: Managing Grasslands for Biodiversity and Livestock Production, 2014. a, b
Sapart, C. J., Monteil, G., Prokopiou, M., van de Wal, R. S. W., Kaplan, J. O., Sperlich, P., Krumhardt, K. M., van der Veen, C., Houweling, S., Krol, M. C., Blunier, T., Sowers, T., Martinerie, P., Witrant, E., Dahl-Jensen, D., and Röckmann, T.: Natural and anthropogenic variations in methane sources during the past two millennia, Nature, 490, 85–88, https://doi.org/10.1038/nature11461, 2012. a
Scheidel, W.: Roman Population Size: The Logic of the Debate, Brill, 2008. a, b, c
Scheidel, W.: Population and Demography, chap. 13, 134–145, John Wiley & Sons, Ltd, https://doi.org/10.1002/9781444308372.ch13, 2009. a, b
Shea, R. W., Shea, B. W., Kauffman, J. B., Ward, D. E., Haskins, C. I., and Scholes, M. C.: Fuel biomass and combustion factors associated with fires in savanna ecosystems of South Africa and Zambia, J. Geophys. Res., 101, 23551–23568, 1996. a
Sigg, A. and Neftel, A.: Evidence for a 50% increase in H2O2 over the past 200 years from a Greenland ice core, Nature, 351, 557–559, 1991. a
Sinclair, T. R.: Historical Changes in Harvest Index and Crop Nitrogen Accumulation, Crop Sci., 38, 638–643, 1998. a, b
Smit, H., Metzger, M., and Ewert, F.: Spatial distribution of grassland productivity and land use in Europe, Agr. Syst., 98, 208–219, https://doi.org/10.1016/j.agsy.2008.07.004, 2008. a, b
Smith, A.: Provenance of Coals from Roman Sites in England and Wales, Britannia, 28, 297–324, https://doi.org/10.2307/526770, 1997. a
Smith, M. C., Singarayer, J. S., Valdes, P. J., Kaplan, J. O., and Branch, N. P.: The biogeophysical climatic impacts of anthropogenic land use change during the Holocene, Clim. Past, 12, 923–941, https://doi.org/10.5194/cp-12-923-2016, 2016. a, b
Smith, M. D., van Wilgen, B. W., Burns, C. E., Govender, N., Potgieter, A. L. F., Andelman, S., Biggs, H. C., Botha, J., and Trollope, W. S. W.: Long-term effects of fire frequency and season on herbaceous vegetation in savannas of the Kruger National Park, South Africa, J. Plant Ecol., 6, 71–83, https://doi.org/10.1093/jpe/rts014, 2013. a, b
Spurr, M. S.: Arable cultivation in Roman Italy, The Society for the Promotion of Roman Studies, 1986. a, b, c, d, e, f, g, h, i, j, k, l
Stadtler, S., Kühn, T., Schröder, S., Taraborrelli, D., Schultz, M. G., and Kokkola, H.: Isoprene-derived secondary organic aerosol in the global aerosol–chemistry–climate model ECHAM6.3.0–HAM2.3–MOZ1.0, Geosci. Model Dev., 11, 3235–3260, https://doi.org/10.5194/gmd-11-3235-2018, 2018. a
Stern, E. M.: Roman Glassblowing in a Cultural Context, American Journal of Archaeology, 103, 441–484, 1999. a
Stier, P., Feichter, J., Kinne, S., Kloster, S., Vignati, E., Wilson, J., Ganzeveld, L., Tegen, I., Werner, M., Balkanski, Y., Schulz, M., Boucher, O., Minikin, A., and Petzold, A.: The aerosol-climate model ECHAM5-HAM, Atmos. Chem. Phys., 5, 1125–1156, https://doi.org/10.5194/acp-5-1125-2005, 2005. a
Tegen, I., Neubauer, D., Ferrachat, S., Siegenthaler-Le Drian, C., Bey, I., Schutgens, N., Stier, P., Watson-Parris, D., Stanelle, T., Schmidt, H., Rast, S., Kokkola, H., Schultz, M., Schroeder, S., Daskalakis, N., Barthel, S., Heinold, B., and Lohmann, U.: The global aerosol–climate model ECHAM6.3–HAM2.3 – Part 1: Aerosol evaluation, Geosci. Model Dev., 12, 1643–1677, https://doi.org/10.5194/gmd-12-1643-2019, 2019. a, b
Temin, P.: The Economy of the Early Roman Empire, J. Econ. Perspect., 20, 133–151, 2006. a
Thonicke, K., Spessa, A., Prentice, I. C., Harrison, S. P., Dong, L., and Carmona-Moreno, C.: The influence of vegetation, fire spread and fire behaviour on biomass burning and trace gas emissions: results from a process-based model, Biogeosciences, 7, 1991–2011, https://doi.org/10.5194/bg-7-1991-2010, 2010. a
Tinner, W., Conedera, M., Ammann, B., and Lotter, A. F.: Fire ecology north and south of the Alps since the last ice age, Holocene, 15, 1214–1226, https://doi.org/10.1191/0959683605hl892rp, 2005. a
Tinner, W., van Leeuwen, J. F., Colombaroli, D., Vescovi, E., van der Knaap, W., Henne, P. D., Pasta, S., D'Angelo, S., and Mantia, T. L.: Holocene environmental and climatic changes at Gorgo Basso, a coastal lake in southern Sicily, Italy, Quaternary Sci. Rev., 28, 1498–1510, https://doi.org/10.1016/j.quascirev.2009.02.001, 2009. a
Turn, S. Q., Jenkins, B. M., Chow, J. C., Pritchett, L. C., Campbell, D., Cahill, T., and Whalen, S. A.: Elemental characterizationof particulate matter emitted from biomass burning: Wind tunnel derived source profiles for herbaceous and wood fuels, J. Geophys. Res., 102, 3683–3699, 1997. a
Twomey, S.: Pollution and planetary albedo, Atmos. Environ., 8, 1251–1256, https://doi.org/10.1016/0004-6981(74)90004-3, 1974. a
Twomey, S.: The Influence of Pollution on the Shortwave Albedo of Clouds, Am. Meteorol. Soc., 34, 1149–1152, https://doi.org/10.1175/1520-0469(1977)034<1149:TIOPOT>2.0.CO;2, 1977. a
van Marle, M. J. E., Kloster, S., Magi, B. I., Marlon, J. R., Daniau, A.-L., Field, R. D., Arneth, A., Forrest, M., Hantson, S., Kehrwald, N. M., Knorr, W., Lasslop, G., Li, F., Mangeon, S., Yue, C., Kaiser, J. W., and van der Werf, G. R.: Historic global biomass burning emissions for CMIP6 (BB4CMIP) based on merging satellite observations with proxies and fire models (1750–2015), Geosci. Model Dev., 10, 3329–3357, https://doi.org/10.5194/gmd-10-3329-2017, 2017. a, b
Veal, R.: Wood and Charcoal for Rome: Towards an Understanding of Ancient Regional Fuel Economics, 388–406, Brill, Leiden, the Netherlands, available at: https://brill.com/view/book/edcoll/9789004345027/B9789004345027_017.xml (last access: 15 October 2019), 2017. a, b, c, d
Veira, A., Kloster, S., Schutgens, N. A. J., and Kaiser, J. W.: Fire emission heights in the climate system – Part 2: Impact on transport, black carbon concentrations and radiation, Atmos. Chem. Phys., 15, 7173–7193, https://doi.org/10.5194/acp-15-7173-2015, 2015. a, b
Warde, P.: Fear of Wood Shortage and the Reality of the Woodland in Europe, c. 1450–1850, Hist. Workshop J., 62, 28–57, https://doi.org/10.1093/hwj/dbl009, 2006. a
Webb, J., Hutchings, N., Amon, B., Nielsen, O.-K., Phillips, R., and Dämmgen, U.: Field burning of agricultural residues, Tech. rep., EMEP/EEA emission inventory guidebook 2013, booktitle: The Economic Integration of Roman Italy, available at: https://www.eea.europa.eu/ds_resolveuid/MWU9XE0TKP (last access: 15 October 2019), 2013. a, b
Weiberg, E., Hughes, R. E., Finné, M., Bonnier, A., and Kaplan, J. O.: Mediterranean land use systems from prehistory to antiquity: a case study from Peloponnese (Greece), Journal of Land Use Science, 14, 1–20, https://doi.org/10.1080/1747423X.2019.1639836, 2019. a
Weir, J. R., Fuhlendorf, S. D., Engle, D. M., Bidwell, T. G., Cummings, D. C., Elmore, D., Limb, R. F., Allred, B. W., Scasta, J. D., and Winter, S. L.: Patch Burning: Integrating Fire and Grazing to Promote Heterogeneity, available at: https://www.cattlemen.bc.ca/docs/paper_integrating_fire_and_grazing.pdf (last access: 15 October 2019), 2013. a, b
White, K. D.: Fallowing, Crop Rotation, and Crop Yields in Roman Times, Agr. Hist., 44, 281–290, 1970. a
Wilkenskjeld, S., Kloster, S., Pongratz, J., Raddatz, T., and Reick, C. H.: Comparing the influence of net and gross anthropogenic land-use and land-cover changes on the carbon cycle in the MPI-ESM, Biogeosciences, 11, 4817–4828, https://doi.org/10.5194/bg-11-4817-2014, 2014. a
Wilks, D. S.: The Stippling Shows Statistically Significant Grid Points: How Research Results are Routinely Overstated and Overinterpreted, and What to Do about It, B. Am. Meteorol. Soc., 97, 2263–2273, https://doi.org/10.1175/BAMS-D-15-00267.1, 2016. a
Winckler, J., Reick, C. H., Luyssaert, S., Cescatti, A., Stoy, P. C., Lejeune, Q., Raddatz, T., Chlond, A., Heidkamp, M., and Pongratz, J.: Different response of surface temperature and air temperature to deforestation in climate models, Earth Syst. Dynam., 10, 473–484, https://doi.org/10.5194/esd-10-473-2019, 2019. a
Witcher, R.: Agricultural Production in Roman Italy, 23, 459–482, Wiley-Blackwell, https://doi.org/10.1002/9781118993125.ch23, 2016. a
Wood, T. S. and Baldwin, S.: Fuelwood and charcoal use in developing countries, Ann. Rev. Energy, 10, 407–429, 1985. a
World Health Organisation: WHO Air quality guidelines for particulate matter, ozone, nitrogen dioxide and sulfur dioxid, Global update 2005, Summary of risk assessment, 2006. a
Yang, S., He, H., Lu, S., Chen, D., and Zhu, J.: Quantification of crop residue burning in the field and its influence on ambient air quality in Suqian, China, Atmos. Environ., 42, 1961–1969, https://doi.org/10.1016/j.atmosenv.2007.12.007, 2008. a, b
Yevich, R. and Logan, J. A.: An assessment of biofuel use and burning of agricultural waste in the developing world, Global Biogeochem. Cy., 17, 1095, https://doi.org/10.1029/2002GB001952, 2003. a, b, c, d, e, f, g
Zhang, L., Liu, Y., and Hao, L.: contributions of open crop straw burning emissions to PM2.5 concentrations in China, Environ. Res. Lett., 11, 014014, 2016. a
https://svn-ccsm-inputdata.cgd.ucar.edu/trunk/inputdata/atm/cam/tracer_cnst/tracer_cnst_WACCM6_halons_3DmonthlyL70_1850climo295_c180426.nc, last access: 27 July 2018.
http://www.claas.de/faszination-claas/themen/erntekalender-weltweit, last access: 23 August 2018.